From anomaly detection to classification with graph attention and transformer for multivariate time series
ADVANCED ENGINEERING INFORMATICS(2024)
摘要
Numerous industrial environments and IoT systems in the real world contain a range of sensor devices. These devices, when in operation, produce a large amount of multivariate time series data. These data are interconnected and together reflect the status and changing trends of the devices and environment. To ensure the safety and stability of the working devices, it is of great research value to continuously monitor the generated data and perform accurate and rapid anomaly detection. Moreover, in some emergency situations, after detecting an anomaly, making a preliminary judgment and reporting the type of anomaly can save a lot of precious time and reduce property losses. Therefore, we propose an anomaly detection and classification architecture that can quickly perform sequence modeling. The architecture includes our proposed novel graph learning method and the high -efficiency Transformer model. We use two -stage adversarial training to train the anomaly detection model and apply the model to anomaly classification using a prototype network. Extensive experiments on four public datasets prove that our anomaly detection method outperforms other state-of-the-art methods. We also verify the accuracy of anomaly classification through case studies.
更多查看译文
关键词
Multivariate time series,Anomaly detection,Classification,Graph attention,Transformer
AI 理解论文
溯源树
样例
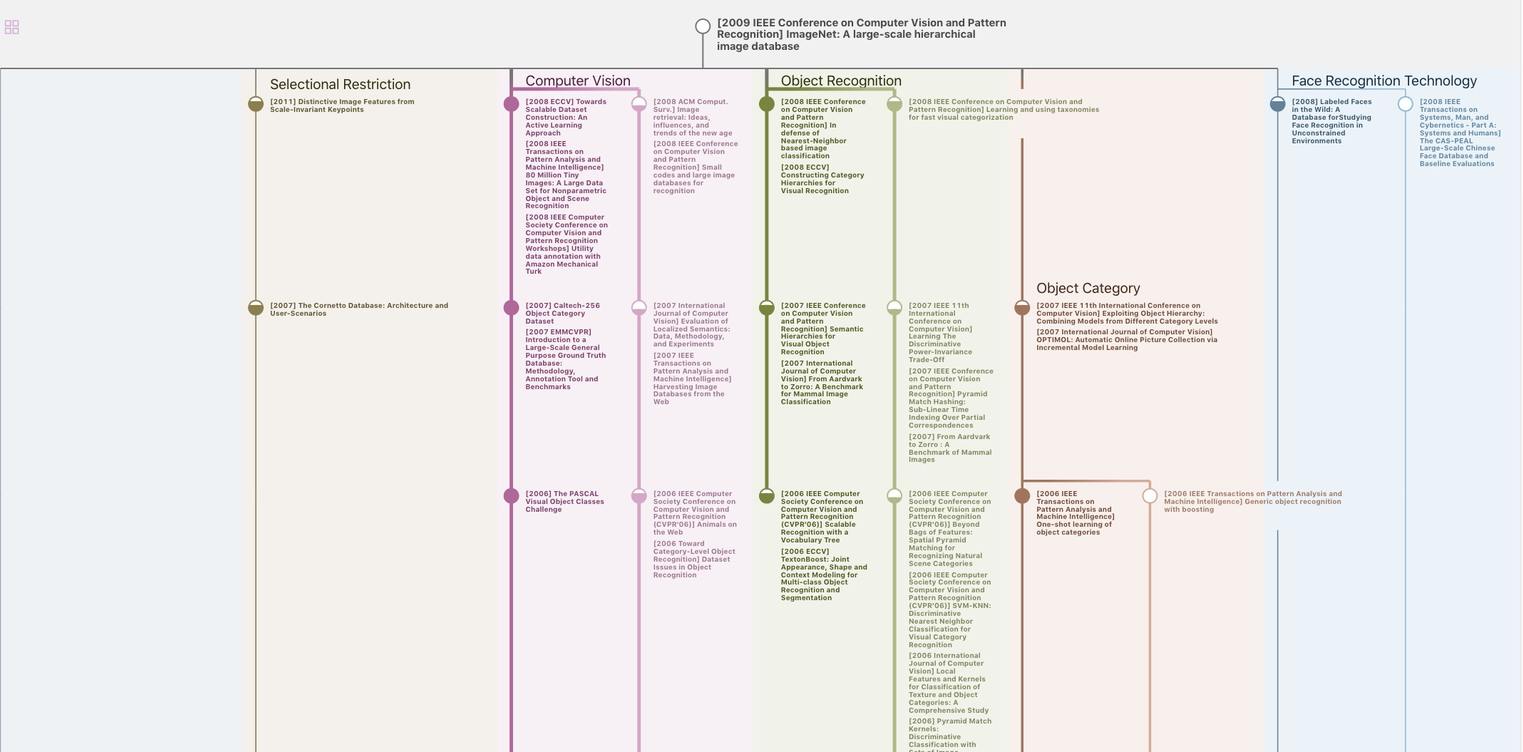
生成溯源树,研究论文发展脉络
Chat Paper
正在生成论文摘要