Fast and Scalable Network Slicing by Integrating Deep Learning with Lagrangian Methods
IEEE CONFERENCE ON GLOBAL COMMUNICATIONS, GLOBECOM(2023)
摘要
Network slicing is a key technique in 5G and beyond for efficiently supporting diverse services. Many network slicing solutions rely on deep learning to manage complex and high-dimensional resource allocation problems. However, deep learning models suffer limited generalization and adaptability to dynamic slicing configurations. In this paper, we propose a novel framework that integrates constrained optimization methods and deep learning models, resulting in strong generalization and superior approximation capability. Based on the proposed framework, we design a new neural-assisted algorithm to allocate radio resources to slices to maximize the network utility under interslice resource constraints. The algorithm exhibits high scalability, accommodating varying numbers of slices and slice configurations with ease. We implement the proposed solution in a system-level network simulator and evaluate its performance extensively by comparing it to state-of-the-art solutions including deep reinforcement learning approaches. The numerical results show that our solution obtains near-optimal quality-of-service satisfaction and promising generalization performance under different network slicing scenarios.
更多查看译文
关键词
Deep Learning,Lagrangian Method,Network Slicing,Resource Allocation,Service Quality,Deep Learning Models,Constrained Optimization,Deep Reinforcement Learning,Superior Capability,Resource Allocation Problem,High Scalability,Strong Generalization,Network Utility,Deep Reinforcement Learning Approach,System-level Simulation,Optimization Problem,Upper Bound,Deep Neural Network,Partial Differential,Network System,Deep Neural Network Model,Time Slot,Neighboring Points,Optimal Resource Allocation,Local Optimum,Key Performance Indicators,Network Scenarios,Achievable Throughput,Automatic Differentiation,Delay Requirements
AI 理解论文
溯源树
样例
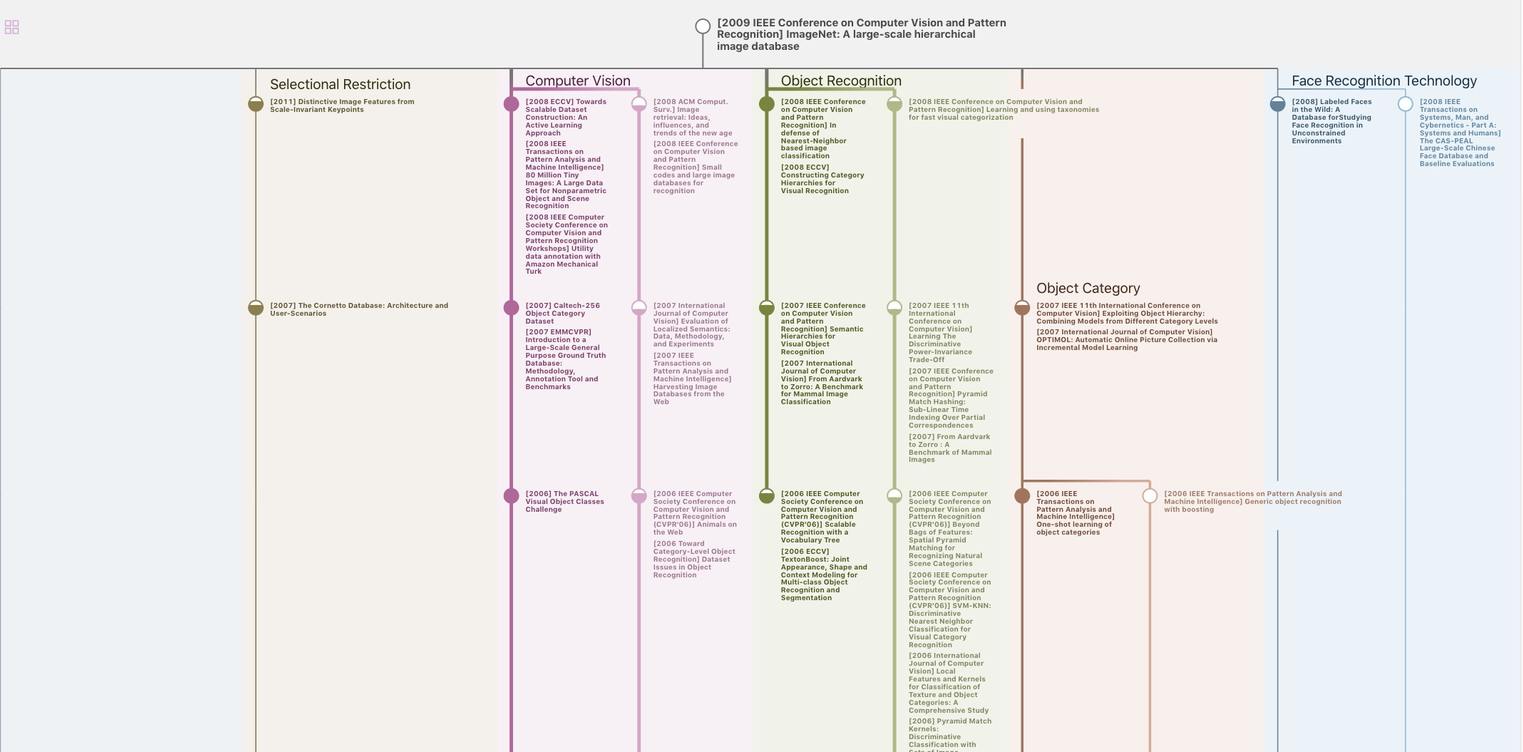
生成溯源树,研究论文发展脉络
Chat Paper
正在生成论文摘要