A fine digital soil mapping by integrating remote sensing-based process model and deep learning method in Northeast China
SOIL & TILLAGE RESEARCH(2024)
摘要
Accurate soil type maps provide an important basis for agricultural decision-making and land degradation control. In soil classification studies, the various environmental covariates are often selected based on the soilforming framework. Since the mapping area and available observation data are limited, meteorological and vegetation cover factors have not been fully developed, and their role in soil classification needs to be evaluated. In addition, whether deep learning has out -performance in soil classification remains to be tested. The aim of this paper is to evaluate the accuracy of deep learning modelling techniques in classifying soil type using different combinations of input variables, and evaluate the importance of soil-forming variables in soil type classification. Therefore, we collected commonly used environmental covariates in Northeast China (NEC), including multiple meteorological factors and adopted a satellite-based biophysical model (Boreal Ecosystem Productivity Simulator, BEPS) to enrich vegetation cover factors. Next, four modeling strategies were developed: the soil-forming factors of soil and relief were considered as traditional environmental covariates (T), as well as combined with meteorologic variables (T + C), vegetation cover variables (T + V) and all available environmental covariates (T + C + V). Then, the effectiveness of different modeling strategies for soil classification was explored with convolutional neural network (CNN) model and multi-layer random forest (MRF) model based on soil separability. Finally, a 30 m resolution soil type map was established. The results demonstrated that both MRF and CNN can achieve high accuracy soil classification, while the CNN model performs better. The descending order of classification accuracy based on different modeling strategies of the CNN model is shown as T + C + V: 91.08%, T + V: 88.84%, T + C: 86.82%, and T: 83.96%. Meanwhile, the separability of different soil-forming factors for soil classification is soil properties, vegetation cover, temporal variation, meteorologic and relief in descending order. For Castanozems and Brown soils, MRF has higher classification accuracy, while CNN has better performance in Meadow soils and Fluvo-aquic soils. The methodology proposed in this paper aims to achieve high accuracy soil classification, provide an approach to understand the importance of soil-forming factors for the region, as well as for different soil types, and provide references for facilitating the interpretation of misclassified areas. Our results are accurate in the core areas, and therefore, this work facilitates researchers to be able to focus more on areas where different soil types intersect, thus significantly improves efficiency and saves resources, and promises to be a useful tool for future soil surveys.
更多查看译文
关键词
Digital soil type mapping,Soil-forming,Boreal ecosystem productivity simulator,(BEPS) model,Convolutional neural network (CNN),Multi-layer random forest (MRF),Net primary productivity
AI 理解论文
溯源树
样例
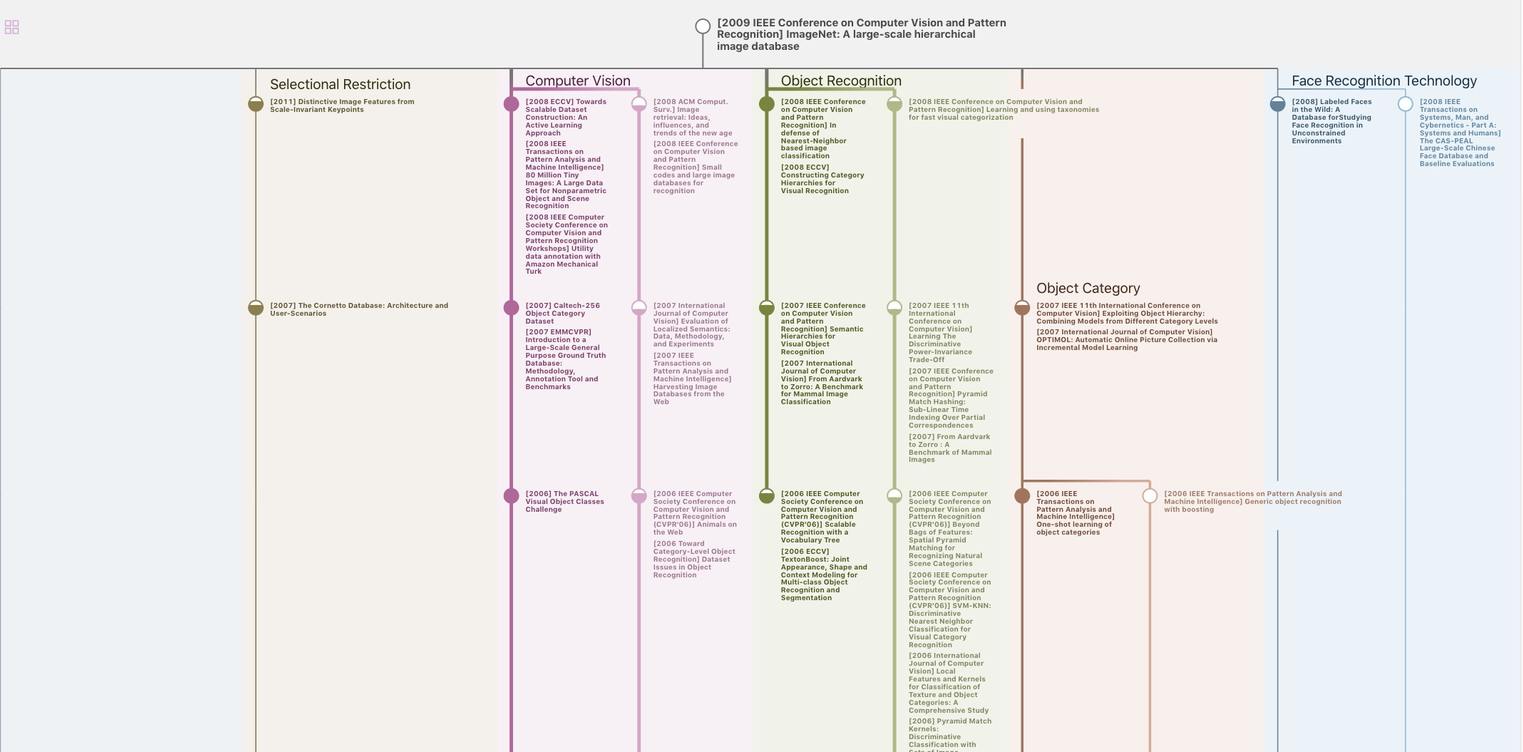
生成溯源树,研究论文发展脉络
Chat Paper
正在生成论文摘要