Dconformer: A denoising convolutional transformer with joint learning strategy for intelligent diagnosis of bearing faults
MECHANICAL SYSTEMS AND SIGNAL PROCESSING(2024)
摘要
Rolling bearings are the core components of rotating machinery, and their normal operation is crucial to entire industrial applications. Most existing condition monitoring methods have been devoted to extracting discriminative features from vibration signals that reflect bearing health status. However, the complex working conditions of rolling bearings often make the faultrelated information easily buried in noise and other interference. Therefore, it is challenging for existing approaches to extract sufficient critical features in these scenarios. To address this issue, this paper proposes a novel CNN -Transformer network, referred to as Dconformer, capable of extracting both local and global discriminative features from noisy vibration signals. The main contributions of this research include: (1) Developing a novel joint -learning strategy that simultaneously enhances the performance of signal denoising and fault diagnosis, leading to robust and accurate diagnostic results; (2) Constructing a novel CNN -transformer network with a multi -branch cross -cascaded architecture, which inherits the strengths of CNNs and transformers and demonstrates superior anti -interference capability. Extensive experimental results reveal that the proposed Dconformer outperforms five state-of-the-art approaches, particularly in strong noisy scenarios.
更多查看译文
关键词
Rolling bearing,Fault diagnosis,Vibration signal,Dconformer,Complex working conditions,Noisy scenarios
AI 理解论文
溯源树
样例
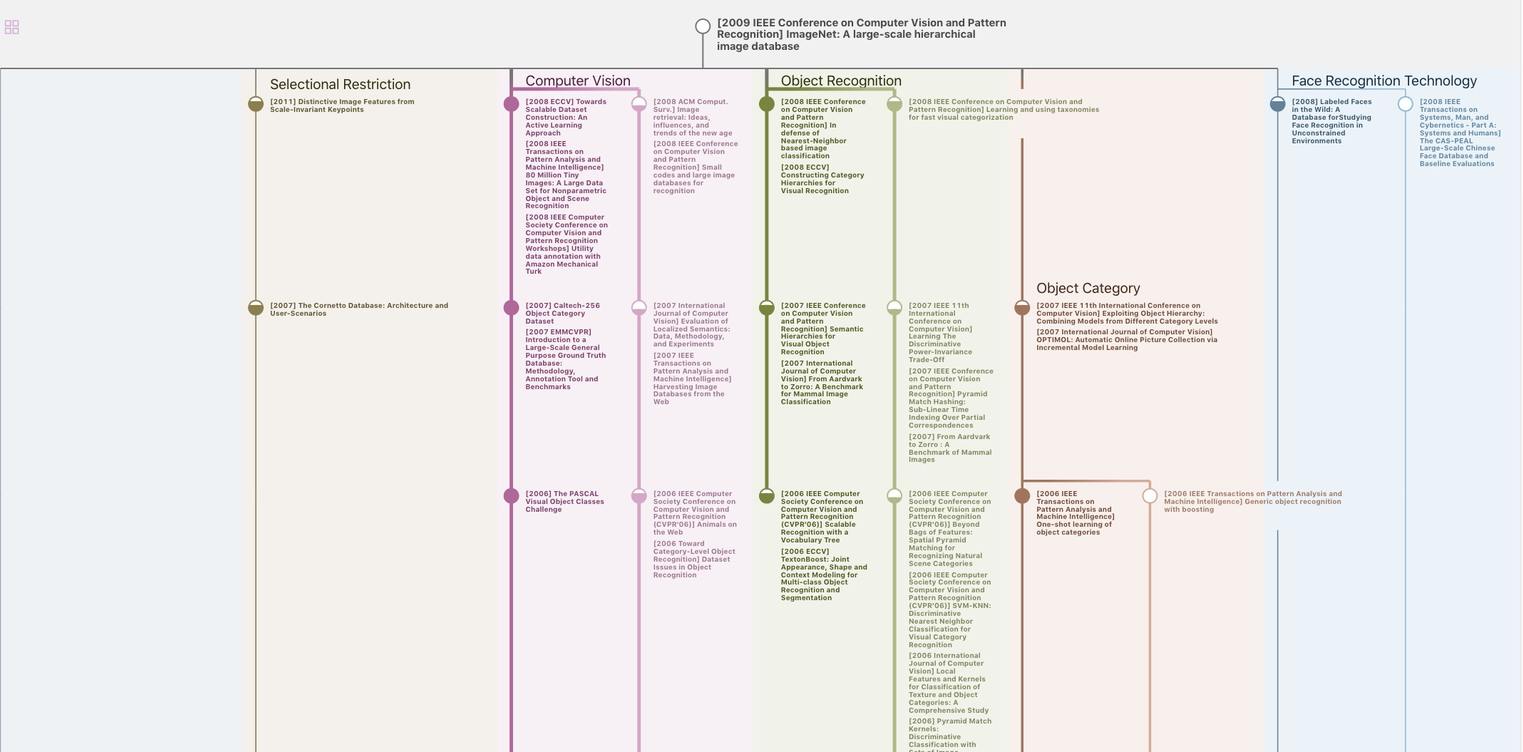
生成溯源树,研究论文发展脉络
Chat Paper
正在生成论文摘要