An Unsupervised Transformer-Based Multivariate Alteration Detection Approach for Change Detection in VHR Remote Sensing Images
IEEE JOURNAL OF SELECTED TOPICS IN APPLIED EARTH OBSERVATIONS AND REMOTE SENSING(2024)
摘要
Multitemporal change detection (CD) plays a crucial role in the remote sensing application field. In recent years, supervised deep learning methods have shown excellent performance in detecting changes in very-high-resolution (VHR) images. However, these methods require a large number of labeled samples for training, making the process time-consuming and labor-intensive. Unsupervised approaches are more attractive in practical applications since they can produce a CD map without relying on any ground reference or prior knowledge. In this article, we propose a novel unsupervised CD approach, named transformer-based multivariate alteration detection (trans-MAD). It utilizes a pre-detection strategy that combines the compressed change vector analysis and the iteratively reweighted multivariate alteration detection (IR-MAD) to generate reliable pseudotraining samples. More accurate and robust CD results can be achieved by leveraging the IR-MAD to detect insignificant changes and by incorporating the transformer-based attention mechanism to model the difference or similarity between two distant pixels in an image. The proposed trans-MAD approach was validated on two VHR bitemporal satellite remote sensing datasets, and the obtained experimental results demonstrated its superiority comparing with the state-of-the-art unsupervised CD methods.
更多查看译文
关键词
Change detection (CD),deep learning,iteratively reweighted multivariate alteration detection (IR-MAD),transformer,unsupervised,very-high-resolution (VHR) remote sensing images
AI 理解论文
溯源树
样例
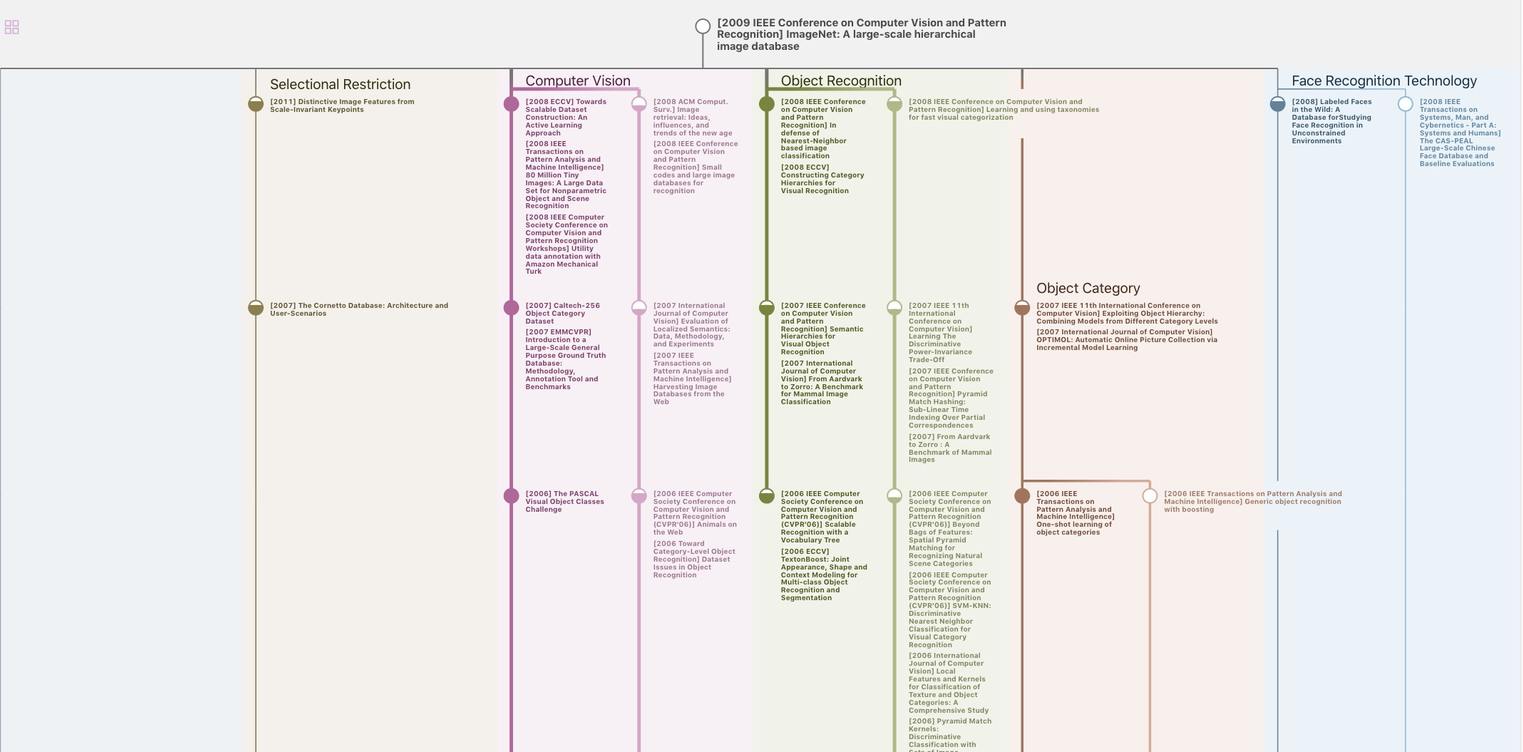
生成溯源树,研究论文发展脉络
Chat Paper
正在生成论文摘要