Human Pose Estimation Based on Efficient and Lightweight High-Resolution Network (EL-HRNet)
SENSORS(2024)
摘要
As an important direction in computer vision, human pose estimation has received extensive attention in recent years. A High-Resolution Network (HRNet) can achieve effective estimation results as a classical human pose estimation method. However, the complex structure of the model is not conducive to deployment under limited computer resources. Therefore, an improved Efficient and Lightweight HRNet (EL-HRNet) model is proposed. In detail, point-wise and grouped convolutions were used to construct a lightweight residual module, replacing the original 3 x 3 module to reduce the parameters. To compensate for the information loss caused by the network's lightweight nature, the Convolutional Block Attention Module (CBAM) is introduced after the new lightweight residual module to construct the Lightweight Attention Basicblock (LA-Basicblock) module to achieve high-precision human pose estimation. To verify the effectiveness of the proposed EL-HRNet, experiments were carried out using the COCO2017 and MPII datasets. The experimental results show that the EL-HRNet model requires only 5 million parameters and 2.0 GFlops calculations and achieves an AP score of 67.1% on the COCO2017 validation set. In addition, PCKh@0.5mean is 87.7% on the MPII validation set, and EL-HRNet shows a good balance between model complexity and human pose estimation accuracy.
更多查看译文
关键词
human pose estimation,lightweight network,HRNet,CBAM
AI 理解论文
溯源树
样例
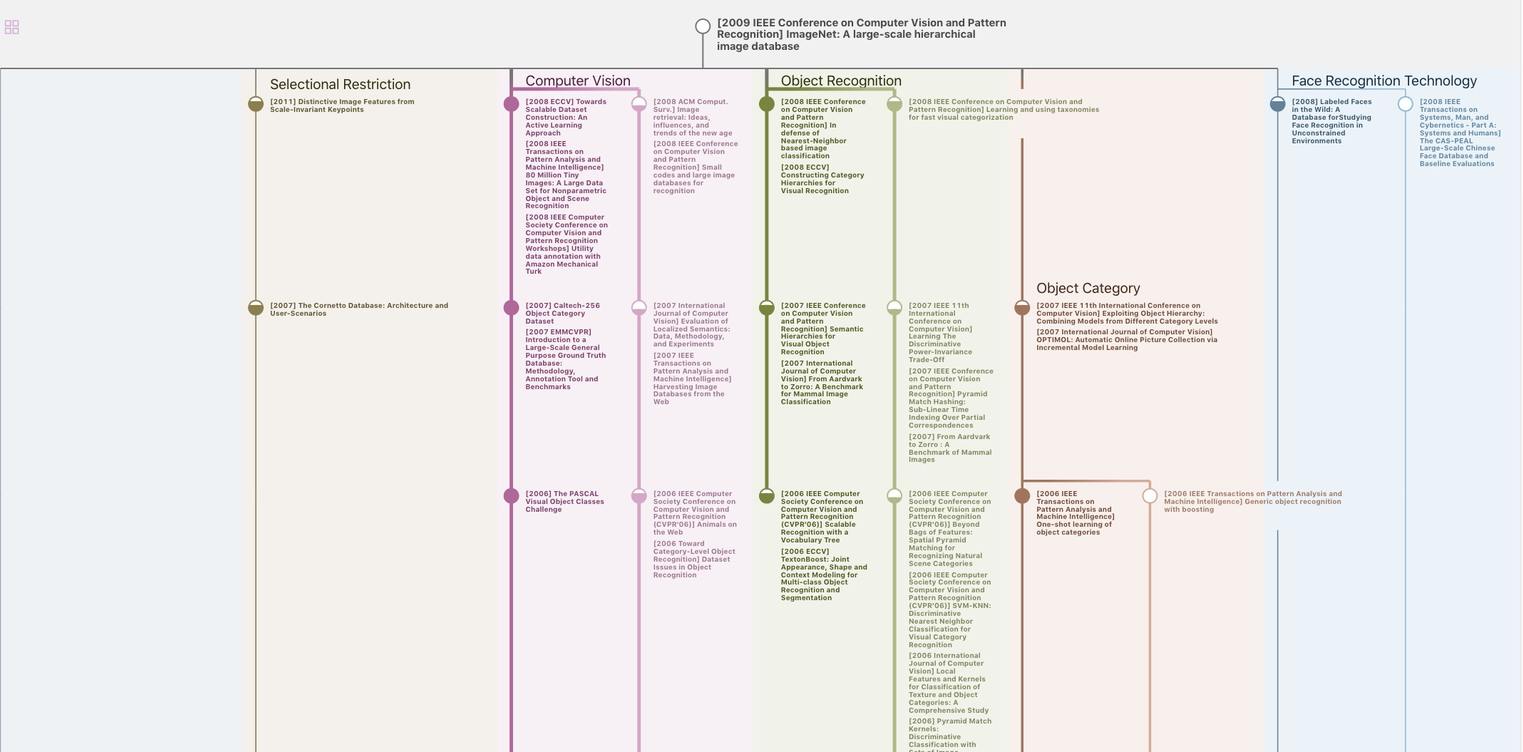
生成溯源树,研究论文发展脉络
Chat Paper
正在生成论文摘要