Adaptive Low-Rank Gradient Descent
2023 62ND IEEE CONFERENCE ON DECISION AND CONTROL, CDC(2023)
摘要
Low-rank structures have been observed in several recent empirical studies in many machine and deep learning problems, where the loss function demonstrates significant variation only in a lower dimensional subspace. While traditional gradient-based optimization algorithms are computationally costly for high-dimensional parameter spaces, such low-rank structures provide an opportunity to mitigate this cost. In this paper, we aim to leverage low-rank structures to alleviate the computational cost of first-order methods and study Adaptive Low-Rank Gradient Descent (AdaLRGD). The main idea of this method is to begin the optimization procedure in a very small subspace and gradually and adaptively augment it by including more directions. We show that for smooth and strongly convex objectives and any target accuracy., AdaLRGD's complexity is O(r ln(r/epsilon)) for some rank r no more than dimension d. This significantly improves upon gradient descent's complexity of O(d ln(1/epsilon)) when r << d. We also propose a practical implementation of AdaLRGD and demonstrate its ability to leverage existing low-rank structures in data.
更多查看译文
AI 理解论文
溯源树
样例
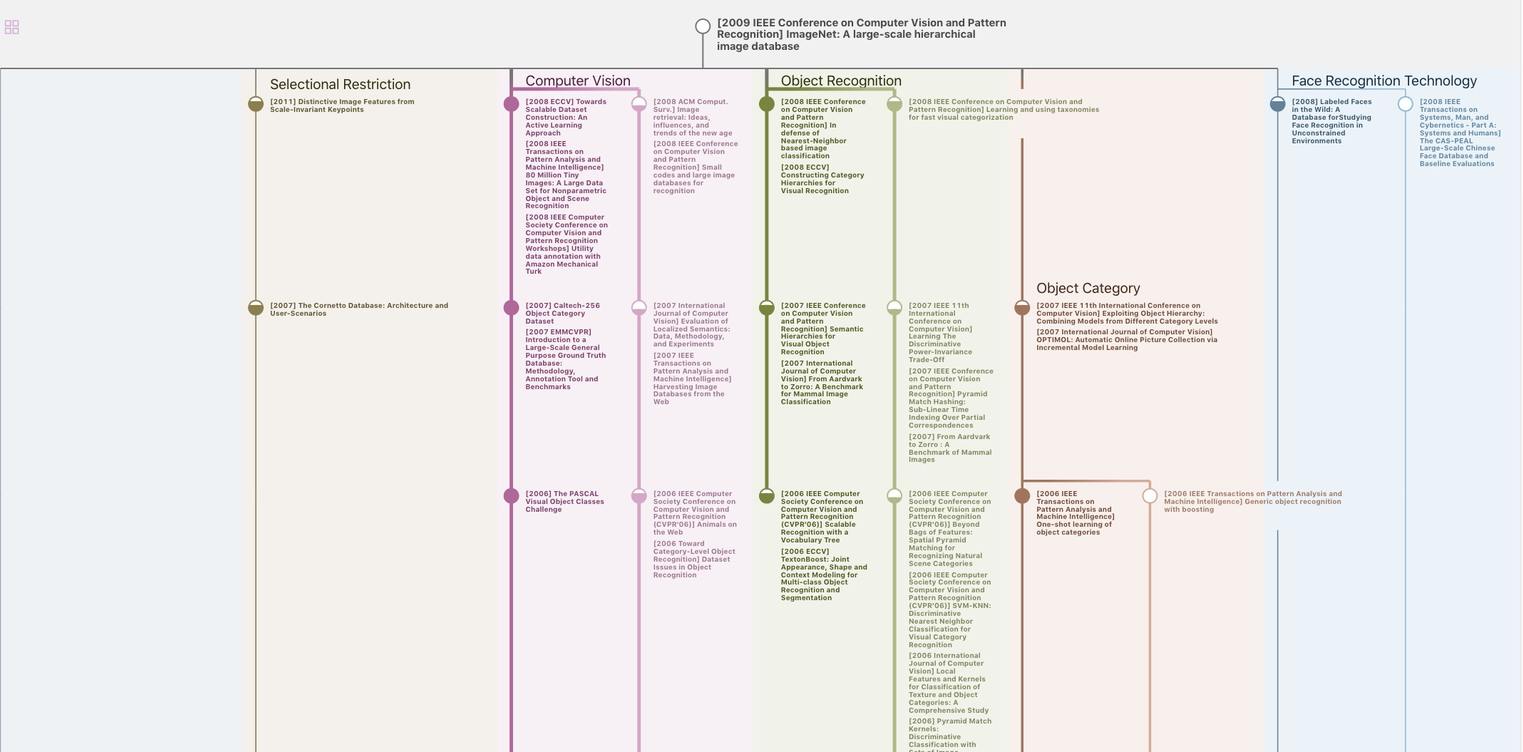
生成溯源树,研究论文发展脉络
Chat Paper
正在生成论文摘要