Predicting Microbe-Metabolite Interactions by Integrating Non-negative Matrix Factorization and Generative Network.
2023 IEEE International Conference on Bioinformatics and Biomedicine (BIBM)(2023)
摘要
Despite profound impacts on human health and nature, accurately predicting microbe-metabolite interactions remains challenging due to inherent data noise. This study applies non-negative matrix factorization (NMF) and multi-view NMF to reduce noise and exploit associations across data perspectives. NMF obtains low-dimensional microbial and metabolic representations, effectively reducing noise. The dimension-reduced spectral matrices were input into the generative network model to derive conditional probabilities of individual microbe-associated metabolites and microbe-metabolite co-occurrence probabilities, the latter enabling prediction of microbe-metabolite interactions. Moreover, multi-view NMF integrates microbial and metabolic data by mapping them into a shared subspace, thereby enhancing prediction performance and validating cross-perspective correlation modeling. This study demonstrates NMF's efficacy in noise reduction through dimensionality reduction, and multiview NMF's ability to leverage cross-view associations. Both approaches demonstrate enhanced microbe-metabolite interaction prediction utilizing NMF-based and multi-view NMF-based generative network models.
更多查看译文
关键词
Non-negative matrix factorization,Microbe-metabolite interactions,Multi-view,Microbiome,Metabolome
AI 理解论文
溯源树
样例
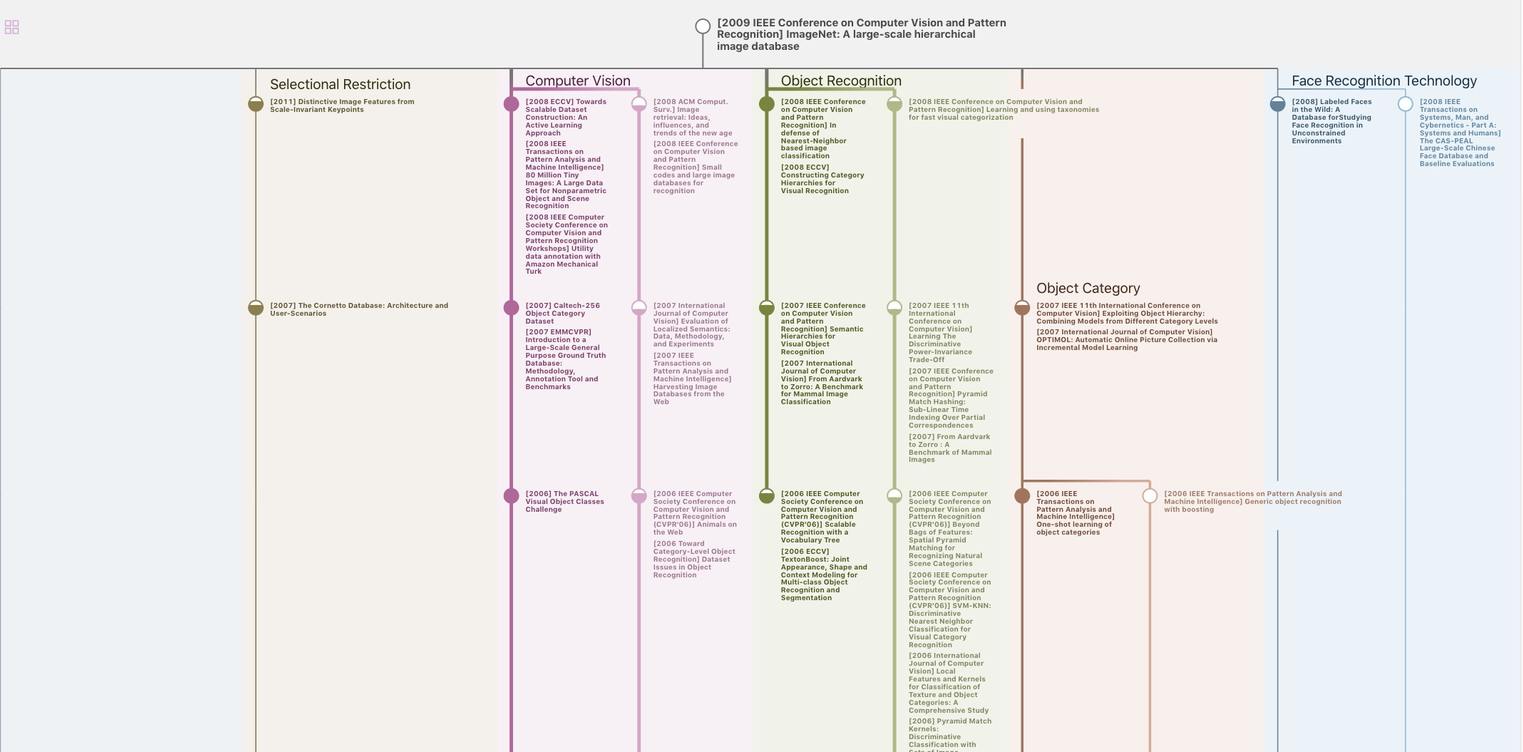
生成溯源树,研究论文发展脉络
Chat Paper
正在生成论文摘要