Generalized building energy and carbon emissions benchmarking with post-prediction analysis
DEVELOPMENTS IN THE BUILT ENVIRONMENT(2024)
摘要
This study proposes a generalized building energy and carbon emissions benchmarking approach. Leveraging eleven years of real-world data from twelve U.S. cities, an advanced ensemble learning model is employed to predict building site, source energy, and carbon emissions. The generalizability is validated across seven climate zones, yielding R2 values ranging from 0.3 to 0.9. Additionally, a post-prediction analysis method is proposed that categorizes actual and predicted values into well-estimated, underestimated, and overestimated groups by an [-15%, 15%] acceptance interval (AI). This approach allows for assessing predictions related to energy, building types, and climate zones. The findings indicate the feasibility of a reliable generalized benchmarking tool. It's been observed that energy in most underestimated groups tends to be higher than that of their overestimated counterparts. Over 63% of buildings' predictions perform within the well-estimated group by [-15%, 15%] AI.
更多查看译文
关键词
Generalized energy benchmarking,Carbon emissions,Data -driven,Machine learning,Big data,Post-prediction analysis
AI 理解论文
溯源树
样例
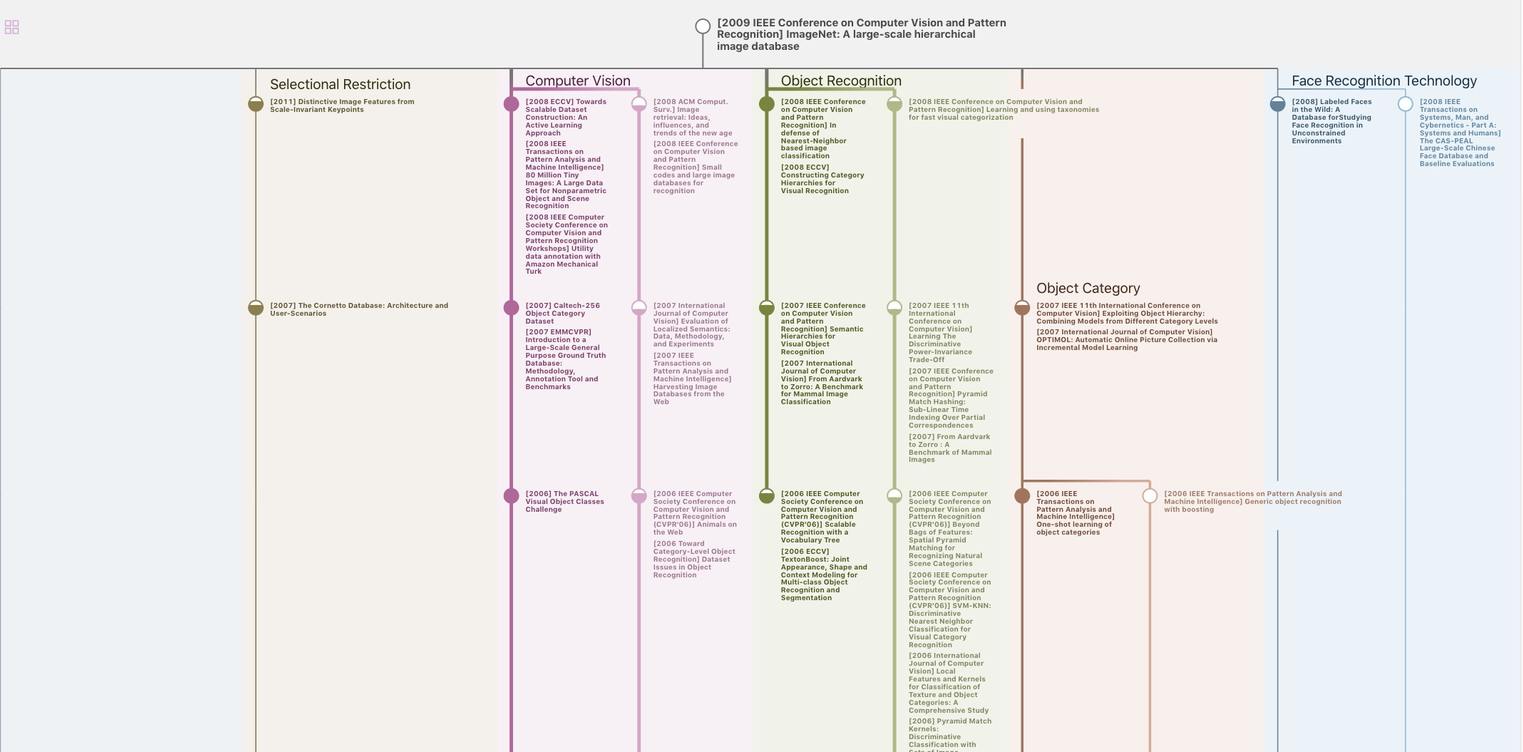
生成溯源树,研究论文发展脉络
Chat Paper
正在生成论文摘要