Efficient Defect Detection of Rotating Goods under the Background of Intelligent Retail
SENSORS(2024)
摘要
Dynamic visual vending machines are rapidly growing in popularity, offering convenience and speed to customers. However, there is a prevalent issue with consumers damaging goods and then returning them to the machine, severely affecting business interests. This paper addresses the issue from the standpoint of defect detection. Although existing industrial defect detection algorithms, such as PatchCore, perform well, they face challenges, including handling goods in various orientations, detection speeds that do not meet real-time monitoring requirements, and complex backgrounds that hinder detection accuracy. These challenges hinder their application in dynamic vending environments. It is crucial to note that efficient visual features play a vital role in memory banks, yet current memory repositories for industrial inspection algorithms do not adequately address the problem of location-specific feature redundancy. To tackle these issues, this paper introduces a novel defect detection algorithm for goods using adaptive subsampling and partitioned memory banks. Firstly, Grad-CAM is utilized to extract deep features, which, in combination with shallow features, mitigate the impact of complex backgrounds on detection accuracy. Next, graph convolutional networks extract rotationally invariant features. The adaptive subsampling partitioned memory bank is then employed to store features of non-defective goods, which reduces memory consumption and enhances training speed. Experimental results on the MVTec AD dataset demonstrate that the proposed algorithm achieves a marked improvement in detection speed while maintaining accuracy that is comparable to state-of-the-art models.
更多查看译文
关键词
anomaly detection,few-shot learning,memory repository,dynamic visual vending
AI 理解论文
溯源树
样例
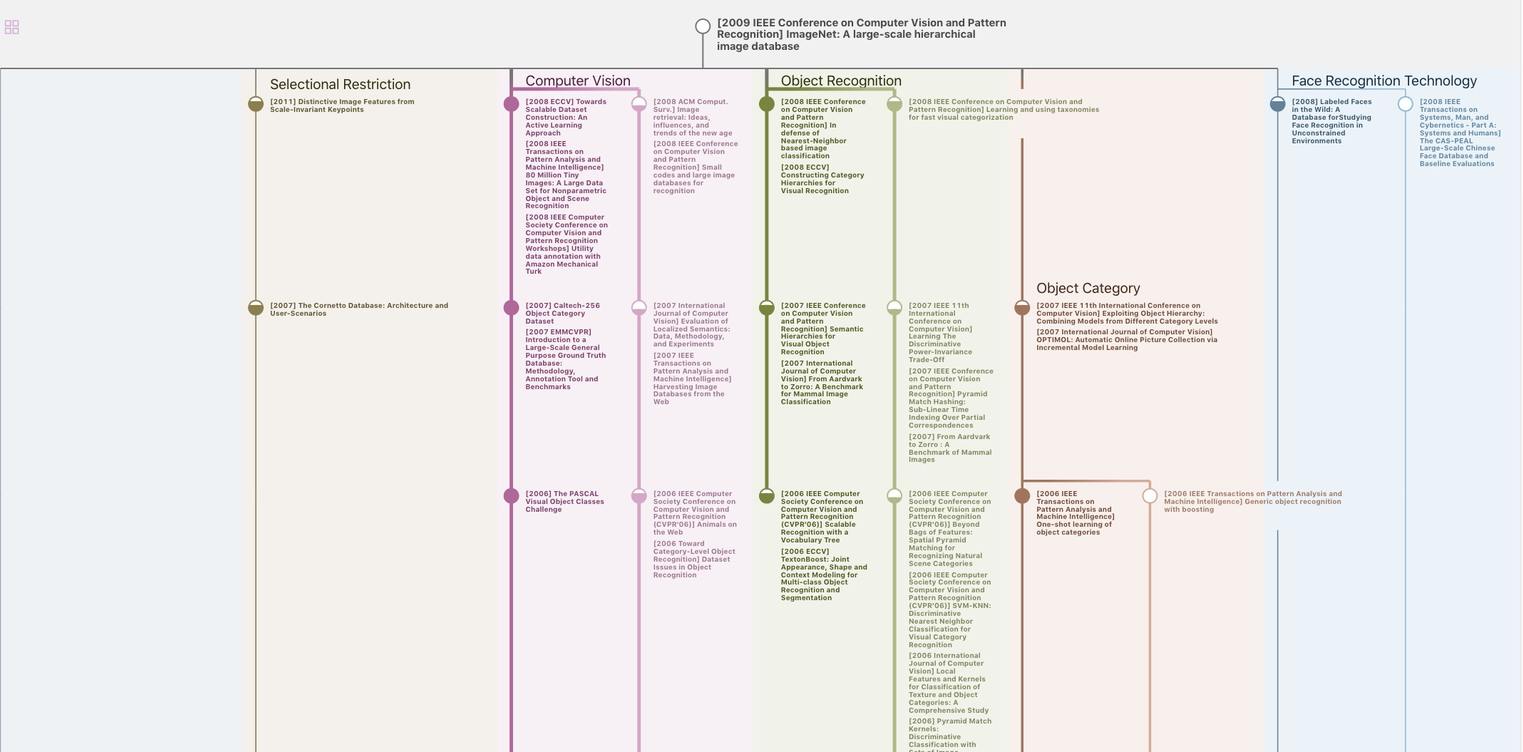
生成溯源树,研究论文发展脉络
Chat Paper
正在生成论文摘要