Dynamic Semantic Compression for CNN Inference in Multi-access Edge Computing: A Graph Reinforcement Learning-based Autoencoder
CoRR(2024)
摘要
This paper studies the computational offloading of CNN inference in dynamic
multi-access edge computing (MEC) networks. To address the uncertainties in
communication time and computation resource availability, we propose a novel
semantic compression method, autoencoder-based CNN architecture (AECNN), for
effective semantic extraction and compression in partial offloading. In the
semantic encoder, we introduce a feature compression module based on the
channel attention mechanism in CNNs, to compress intermediate data by selecting
the most informative features. In the semantic decoder, we design a lightweight
decoder to reconstruct the intermediate data through learning from the received
compressed data to improve accuracy. To effectively trade-off communication,
computation, and inference accuracy, we design a reward function and formulate
the offloading problem of CNN inference as a maximization problem with the goal
of maximizing the average inference accuracy and throughput over the long term.
To address this maximization problem, we propose a graph reinforcement
learning-based AECNN (GRL-AECNN) method, which outperforms existing works
DROO-AECNN, GRL-BottleNet++ and GRL-DeepJSCC under different dynamic scenarios.
This highlights the advantages of GRL-AECNN in offloading decision-making in
dynamic MEC.
更多查看译文
AI 理解论文
溯源树
样例
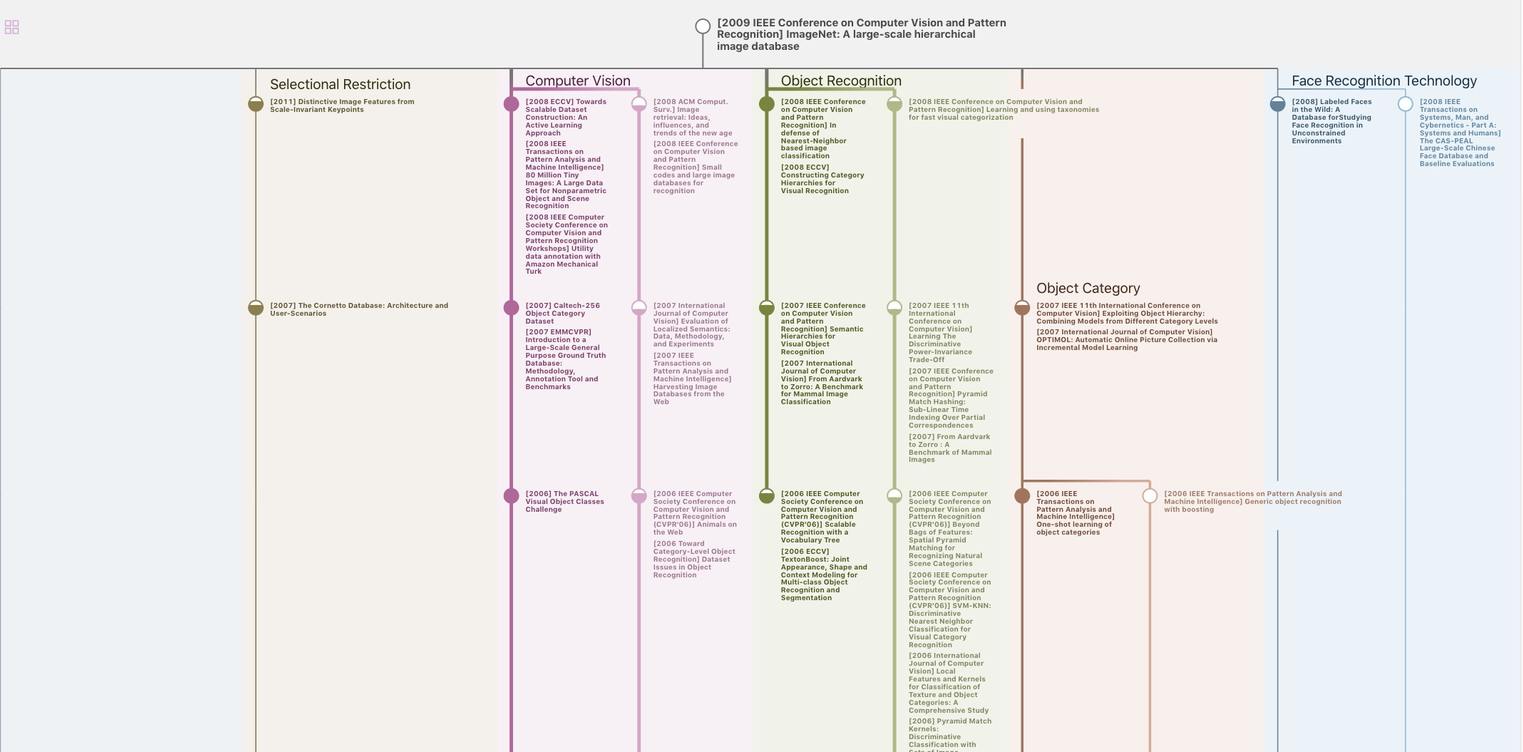
生成溯源树,研究论文发展脉络
Chat Paper
正在生成论文摘要