Fuzzy information gain ratio-based multi-label feature selection with label correlation
International Journal of Machine Learning and Cybernetics(2024)
摘要
Multi-label feature selection aims to mitigate the curse of dimensionality in multi-label data by selecting a smaller subset of features from the original set for classification. Existing multi-label feature selection algorithms frequently neglect the inherent uncertainty in multi-label data and fail to adequately consider the relationships between features and labels when assessing the importance of features. In response to this challenge, a Fuzzy Information Gain Ratio-based multi-label feature selection considering Label Correlation (FIGR_LC) algorithm is proposed. FIGR_LC evaluates feature importance by combining the relationship between features and individual labels, as well as the correlation between features and label sets. Subsequently, a feature ranking is established based on these feature weights. Experimental results substantiate the effectiveness of FIGR_LC, showcasing its superiority over several established feature selection methods.
更多查看译文
关键词
Multi-label feature selection,Fuzzy information gain ratio,Fuzzy rough sets
AI 理解论文
溯源树
样例
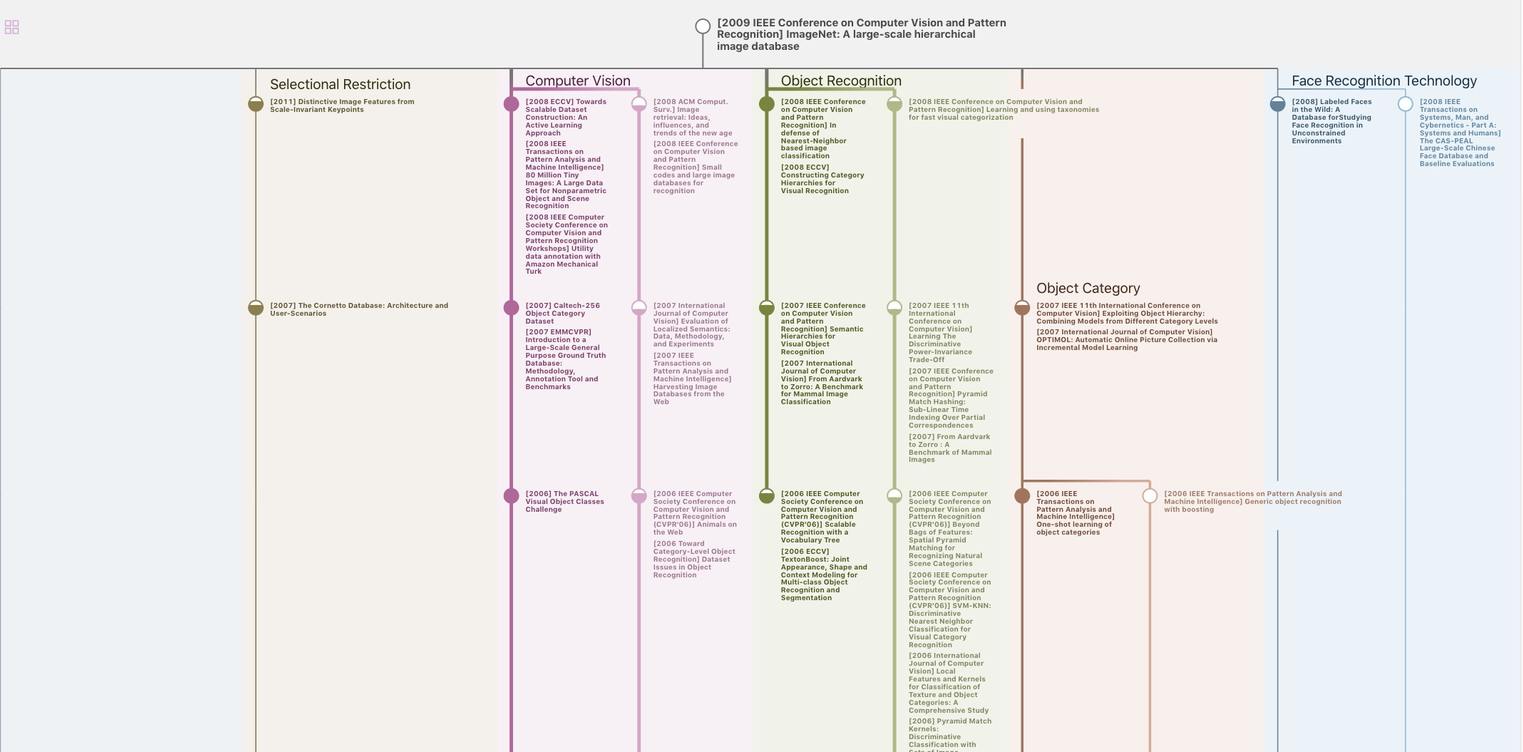
生成溯源树,研究论文发展脉络
Chat Paper
正在生成论文摘要