Incipient fault detection with feature ensemble based on one-class machine learning methods
2023 62ND IEEE CONFERENCE ON DECISION AND CONTROL, CDC(2023)
摘要
Considering production quality and process safety, incipient fault detection has drawn more and more attention. With the rapid development of machine learning, numerous researches for fault detection based on machine learning have been published. However, almost all machine learning methods used for fault detection need abnormal data to construct models. Unfortunately, it is difficult to obtain sufficient fault samples in practical industrial processes. In addition, the existing fault detection methods are based on single feature extraction strategy. Process monitoring methods with different working principles often extract and utilize different process information. Reasonable integration of features extracted by multiple methods can usually effectively improve the performance of incipient fault detection. Therefore, this paper proposes an one-class machine learning feature ensemble model (OCMLFEM) for incipient fault detection. In OCMLFEM, various one-class machine learning models are constructed as basic detectors. In order to effectively mine the features obtained by basic detectors, a feature ensemble strategy with the technologies of sliding window singular value and principal component analysis is adopted. Then, Tennessee Eastman process is utilized to verify the validity of the proposed detection model, which proves that OCMLFEM has significant superiority.
更多查看译文
关键词
fault detection,ensemble strategy,machine learning
AI 理解论文
溯源树
样例
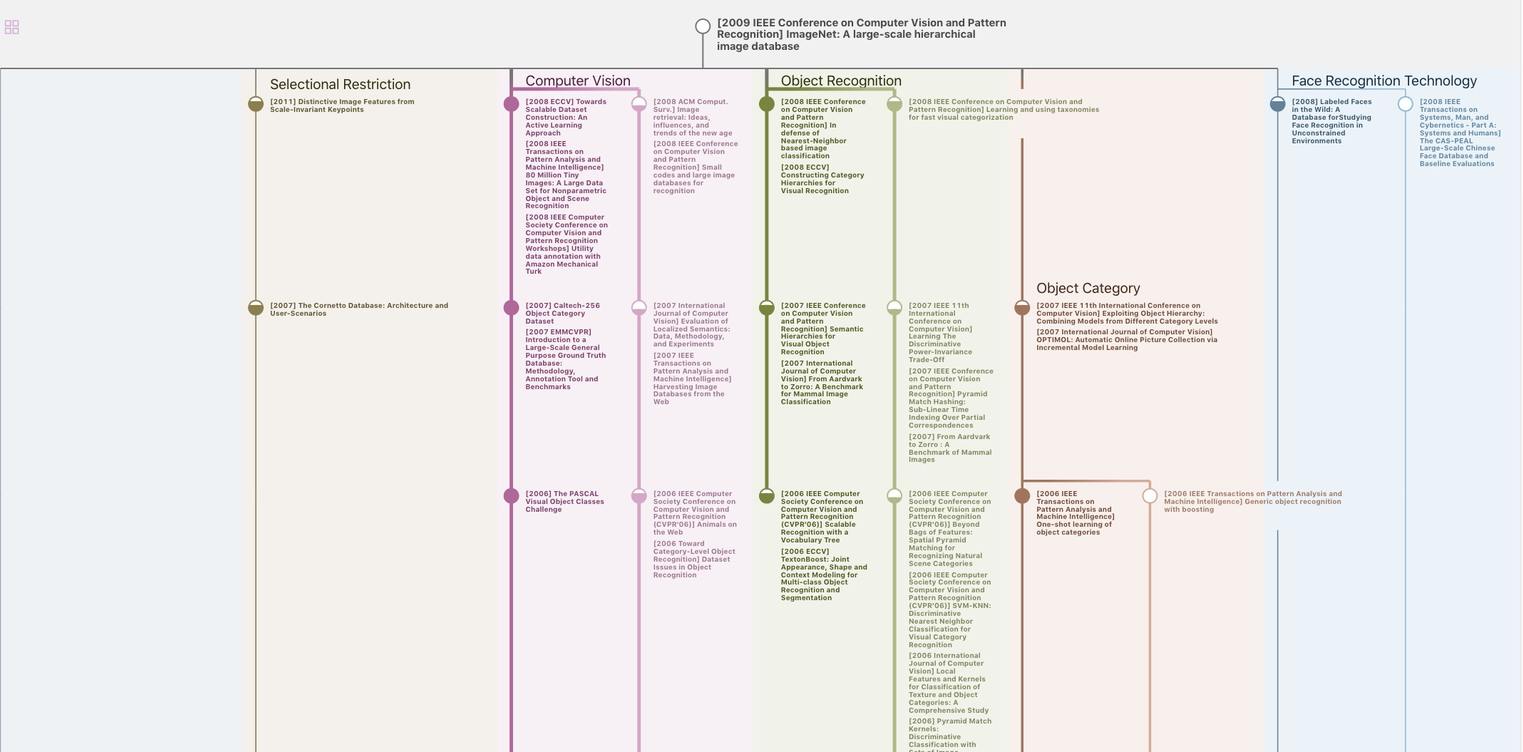
生成溯源树,研究论文发展脉络
Chat Paper
正在生成论文摘要