Accelerating Approximate Thompson Sampling with Underdamped Langevin Monte Carlo
CoRR(2024)
摘要
Approximate Thompson sampling with Langevin Monte Carlo broadens its reach
from Gaussian posterior sampling to encompass more general smooth posteriors.
However, it still encounters scalability issues in high-dimensional problems
when demanding high accuracy. To address this, we propose an approximate
Thompson sampling strategy, utilizing underdamped Langevin Monte Carlo, where
the latter is the go-to workhorse for simulations of high-dimensional
posteriors. Based on the standard smoothness and log-concavity conditions, we
study the accelerated posterior concentration and sampling using a specific
potential function. This design improves the sample complexity for realizing
logarithmic regrets from Õ(d) to Õ(√(d)). The scalability and robustness of our algorithm are also
empirically validated through synthetic experiments in high-dimensional bandit
problems.
更多查看译文
AI 理解论文
溯源树
样例
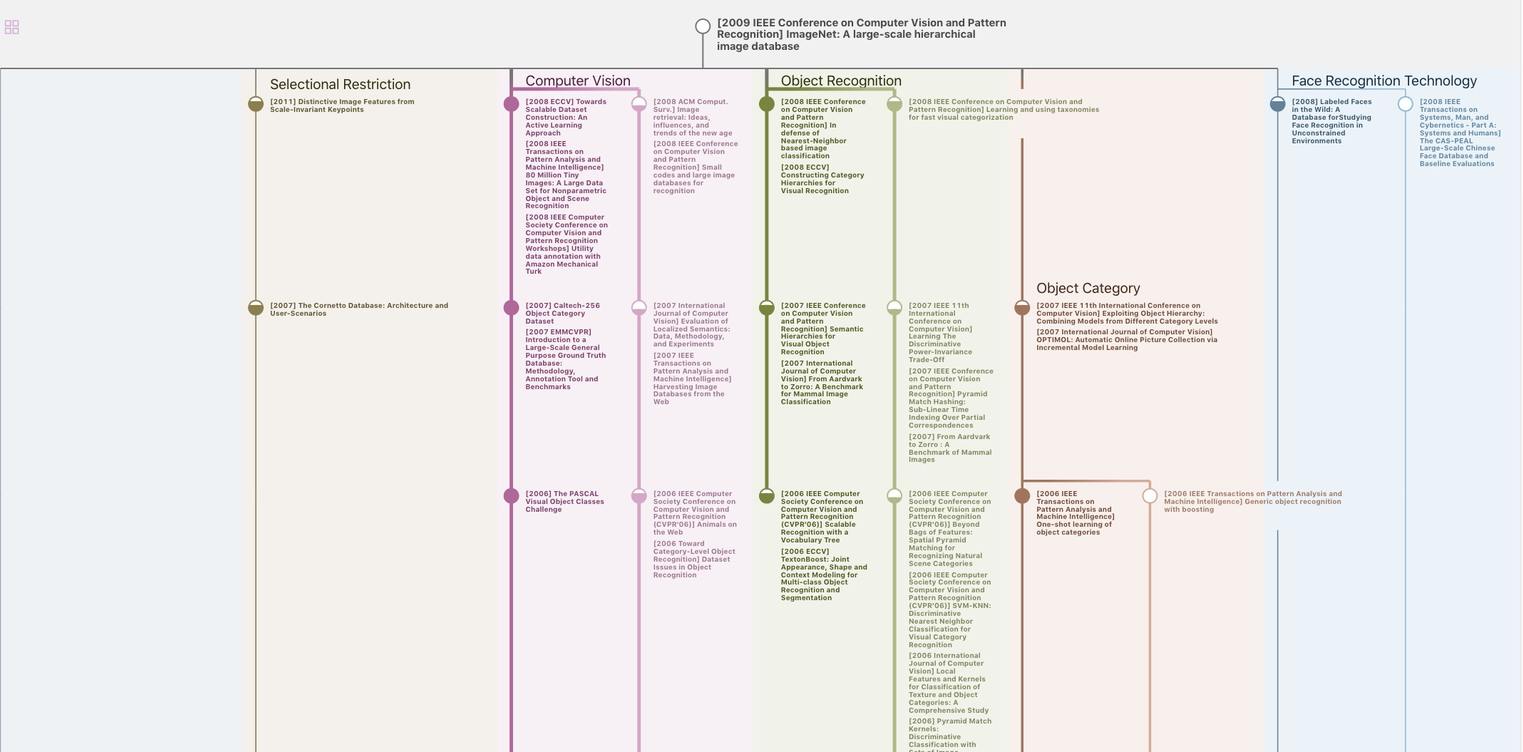
生成溯源树,研究论文发展脉络
Chat Paper
正在生成论文摘要