Comparison of Model Output Statistics and Neural Networks to Postprocess Wind Gusts
arxiv(2024)
摘要
Wind gust prediction plays an important role in warning strategies of
national meteorological services due to the high impact of its extreme values.
However, forecasting wind gusts is challenging because they are influenced by
small-scale processes and local characteristics. To account for the different
sources of uncertainty, meteorological centers run ensembles of forecasts and
derive probabilities of wind gusts exceeding a threshold. These probabilities
often exhibit systematic errors and require postprocessing. Model Output
Statistics (MOS) is a common operational postprocessing technique, although
more modern methods such as neural network-bases approaches have shown
promising results in research studies. The transition from research to
operations requires an exhaustive comparison of both techniques. Taking a first
step into this direction, our study presents a comparison of a postprocessing
technique based on linear and logistic regression approaches with different
neural network methods proposed in the literature to improve wind gust
predictions, specifically distributional regression networks and Bernstein
quantile networks. We further contribute to investigating optimal design
choices for neural network-based postprocessing methods regarding changes of
the numerical model in the training period, the use of persistence predictors,
and the temporal composition of training datasets. The performance of the
different techniques is compared in terms of calibration, accuracy, reliability
and resolution based on case studies of wind gust forecasts from the
operational weather model of the German weather service and observations from
170 weather stations.
更多查看译文
AI 理解论文
溯源树
样例
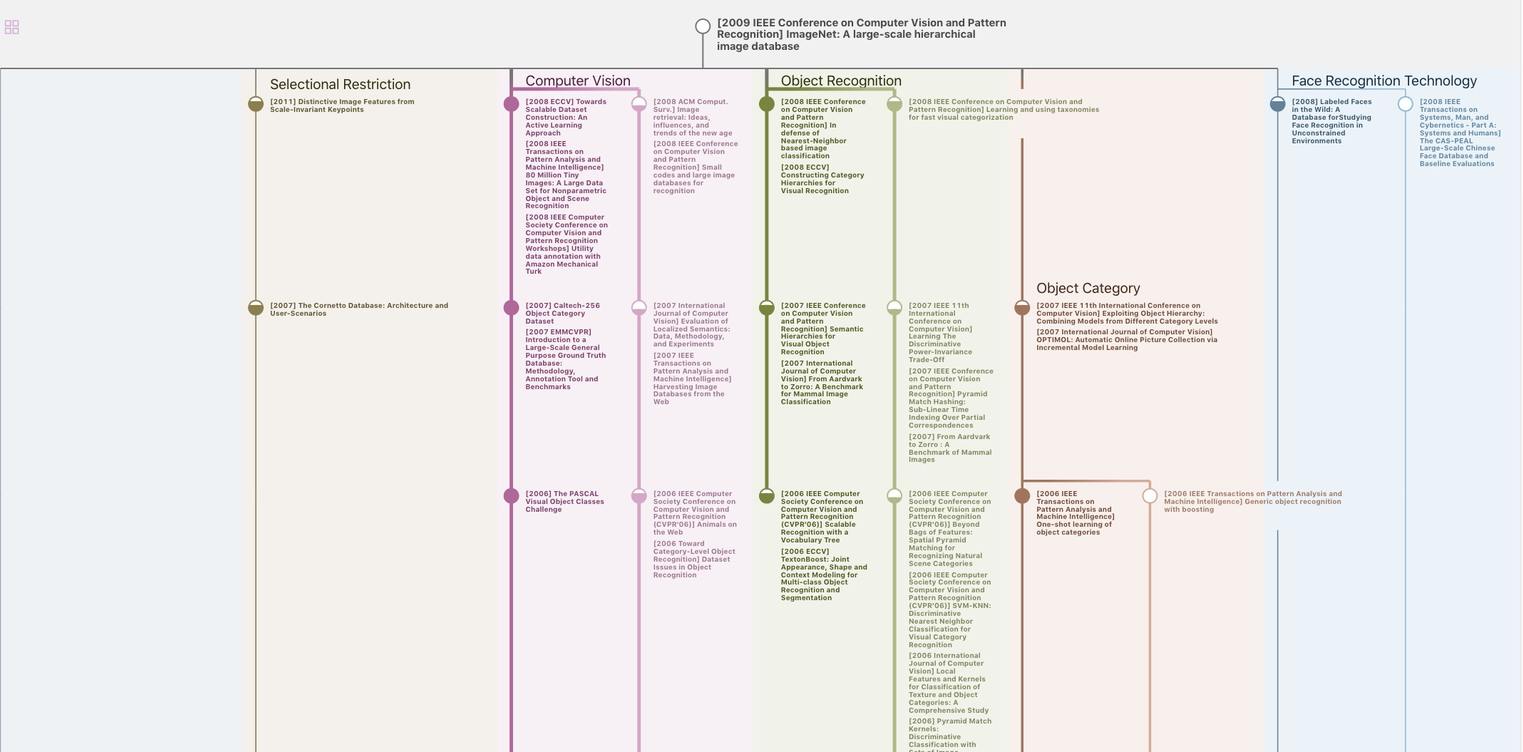
生成溯源树,研究论文发展脉络
Chat Paper
正在生成论文摘要