DPDFormer: A Coarse-to-Fine Model for Monocular Depth Estimation.
ACM Trans. Multim. Comput. Commun. Appl.(2024)
摘要
Monocular depth estimation attracts great attention from computer vision researchers for its convenience in acquiring environment depth information. Recently classification-based MDE methods show its promising performance and begin to act as an essential role in many multi-view applications such as reconstruction and 3D object detection. However, existed classification-based MDE models usually apply fixed depth range discretization strategy across a whole scene. This fixed depth range discretization leads to the imbalance of discretization scale among different depth ranges, resulting in the inexact depth range localization. In this article, to alleviate the imbalanced depth range discretization problem in classification-based monocular depth estimation (MDE) method we follow the coarse-to-fine principle and propose a novel depth range discretization method called depth post-discretization (DPD). Based on a coarse depth anchor roughly indicating the depth range, the DPD generates the depth range discretization adaptively for every position. The depth range discretization with DPD is more fine-grained around the actual depth, which is beneficial for locating the depth range more precisely for each scene position. Besides, to better manage the prediction of the coarse depth anchor and depth probability distribution for calculating the final depth, we design a dual-decoder transformer-based network, i.e., DPDFormer, which is more compatible with our proposed DPD method. We evaluate DPDFormer on popular depth datasets NYU Depth V2 and KITTI. The experimental results prove the superior performance of our proposed method.
更多查看译文
关键词
Monocular depth estimation,coarse-to-fine,depth post-discretization,DPDformer
AI 理解论文
溯源树
样例
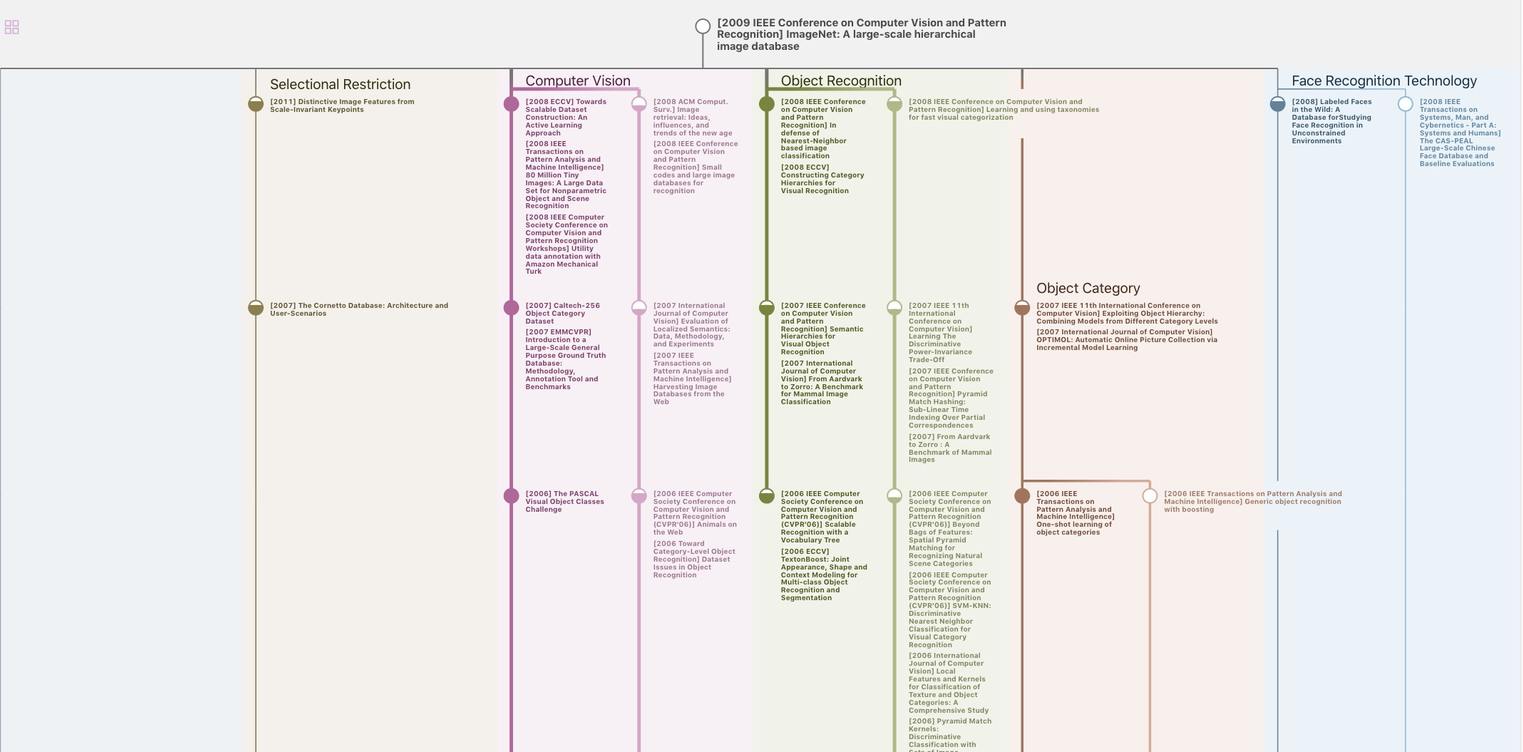
生成溯源树,研究论文发展脉络
Chat Paper
正在生成论文摘要