Model-free Reinforcement Learning for Spatiotemporal Tasks using Symbolic Automata
2023 62ND IEEE CONFERENCE ON DECISION AND CONTROL, CDC(2023)
摘要
Reinforcement learning (RL) is a popular paradigm for synthesizing controllers in environments modeled as Markov Decision Processes (MDPs). The RL formulation assumes that users define local rewards that depend only on the current state (and action), and learning algorithms seek to find control policies that maximize cumulative rewards along system trajectories. An implicit assumption in RL is that policies that maximize cumulative rewards are desirable as they meet the intended control objectives. However, most control objectives are global properties of system trajectories, and meeting them with local rewards requires tedious, manual and error-prone process of hand-crafting the rewards. We propose a new algorithm for automatically inferring local rewards from high-level task objectives expressed in the form of symbolic automata (SA); a symbolic automaton is a finite state machine where edges are labeled with symbolic predicates over the MDP states. SA subsume many popular formalisms for expressing task objectives, such as discrete-time versions of Signal Temporal Logic (STL). We assume that a model-free RL setting, i.e., we assume no prior knowledge of the system dynamics. We give theoretical results that establish that an optimal policy learned using our shaped rewards also maximizes the probability of satisfying the given SA-based control objective. We empirically compare our approach with other RL methods that try to learn policies enforcing temporal logic and automata-based control objective. We demonstrate that our approach outperforms these methods both in terms of the number of iterations required for convergence and the probability that the learned policy satisfies the SA-based objectives.
更多查看译文
AI 理解论文
溯源树
样例
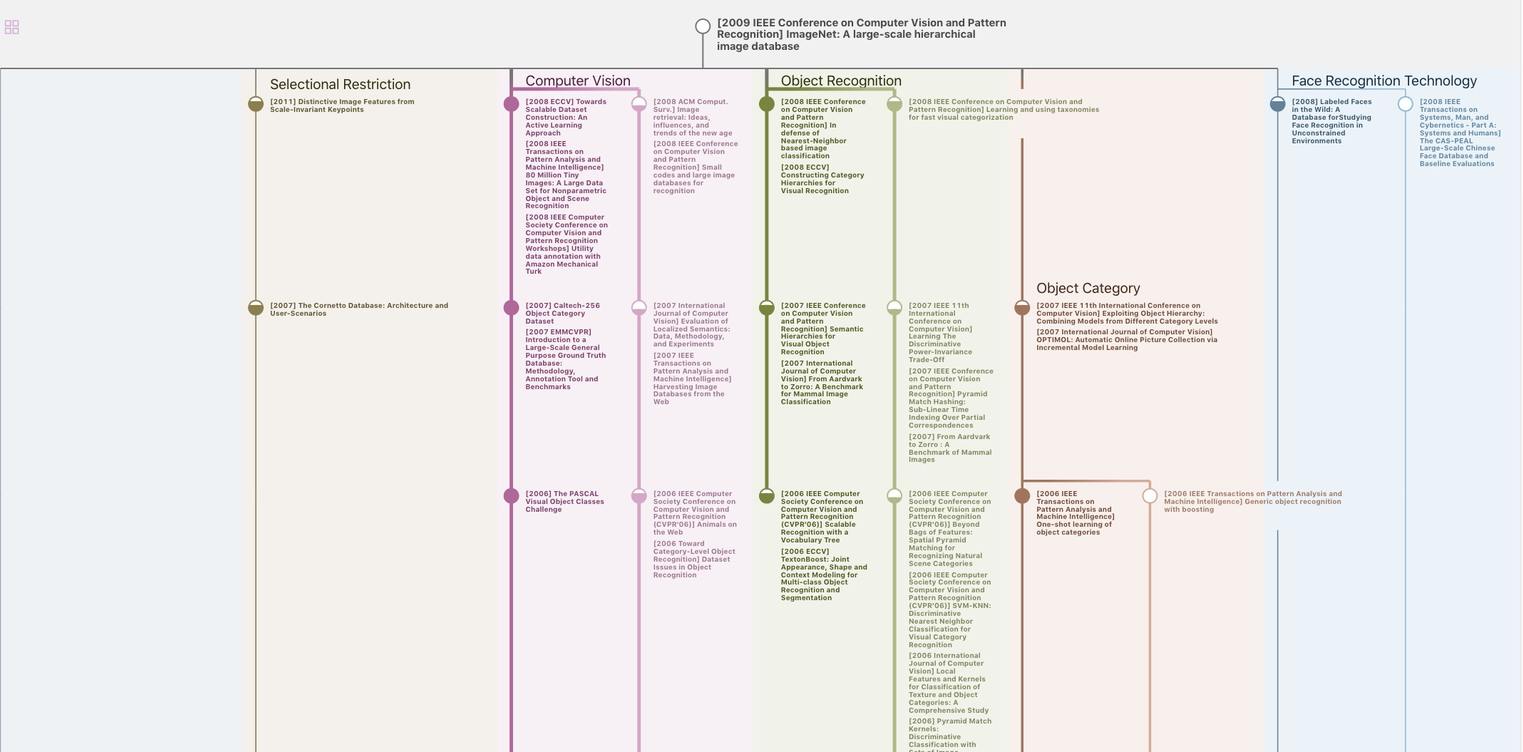
生成溯源树,研究论文发展脉络
Chat Paper
正在生成论文摘要