Data-Driven Reachability Analysis for Gaussian Process State Space Models
2023 62ND IEEE CONFERENCE ON DECISION AND CONTROL, CDC(2023)
摘要
Gaussian process state space models are becoming common tools for the analysis and design of nonlinear systems with uncertain dynamics. When designing control policies for these systems, safety is an important property to consider. In this paper, we provide safety guarantees by computing finite-horizon forward reachable sets for Gaussian process state space models. We use data-driven reachability analysis to provide exact probability measures for state trajectories of arbitrary length, even when no data samples are available. We investigate two numerical examples to demonstrate the power of this approach, such as providing highly non-convex reachable sets and detecting holes in the reachable set.
更多查看译文
AI 理解论文
溯源树
样例
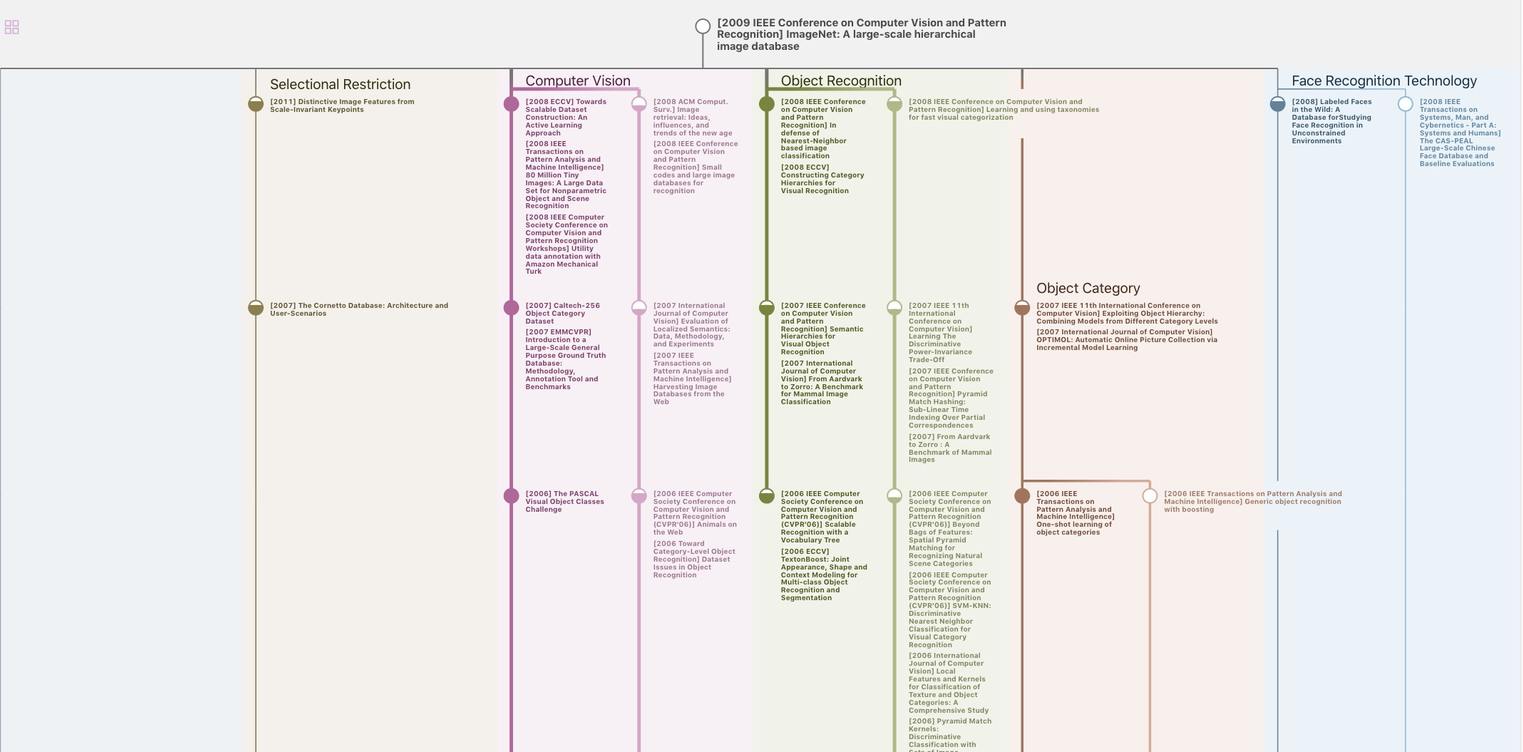
生成溯源树,研究论文发展脉络
Chat Paper
正在生成论文摘要