Deep reinforcement transfer learning for active flow control of a 3D square cylinder under state dimension mismatch
arxiv(2024)
摘要
This paper focuses on developing a deep reinforcement learning (DRL) control strategy to mitigate aerodynamic forces acting on a three dimensional (3D) square cylinder under high Reynolds number flow conditions. Four jets situated at the corners of the square cylinder are used as actuators and pressure probes on the cylinder surface are employed as feedback observers. The Soft Actor-Critic (SAC) algorithm is deployed to identify an effective control scheme. Additionally, we pre-train the DRL agent using a two dimensional (2D) square cylinder flow field at a low Reynolds number (Re =1000), followed by transferring it to the 3D square cylinder at Re =22000. To address the issue of state dimension mismatch in transfer learning from 2D to 3D case, a state dimension mismatch transfer learning method is developed to enhance the SAC algorithm, named SDTL-SAC. The results demonstrate transfer learning across different state spaces achieves the same control policy as the SAC algorithm, resulting in a significant improvement in training speed with a training cost reduction of 51.1%. Furthermore, the SAC control strategy leads to a notable 52.3% reduction in drag coefficient, accompanied by substantial suppression of lift fluctuations. These outcomes underscore the potential of DRL in active flow control, laying the groundwork for efficient, robust, and practical implementation of this control technique in practical engineering.
更多查看译文
AI 理解论文
溯源树
样例
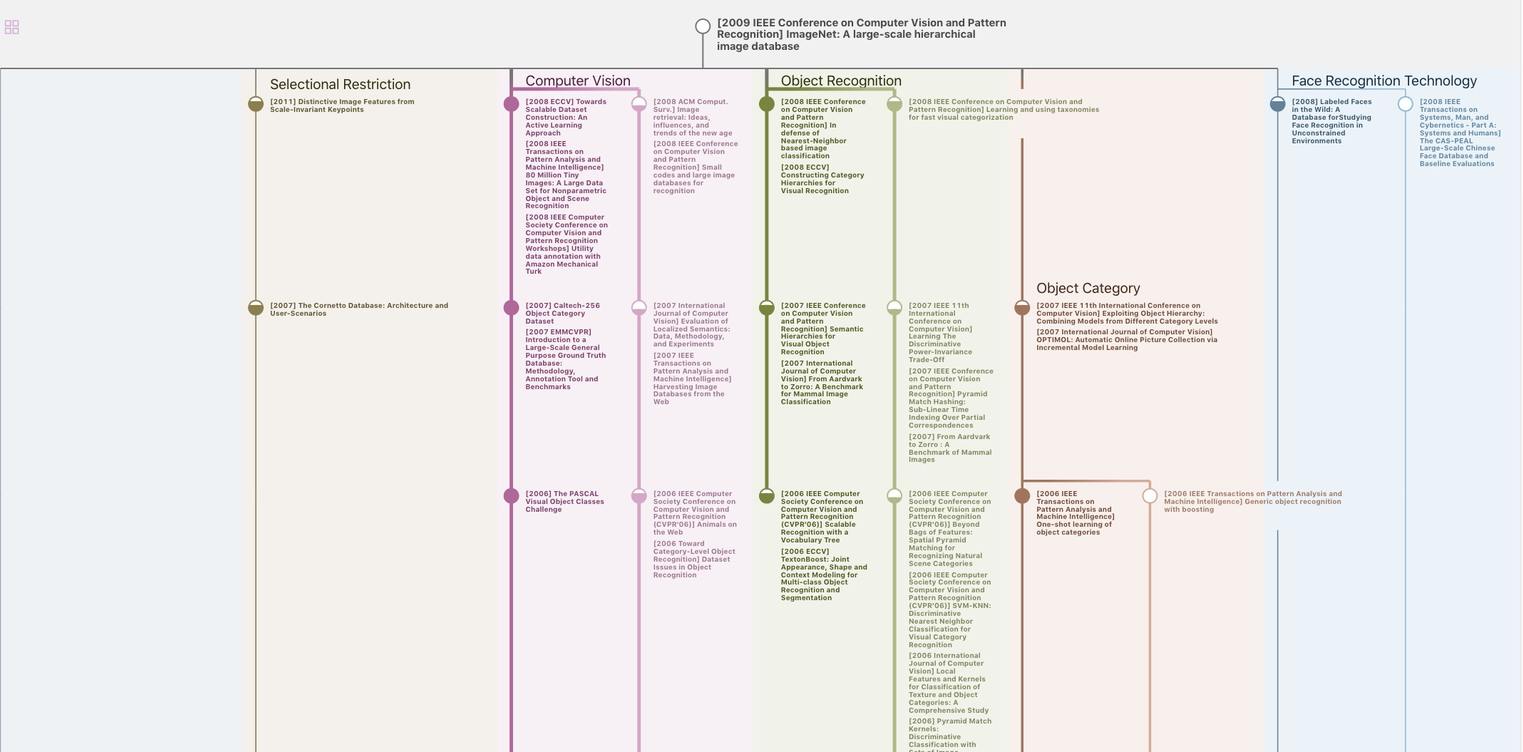
生成溯源树,研究论文发展脉络
Chat Paper
正在生成论文摘要