SCGRFuse: An infrared and visible image fusion network based on spatial/channel attention mechanism and gradient aggregation residual dense blocks
Engineering Applications of Artificial Intelligence(2024)
摘要
The goal of image fusion is to retain the strengths of different images in the fused result. However, existing fusion algorithms are often complex in design and overlook the influence of attention mechanisms on deep features. To address these issues, we propose an image fusion network based on spatial/channel attention mechanisms and gradient-aggregated residual dense blocks(SCGRFuse). Firstly, we design a novel gradient-aggregated residual dense block (GRXDB) that combines the advantages of ResNeXt and DenseNet, which integrating the Sobel and Laplacian operators to preserve both strong and weak texture features. Then, we introduce spatial and channel attention mechanisms to refine the channel and spatial information of feature maps, enhancing their information capturing capability. Additionally, we leverage a pooling fusion block to merge the refined spatial and channel feature maps, yielding high-quality fusion features. Compared to the existing state-of-the-art methods, experimental results on the MSRS, RoadScene and TNO datasets demonstrate the outstanding fusion performance of our proposed approach. In addition, in the task-driven experiments, SCGRFuse achieved an mIoU accuracy of 71.37%.
更多查看译文
关键词
Image fusion,Infrared image,Visible image,Attention mechanisms,Gradient-aggregation residual dense block
AI 理解论文
溯源树
样例
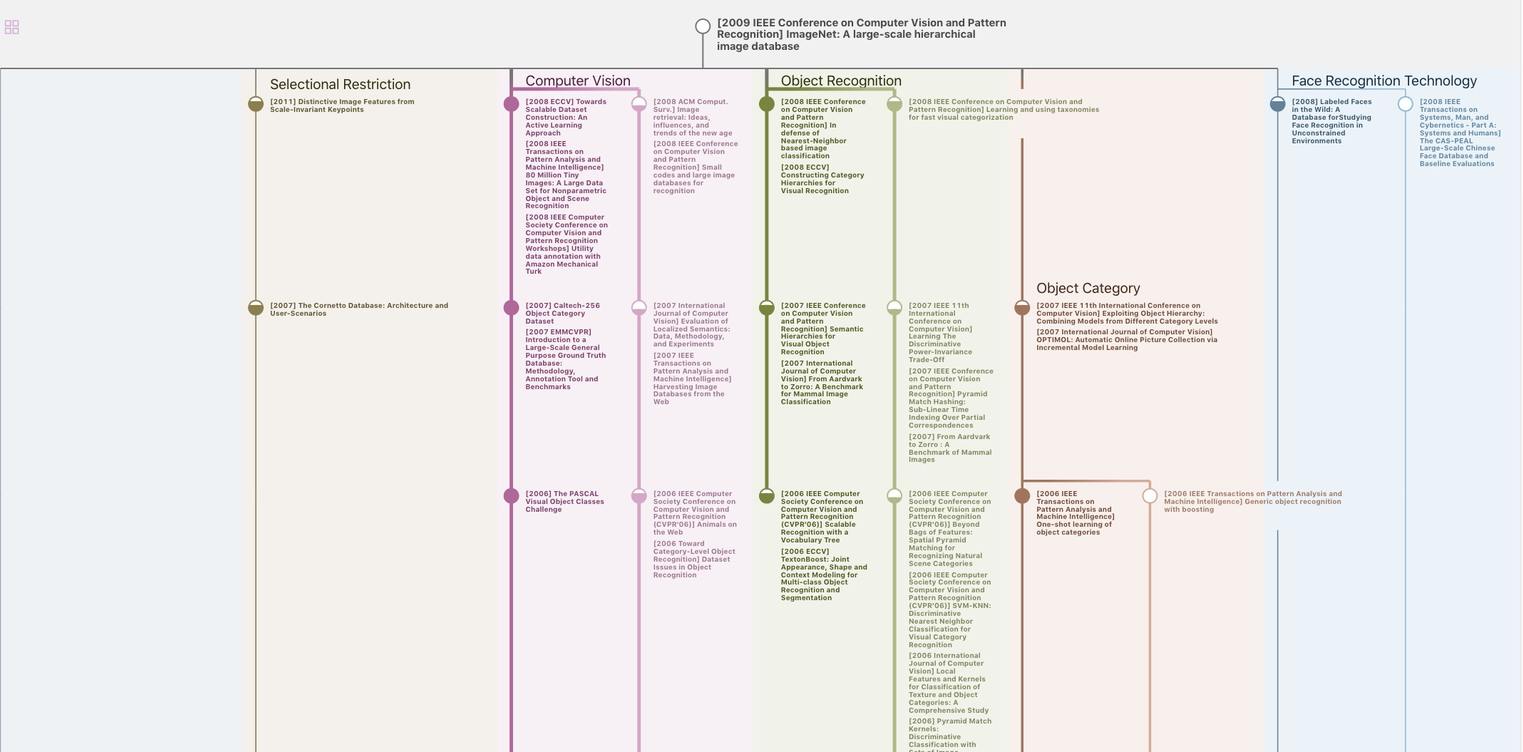
生成溯源树,研究论文发展脉络
Chat Paper
正在生成论文摘要