Depression detection using cascaded attention based deep learning framework using speech data
Multimedia Tools and Applications(2024)
摘要
Efficient detection of depression is a challenging scenario in the field of speech signal processing. Since the speech signals provide a better diagnosis of depression, a significant methodology is required for detection. However, manual examination performed by radiologists can be time-consuming and may not be feasible in complex circumstances. Diverse detection methodologies have been proposed previously, but they are found to be less accurate, time-consuming and lead over maximized error rates. The proposed research article presents an effective and automatic deep learning-based depression detection using speech signal data. The steps involved in depression prediction are data acquisition, pre-processing, Feature Extraction, Feature selection and classification. The initial step in depression detection is data acquisition, which aims at collecting speech signals from the Distress Analysis Interview Corpus (DAIC-WOZ) and Sonde Health-free speech (SH2-FS) datasets. The collected data are pre-processed through MS_DWT (Multi-stage Discrete Wavelet Transform) to offer noise-free signals and improved signal quality. The relevant features required for processing the speech signal are extracted through Hilbert Huang (H-H) transform linear prediction cepstrum coefficient (LPCC), fundamental frequency, formants, speaking rate and Mel frequency cepstral coefficients (MFCC). From the extracted features, ideal features required for enhancing the detection accuracy are selected using the Price Auction optimization algorithm (PAOA). Finally, the depression and non-depression states are classified using deep convolutional Attention Cascaded two directional long short-term memory (DAttn_Conv 2D LSTM) with a softmax classifier. The overall accuracy obtained in classifying the depressed and non-depressed classes is 97.82% and 98.91%, respectively.
更多查看译文
关键词
Speech signals,Multi-stage Discrete Wavelet Transform,Auction Optimization,Deep convolutional Attention,Depression,And Non-depression
AI 理解论文
溯源树
样例
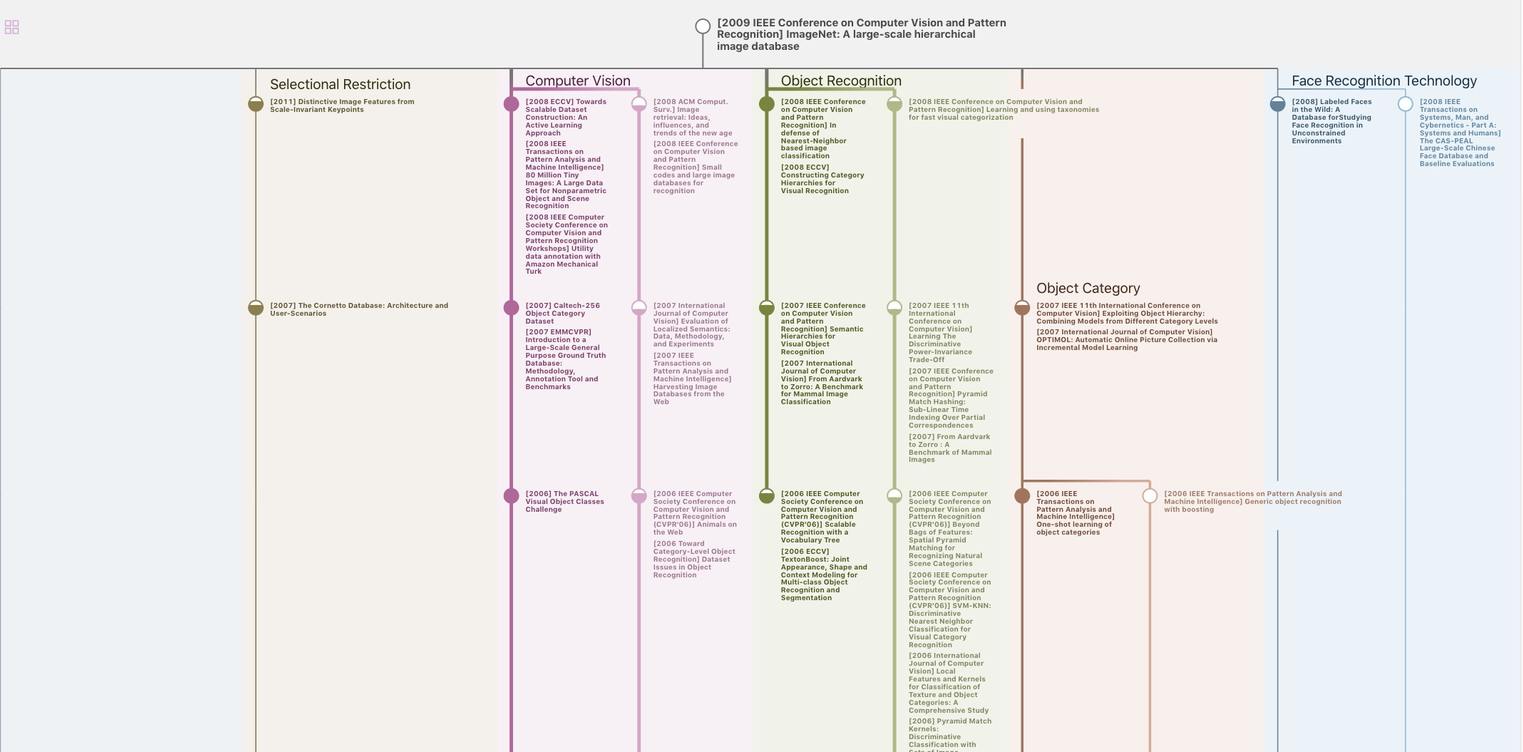
生成溯源树,研究论文发展脉络
Chat Paper
正在生成论文摘要