Are transformer-based models more robust than CNN-based models?
NEURAL NETWORKS(2024)
摘要
As the deployment of artificial intelligence (AI) models in real -world settings grows, their open -environment robustness becomes increasingly critical. This study aims to dissect the robustness of deep learning models, particularly comparing transformer -based models against CNN -based models. We focus on unraveling the sources of robustness from two key perspectives: structural and process robustness. Our findings suggest that transformer -based models generally outperform convolution -based models in robustness across multiple metrics. However, we contend that these metrics may not wholly represent true model robustness, such as the mean of corruption error. To better understand the underpinnings of this robustness advantage, we analyze models through the lens of Fourier transform and game interaction. From our insights, we propose a calibrated evaluation metric for robustness against real -world data, and a blur -based method to enhance robustness performance. Our approach achieves state-of-the-art results, with mCE scores of 2.1% on CIFAR-10-C, 12.4% on CIFAR-100-C, and 24.9% on TinyImageNet-C.
更多查看译文
关键词
Data augmentation,Model robustness,Deep learning,Image classification
AI 理解论文
溯源树
样例
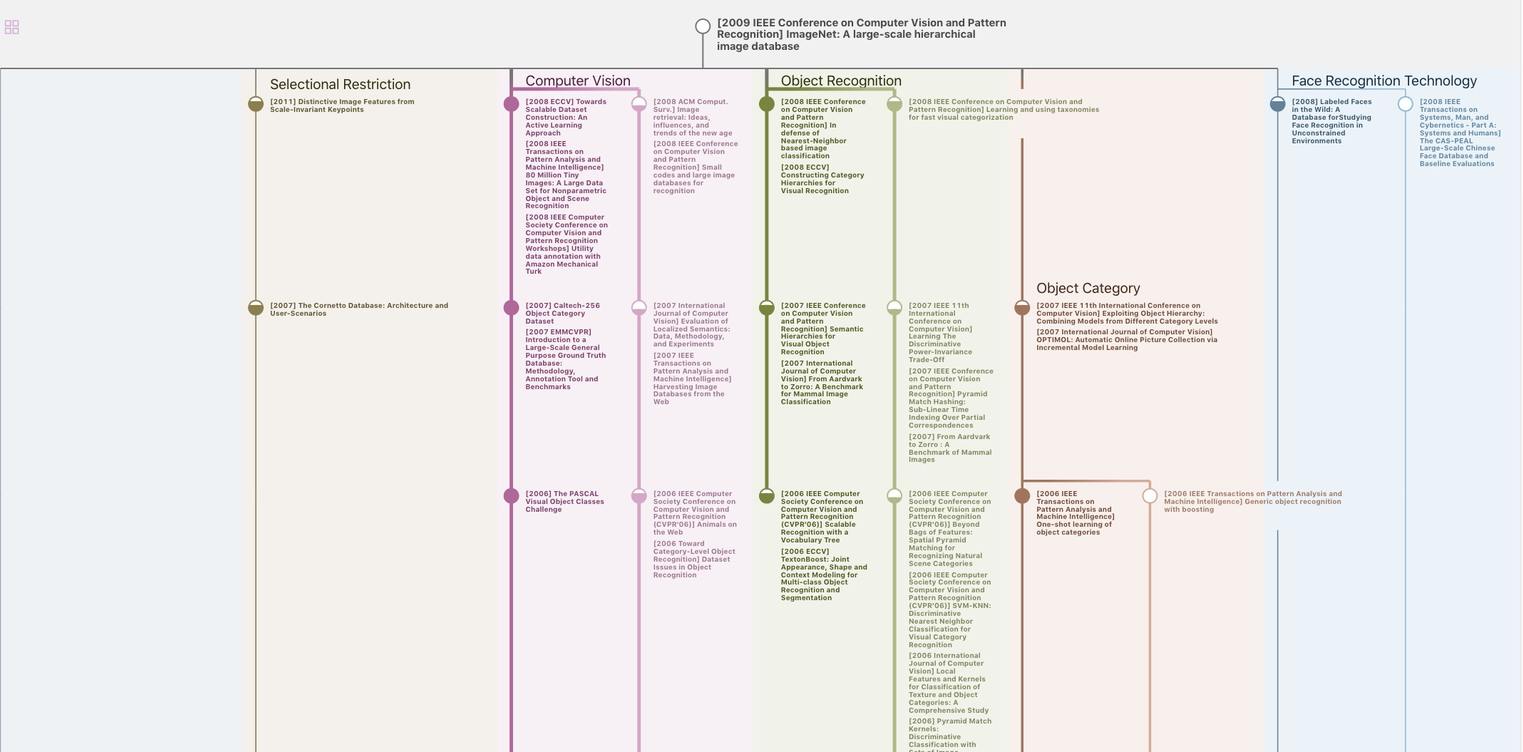
生成溯源树,研究论文发展脉络
Chat Paper
正在生成论文摘要