Predicting the Length of Stay of Cardiac Patients Based on Pre-Operative Variables-Bayesian Models vs. Machine Learning Models
HEALTHCARE(2024)
摘要
Length of stay (LoS) prediction is deemed important for a medical institution's operational and logistical efficiency. Sound estimates of a patient's stay increase clinical preparedness and reduce aberrations. Various statistical methods and techniques are used to quantify and predict the LoS of a patient based on pre-operative clinical features. This study evaluates and compares the results of Bayesian (simple Bayesian regression and hierarchical Bayesian regression) models and machine learning (ML) regression models against multiple evaluation metrics for the problem of LoS prediction of cardiac patients admitted to Tabba Heart Institute, Karachi, Pakistan (THI) between 2015 and 2020. In addition, the study also presents the use of hierarchical Bayesian regression to account for data variability and skewness without homogenizing the data (by removing outliers). LoS estimates from the hierarchical Bayesian regression model resulted in a root mean squared error (RMSE) and mean absolute error (MAE) of 1.49 and 1.16, respectively. Simple Bayesian regression (without hierarchy) achieved an RMSE and MAE of 3.36 and 2.05, respectively. The average RMSE and MAE of ML models remained at 3.36 and 1.98, respectively.
更多查看译文
关键词
length of stay,Bayesian inference,hierarchical Bayesian regression,machine learning
AI 理解论文
溯源树
样例
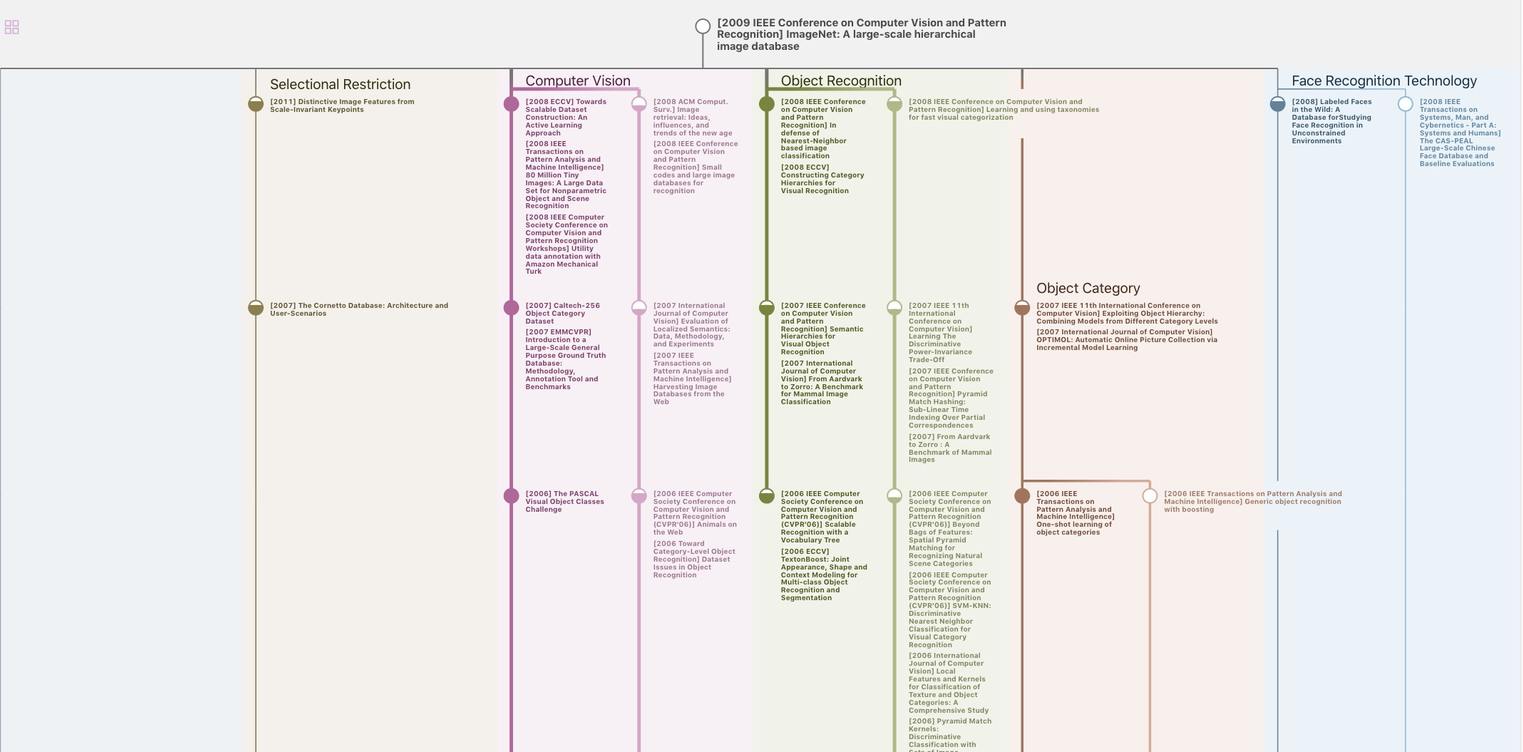
生成溯源树,研究论文发展脉络
Chat Paper
正在生成论文摘要