Exploring low-resource medical image classification with weakly supervised prompt learning
PATTERN RECOGNITION(2024)
摘要
Most advances in medical image recognition supporting clinical auxiliary diagnosis meet challenges due to the low-resource situation in the medical field, where annotations are highly expensive and professional. This low-resource problem can be alleviated by leveraging the transferable representations of large-scale pre-trained vision-language models like CLIP. After being pre-trained using large-scale unlabeled medical images and texts (such as medical reports), the vision-language models can learn transferable representations and support flexible downstream clinical tasks such as medical image classification via relevant medical text prompts. However, existing pre-trained vision-language models require domain experts (clinicians) to carefully design the medical text prompts based on different datasets when applied to specific medical image tasks, which is extremely time-consuming and greatly increases the burden on clinicians. To address this problem, we propose a weakly supervised prompt learning method MedP rompt for automatically generating medical prompts, which includes an unsupervised pre-trained vision-language model and a weakly supervised prompt learning model. The unsupervised pre-trained vision-language model adopts large-scale medical images and texts for pretraining, utilizing the natural correlation between medical images and corresponding medical texts without manual annotations. The weakly supervised prompt learning model only utilizes the classes of images in the dataset to guide the learning of the specific class vector in the prompt, while the learning of other context vectors in the prompt does not require any manual annotations for guidance. To the best of our knowledge, this is the first model to automatically generate medical prompts. With the assistance of these prompts, the pre-trained vision-language model can be freed from the strong expert dependency of manual annotation and manual prompt design, thus achieving end -to -end, low-cost medical image classification. Experimental results show that the model using our automatically generated prompts outperforms all its hand-crafted prompts counterparts in full-shot learning on all four datasets, and achieves superior accuracy on zero-shot image classification and few-shot learning in three of the four medical benchmark datasets and comparable accuracy in the remaining one. In addition, the proposed prompt generator is lightweight and therefore has the potential to be embedded into any network architecture.
更多查看译文
关键词
Medical image classification,Weakly supervised learning,Prompt learning,Few-shot learning,Zero-shot learning
AI 理解论文
溯源树
样例
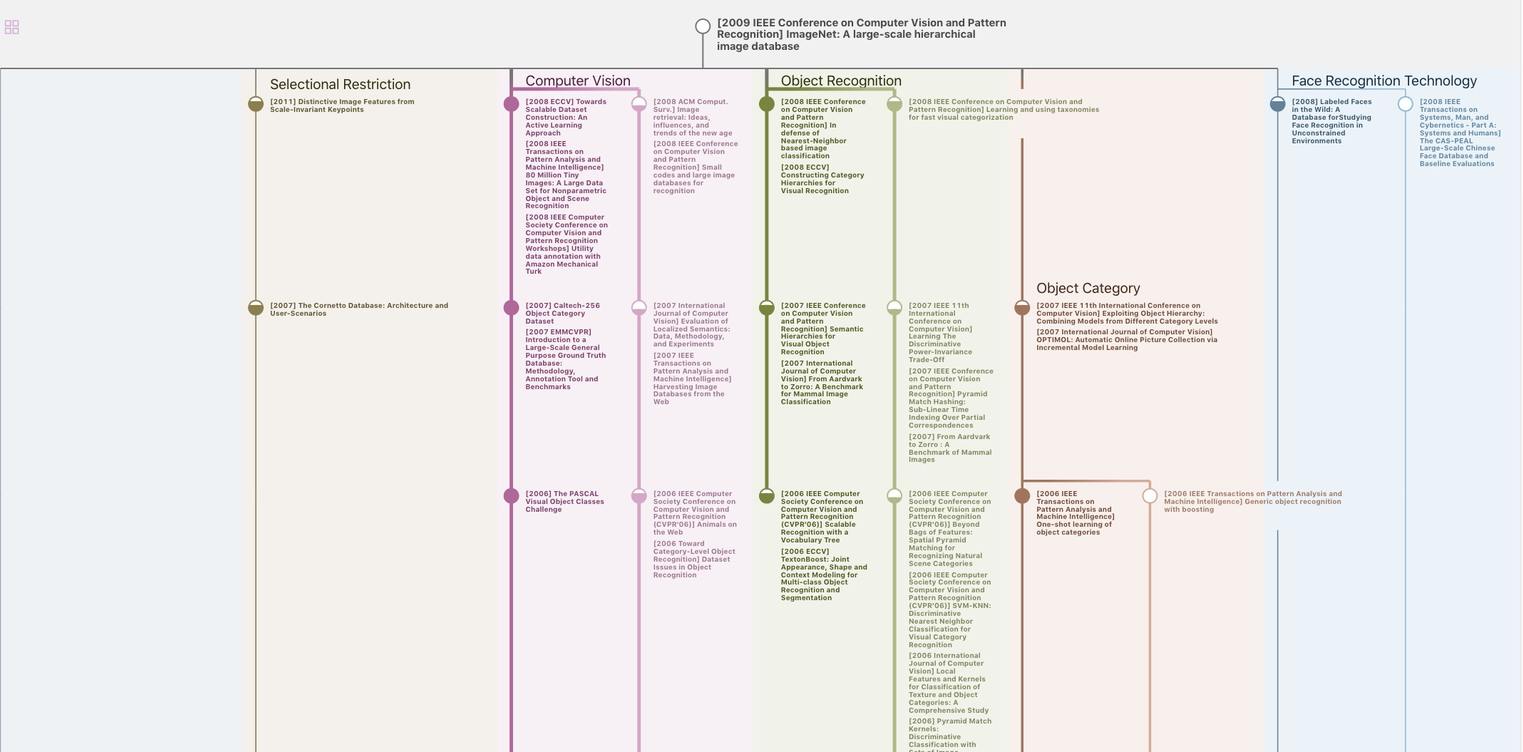
生成溯源树,研究论文发展脉络
Chat Paper
正在生成论文摘要