Multi-Item Continuous Influence Maximization.
2023 IEEE International Conference on Big Data (BigData)(2023)
摘要
Influence maximization in social networks plays an important role in many real-world applications, e.g., viral marketing and trend detection. Current research on influence maximization focuses on promoting one single item/product under discrete settings. The basic assumption is that each user should be chosen to receive either a full incentive (as seed users) or no incentive (as non-seed users) on one single product (e.g., iPhone XS). However, many online shopping platforms, such as Amazon, sell multiple products (e.g., iPhone, iPhone cases, Android phones, etc.) and promoters can give different percentage discounts to different users on different products. In this paper, we study the problem of multi-item influence maximization under continuous settings, where the influence diffusion includes multiple items and each user is given a different level of incentive (e.g., percentage discount) on each item. This problem is challenging and different from previous work on influence maximization since different levels of incentive can lead to different levels of influence on each user. Moreover, not only users can influence each other through a social network, but items can also influence each other through an item network. We propose a novel solution, called MMF (Multi-item Mean-Field), for the multi-item continuous influence maximization problem, based on the mean-field approximation of Ising networks. The proposed MMF model can effectively maximize the total purchase of multiple items by taking social influence as well as item influence into account. The experiments demonstrate the effectiveness of the approach on both simulated and real-world datasets.
更多查看译文
关键词
Influence maximization,Ising network,viral marketing,item influence,social networks
AI 理解论文
溯源树
样例
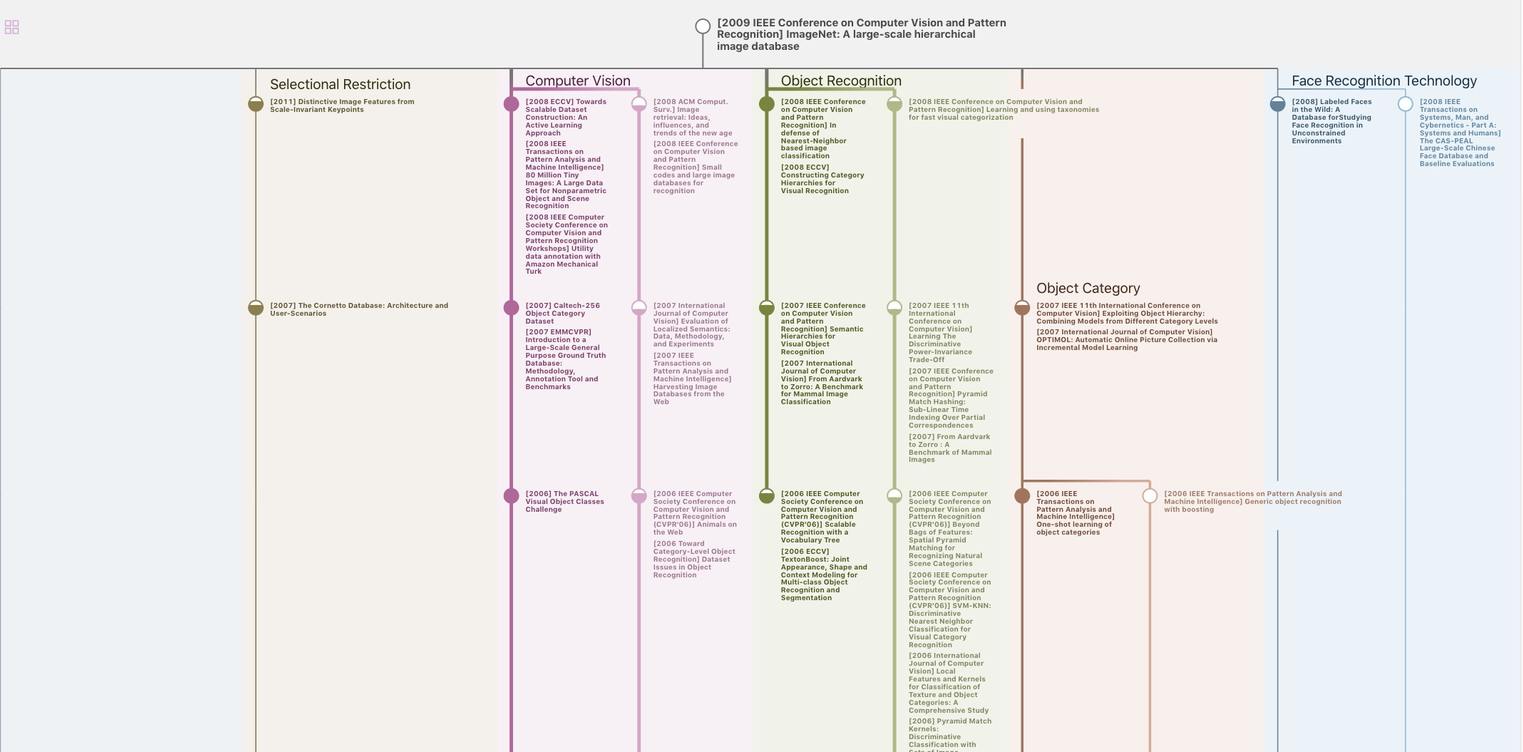
生成溯源树,研究论文发展脉络
Chat Paper
正在生成论文摘要