Back-stepping Experience Replay with Application to Model-free Reinforcement Learning for a Soft Snake Robot
CoRR(2024)
摘要
In this paper, we propose a novel technique, Back-stepping Experience Replay
(BER), that is compatible with arbitrary off-policy reinforcement learning (RL)
algorithms. BER aims to enhance learning efficiency in systems with approximate
reversibility, reducing the need for complex reward shaping. The method
constructs reversed trajectories using back-stepping transitions to reach
random or fixed targets. Interpretable as a bi-directional approach, BER
addresses inaccuracies in back-stepping transitions through a distillation of
the replay experience during learning. Given the intricate nature of soft
robots and their complex interactions with environments, we present an
application of BER in a model-free RL approach for the locomotion and
navigation of a soft snake robot, which is capable of serpentine motion enabled
by anisotropic friction between the body and ground. In addition, a dynamic
simulator is developed to assess the effectiveness and efficiency of the BER
algorithm, in which the robot demonstrates successful learning (reaching a 100
success rate) and adeptly reaches random targets, achieving an average speed
48
更多查看译文
AI 理解论文
溯源树
样例
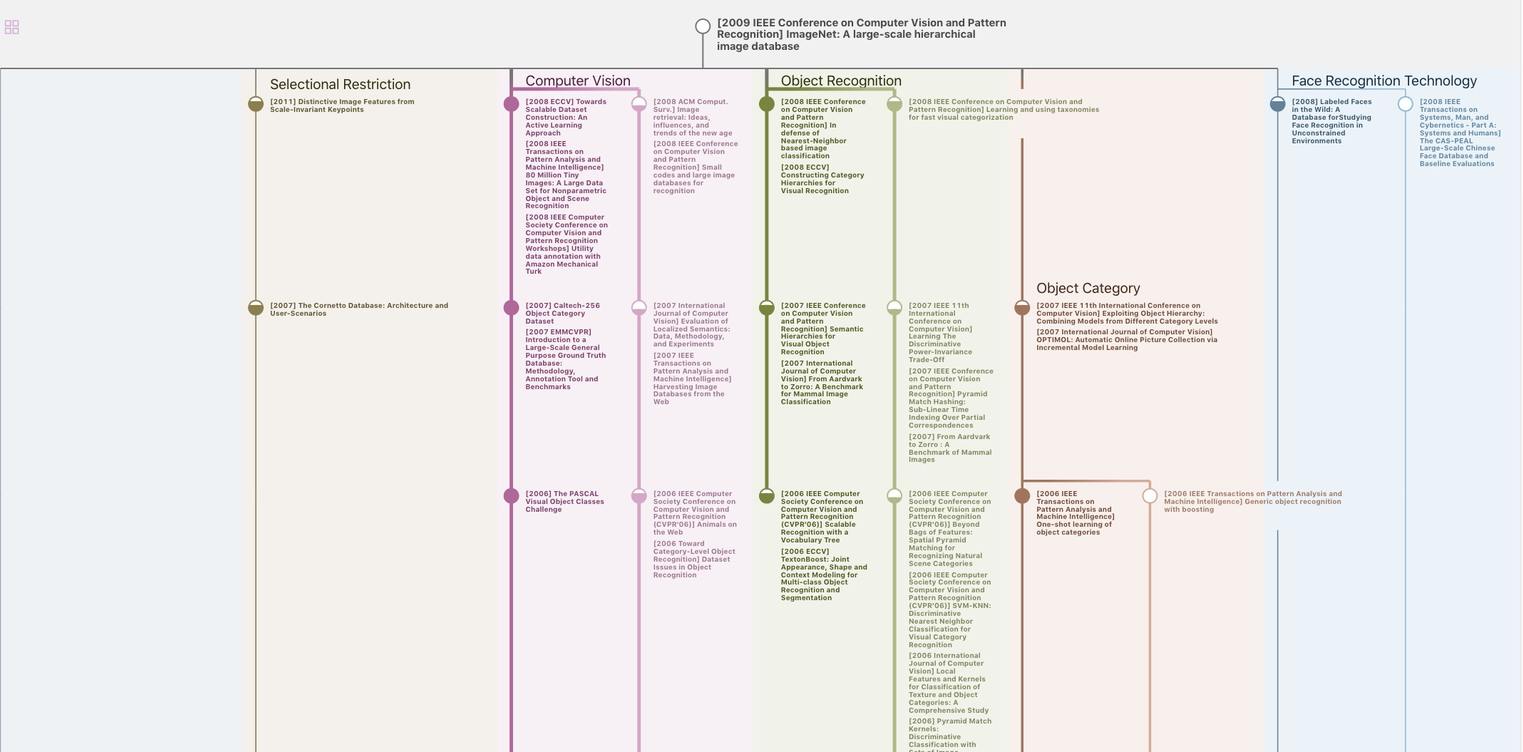
生成溯源树,研究论文发展脉络
Chat Paper
正在生成论文摘要