Composite Deep Learning Architecture for Vehicle Classification Using Vision Transformers and Wheel Position Features
SN Computer Science(2024)
摘要
Vehicle classification holds significant importance in various domains such as infrastructure design and freight analysis. This study presents an innovative composite deep-learning framework for accurate vehicle classification. The framework exploits two distinct types of features extracted from vehicle images: (1) high-level encodings from state-of-the-art vision transformers (ViTs), and (2) localized vehicle wheel position features obtained through real-time object detection models. The former encapsulates global and semantic characteristics, while the latter focuses on specific wheel (axle) positions. Within this composite model paradigm, we evaluate and compare the efficacy of four ViT models: the original ViT, Cross ViT, Transformer-in-Transformer, and Swin Transformer. Similarly, we assess four object detection models for extracting wheel position features: two Faster R-CNN models (with ResNet-50 and MobileNetv3 backbones) and two YOLO models (YOLOv4 and YOLOR). The ViT encodings and wheel position features are then combined and channeled into a multi-layer perceptron classifier for precise vehicle classification. To enhance the ViT model's effectiveness, we employ a wheel masking strategy during its training, which acts as a regularizer, promoting robust and complementary encodings. Our experimental results reveal that introducing randomness by masking a single wheel significantly enhances the inference performance across all composite models. However, masking more wheels introduces excessive noise and causes performance degradation. Furthermore, initializing ViT encoders with pretrained weights through self-supervised methods leads to additional performance improvements. Notably, our best model achieves an impressive Top-1 classification accuracy of 96.7% when categorizing 13 vehicle classes as defined by the Federal Highway Administration. The results underscore the efficacy of the proposed composite architecture in achieving high precision in vehicle classification tasks.
更多查看译文
关键词
Vehicle classification,Vision transformers,Self-supervised learning,Feature fusion,Random wheel masking,Regularization,Object detection
AI 理解论文
溯源树
样例
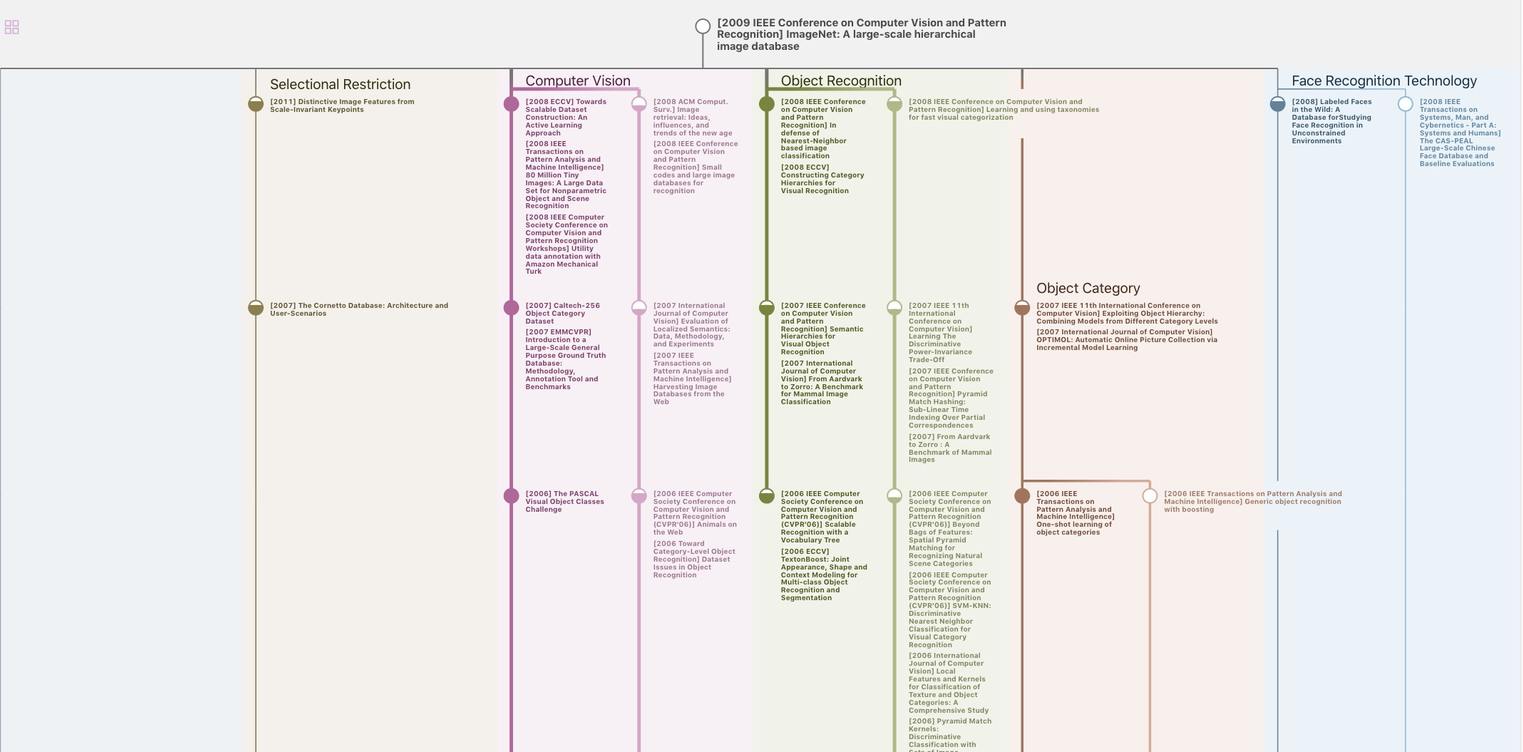
生成溯源树,研究论文发展脉络
Chat Paper
正在生成论文摘要