The Power Function for Adaptive Control in Native Space Embedding
2023 62ND IEEE CONFERENCE ON DECISION AND CONTROL, CDC(2023)
摘要
This paper studies how the power function in a reproducing kernel Hilbert space (RKHS) can be used systematically to design error bounding methods of adaptive estimation and control via the native space embedding method. The approach is based on viewing the original system of ordinary differential equations (ODEs) as a type of distributed parameter system (DPS), and subsequently defining realizable controllers by approximating the DPS with scattered bases over a domain of interest. The approach provides rigorous bounds on ultimate performance guarantees for uncertainty classes defined in the native space. One result derives an upper bound on the ultimate performance of the adaptive controller in terms of the power function. Another version of this upper bound shows how the ultimate performance can bounded in terms of a fill distance of centers of approximation in subsets that contain the closed loop trajectory. In contrast to the general theory for error bounding adaptive controllers in Euclidean space, the general approach in this paper works for functional uncertainties in any RKHS.
更多查看译文
关键词
Native space,Distributed parameter system,Adaptive control
AI 理解论文
溯源树
样例
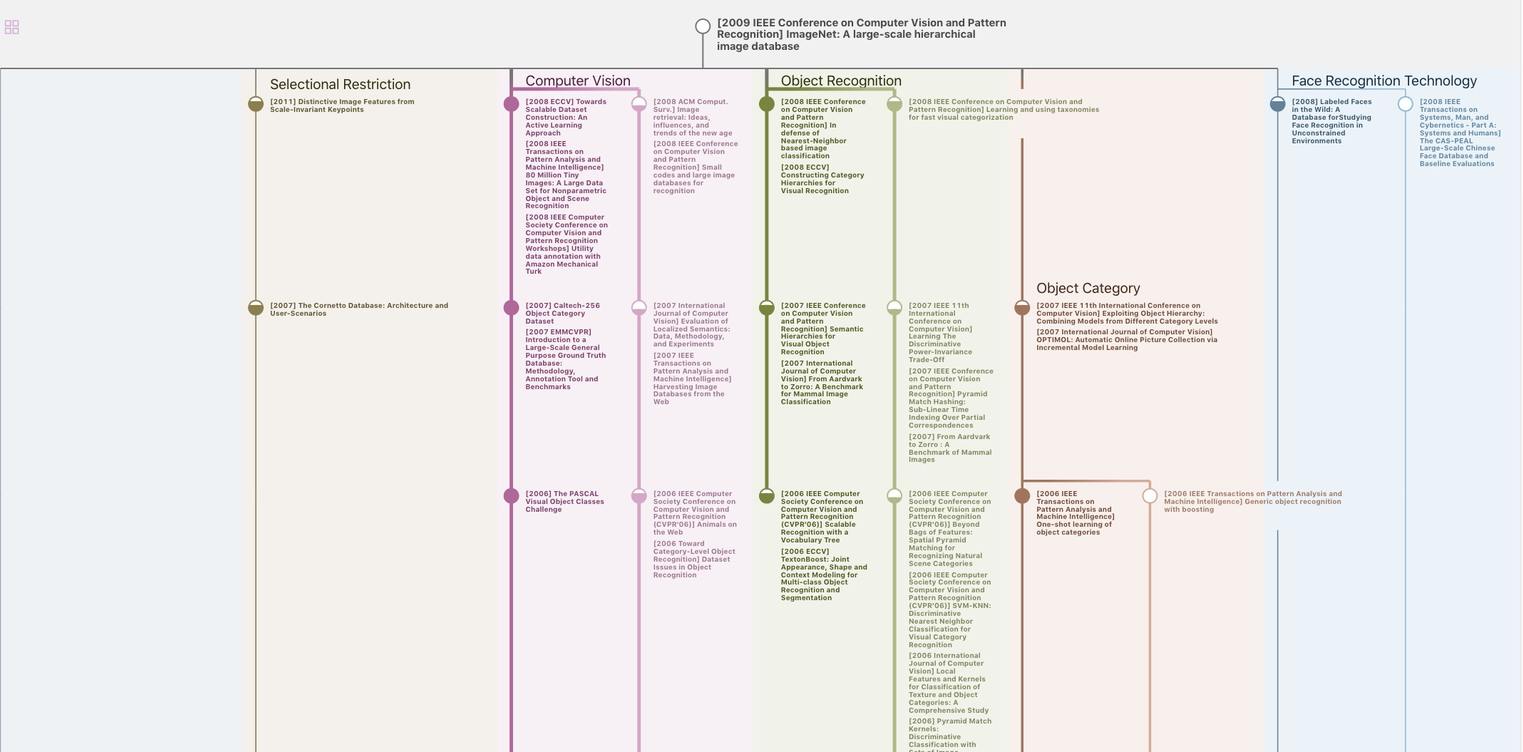
生成溯源树,研究论文发展脉络
Chat Paper
正在生成论文摘要