Text-guided Fourier Augmentation for long-tailed recognition
PATTERN RECOGNITION LETTERS(2024)
摘要
Real-world data often exhibits a long-tailed distribution in practical scenarios. However, deep learning models usually face challenges when it comes to effectively identifying infrequent classes amidst the abundance of prevalent ones. The fundamental issue lies in the scarcity of available information for tail classes. A highly intuitive approach is to uncover a greater amount of valuable information specifically tailored to these tail classes. We find that textual information of class names and frequency domain information of images are ignored by previous works in long-tailed visual recognition. Therefore, we propose a TextGuided Fourier Augmentation (TGFA) method with the aid of language models and the Fourier transform to excavate more useful information for tail classes. Extensive experiments demonstrate that our proposed method effectively enriches training data on-the-fly, allowing for an end-to-end one-stage supervised contrastive learning framework that surpasses other methods including two-stage or multi-experts methods, in terms of efficiency and performance.
更多查看译文
关键词
Long-tailed visual recognition,Language models,Fourier transform,Imbalanced data
AI 理解论文
溯源树
样例
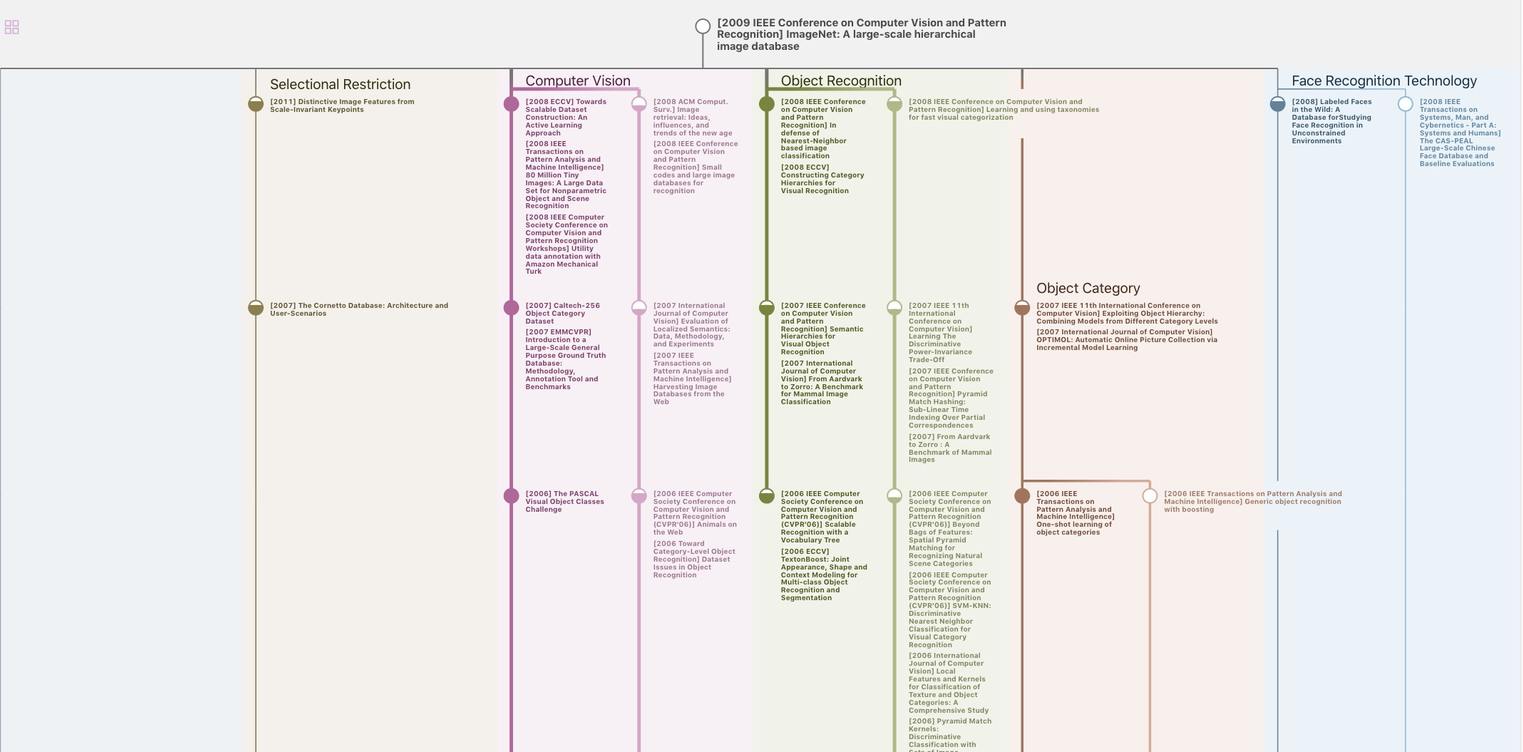
生成溯源树,研究论文发展脉络
Chat Paper
正在生成论文摘要