Curriculum Consistency Learning and Multi-Scale Contrastive Constraint in Semi-Supervised Medical Image Segmentation
BIOENGINEERING-BASEL(2024)
摘要
Data scarcity poses a significant challenge in medical image segmentation, thereby highlighting the importance of leveraging sparse annotation data. In addressing this issue, semi-supervised learning has emerged as an effective approach for training neural networks using limited labeled data. In this study, we introduced a curriculum consistency constraint within the context of semi-supervised medical image segmentation, thus drawing inspiration from the human learning process. By dynamically comparing patch features with full image features, we enhanced the network's ability to learn. Unlike existing methods, our approach adapted the patch size to simulate the human curriculum process, thereby progressing from easy to hard tasks. This adjustment guided the model toward improved convergence optima and generalization. Furthermore, we employed multi-scale contrast learning to enhance the representation of features. Our method capitalizes on the features extracted from multiple layers to explore additional semantic information and point-wise representations. To evaluate the effectiveness of our proposed approach, we conducted experiments on the Kvasir-SEG polyp dataset and the ISIC 2018 skin lesion dataset. The experimental results demonstrated that our method surpassed state-of-the-art semi-supervised methods by achieving a 9.2% increase in the mean intersection over union (mIoU) for the Kvasir-SEG dataset. This improvement substantiated the efficacy of our proposed curriculum consistency constraint and multi-scale contrastive loss.
更多查看译文
关键词
medical image segmentation,semi-supervised learning,curriculum learning,consistency loss,contrastive learning
AI 理解论文
溯源树
样例
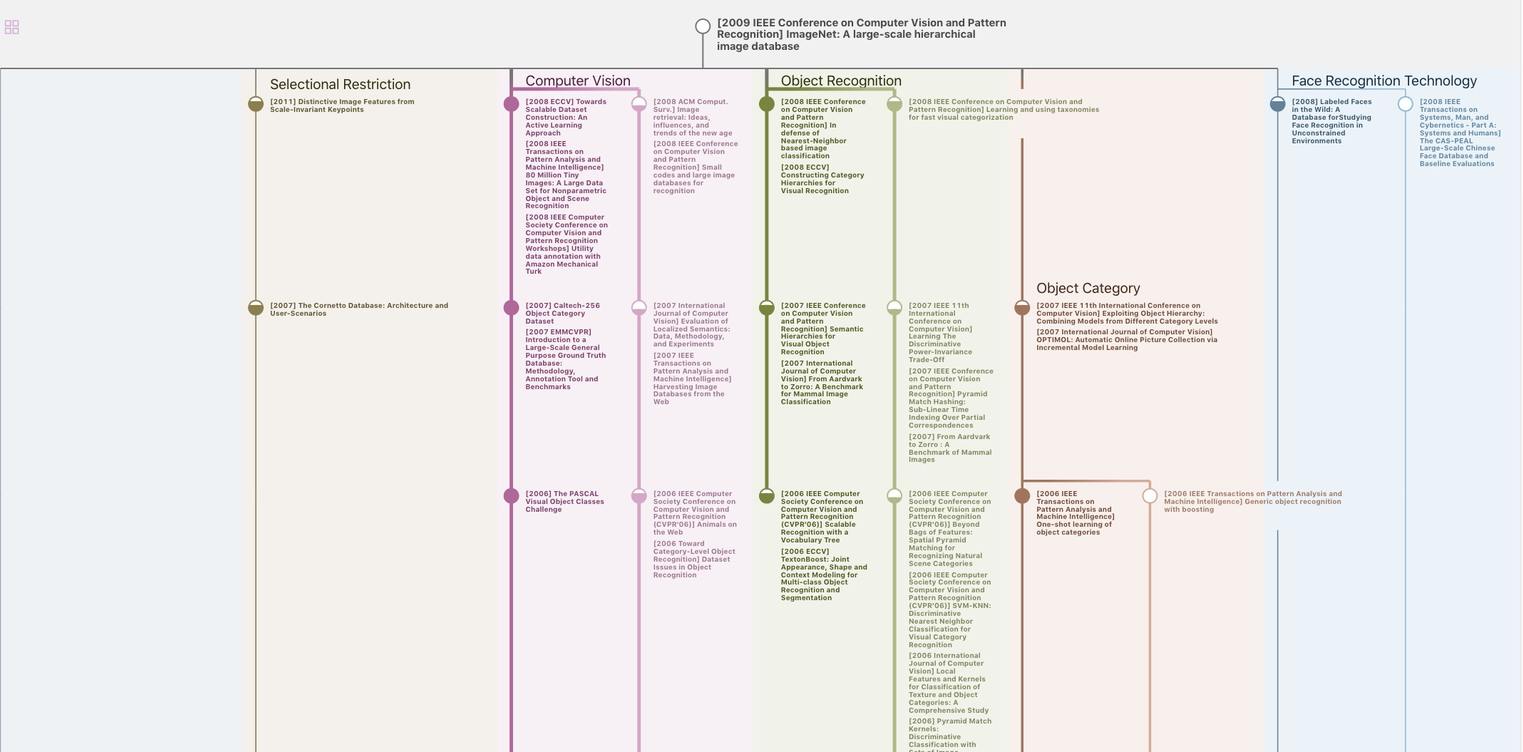
生成溯源树,研究论文发展脉络
Chat Paper
正在生成论文摘要