Interpretable Diagnosis of Face Acne via Complementation Learning of Evidence Localization and Severity Level Grading.
2023 IEEE International Conference on Bioinformatics and Biomedicine (BIBM)(2023)
摘要
Acne seriously affects people’s daily lives. Several studies of automated acne diagnosis either lack reasonable interpretation to support the diagnosis or ignore evidence in the diagnosis process. In this paper, we propose an interpretable diagnosis framework for face acne. This framework uses complementation learning of evidence localization and severity level grading to boost both streams by sharing the features that support each other. Evidence localization learns to identify lesion areas for supporting the diagnosis stream as well as providing interpretation. Severity level grading learns to recognize the diagnosis result and also provides reference and rectification for evidence localization. Experimental results show that complementation learning improves both evidence localization and severity level grading, the lesion areas from evidence localization can support the diagnosis and provide interpretations, and the diagnosis framework reaches the state-of-the-art level and the diagnostic performance of dermatologists.
更多查看译文
关键词
acne severity grading,lesion localization,interpretable diagnosis,deep learning
AI 理解论文
溯源树
样例
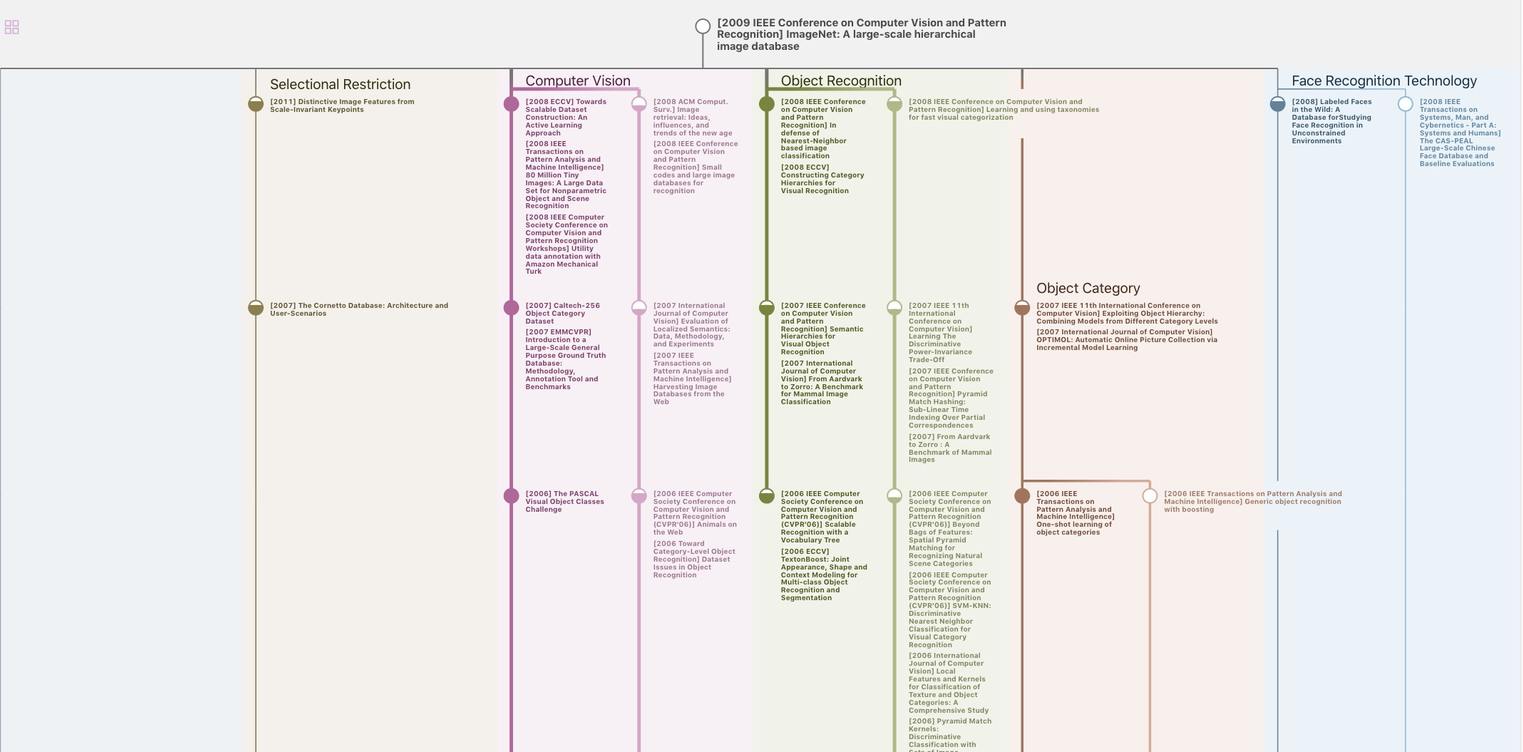
生成溯源树,研究论文发展脉络
Chat Paper
正在生成论文摘要