TPTrack: Strengthening tracking-by-detection methods from tracklet processing perspectives
COMPUTERS & ELECTRICAL ENGINEERING(2024)
摘要
As a fundamental task in computer vision, multi -object tracking (MOT) has gained increasing attention due to its commercial and academic potential. However, accurately tracking multiple objects is highly challenging. The problems of object occlusion, deformation, and real-time requirements have long been obstacles to be tackled in the field of multi -object tracking. In this paper, we first propose a novel approach to address the problem of tracklet fragmentation caused by occlusion and deformation by establishing a global linking model to obtain global information on trajectories. Then, we reduce camera jitter using camera motion compensation to more accurately locate objects in the moving scene. Next, to address the issue of linear interpolation that ignores a large amount of motion information, we utilize Gaussian process regression for smooth interpolation to fill in missing detections and reduce the noise covariance of the Kalman filter through adaptive computation. We integrate our work together and ultimately propose a simple, real-time, and robust multi -object tracker named TPTrack. Through extensive experiments, our proposed TPTrack achieves HOTA scores of 63.5, 61.7, and 56.8 on the MOT17, MOT20, and DanceTrack datasets, respectively. Notably, it exhibits a significant 19% enhancement over the state-of-the-art approach, specifically on the DanceTrack dataset. Furthermore, TPTrack operates at a speed of 33.7 FPS on a single GPU. The source code is available at https://github.com/godHhh/TPTrack.
更多查看译文
关键词
Tracking-by-detection,Multi-object tracking,Occlusion,Global information
AI 理解论文
溯源树
样例
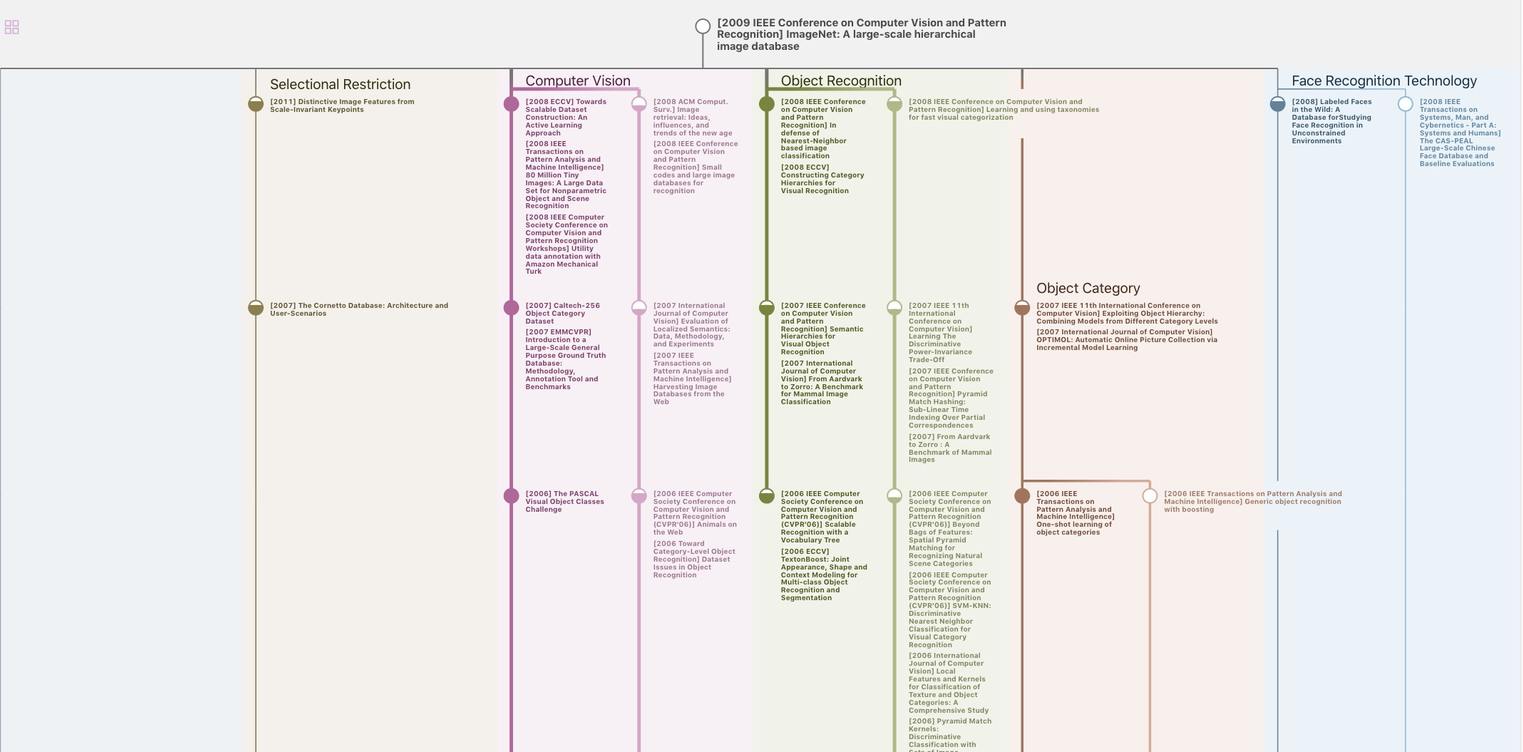
生成溯源树,研究论文发展脉络
Chat Paper
正在生成论文摘要