Transfer Learning in Multiple Hypothesis Testing
ENTROPY(2024)
摘要
In this investigation, a synthesis of Convolutional Neural Networks (CNNs) and Bayesian inference is presented, leading to a novel approach to the problem of Multiple Hypothesis Testing (MHT). Diverging from traditional paradigms, this study introduces a sequence-based uncalibrated Bayes factor approach to test many hypotheses using the same family of sampling parametric models. A two-step methodology is employed: initially, a learning phase is conducted utilizing simulated datasets encompassing a wide spectrum of null and alternative hypotheses, followed by a transfer phase applying this fitted model to real-world experimental sequences. The outcome is a CNN model capable of navigating the complex domain of MHT with improved precision over traditional methods, also demonstrating robustness under varying conditions, including the number of true nulls and dependencies between tests. Although indications of empirical evaluations are presented and show that the methodology will prove useful, more work is required to provide a full evaluation from a theoretical perspective. The potential of this innovative approach is further illustrated within the critical domain of genomics. Although formal proof of the consistency of the model remains elusive due to the inherent complexity of the algorithms, this paper also provides some theoretical insights and advocates for continued exploration of this methodology.
更多查看译文
关键词
bayes factors,deep learning,improper priors,objective bayesian inference,random sequences,RNA-seq experiments
AI 理解论文
溯源树
样例
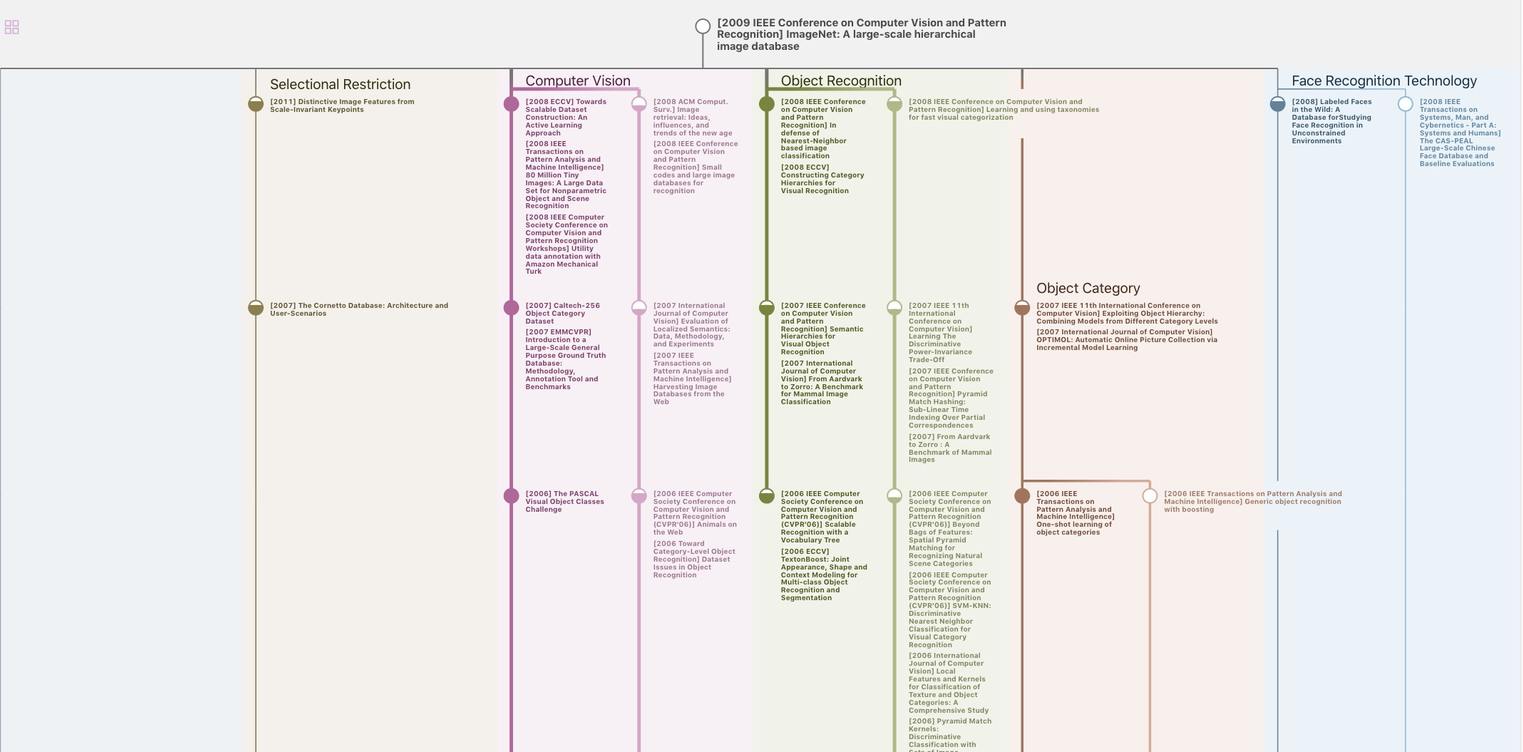
生成溯源树,研究论文发展脉络
Chat Paper
正在生成论文摘要