Lightweight Transmission Line Fault Detection Method Based on Leaner YOLOv7-Tiny
SENSORS(2024)
摘要
Aiming to address the issues of parameter complexity and high computational load in existing fault detection algorithms for transmission lines, which hinder their deployment on devices like drones, this study proposes a novel lightweight model called Leaner YOLOv7-Tiny. The primary goal is to swiftly and accurately detect typical faults in transmission lines from aerial images. This algorithm inherits the ELAN structure from YOLOv7-Tiny network and replaces its backbone with depthwise separable convolutions to reduce model parameters. By integrating the SP attention mechanism, it fuses multi-scale information, capturing features across various scales to enhance small target recognition. Finally, an improved FCIoU Loss function is introduced to balance the contribution of high-quality and low-quality samples to the loss function, expediting model convergence and boosting detection accuracy. Experimental results demonstrate a 20% reduction in model size compared to the original YOLOv7-Tiny algorithm. Detection accuracy for small targets surpasses that of current mainstream lightweight object detection algorithms. This approach holds practical significance for transmission line fault detection.
更多查看译文
关键词
fault detection,Leaner YOLOv7-Tiny,lightweight,transmission lines,YOLOv7-Tiny
AI 理解论文
溯源树
样例
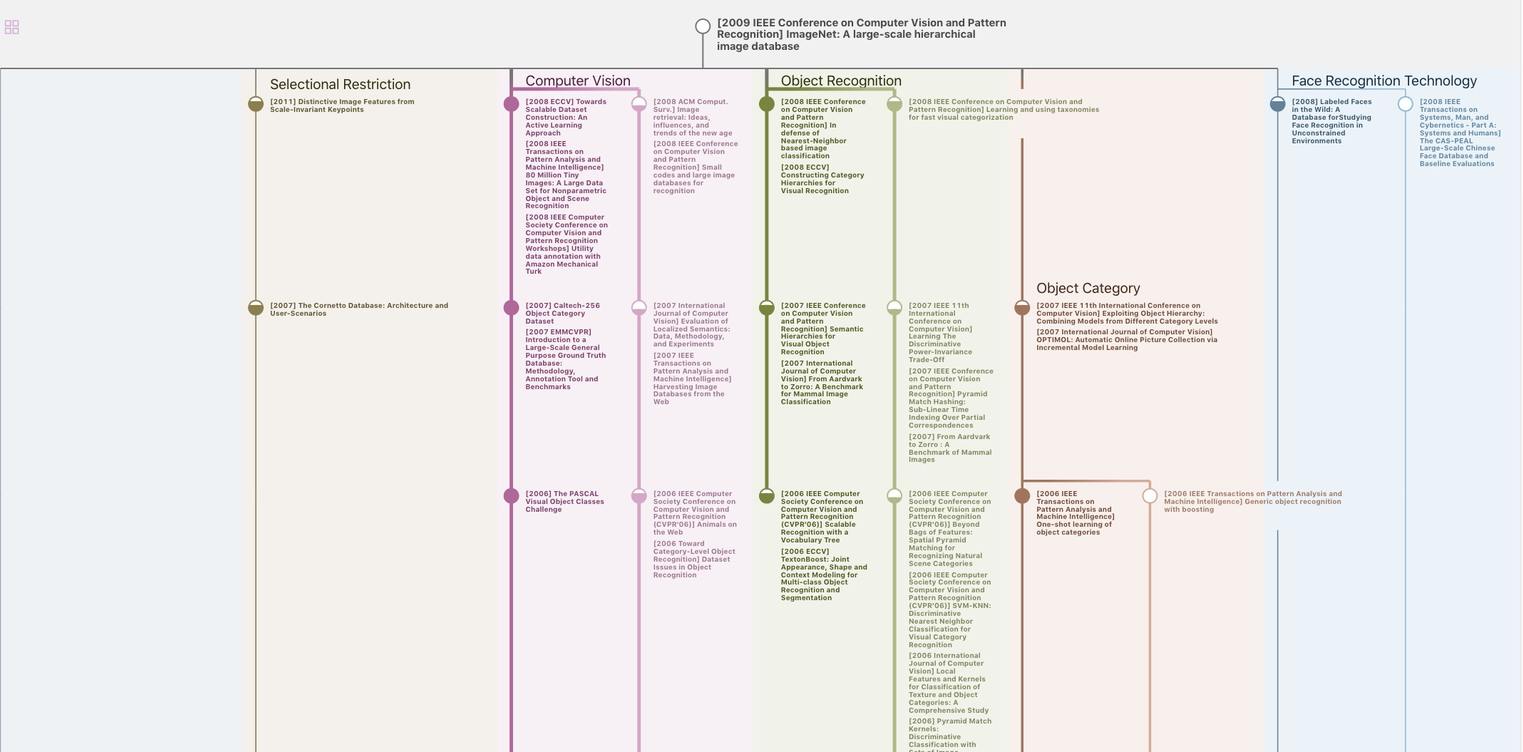
生成溯源树,研究论文发展脉络
Chat Paper
正在生成论文摘要