A Study on Reducing Big Data Image Annotation Burden Through Iterative Expert-In-The-Loop Strategy.
2023 IEEE International Conference on Bioinformatics and Biomedicine (BIBM)(2023)
摘要
A key challenge in development of reliable and robust medical imaging machine learning solution is the lack of annotated data. This problem becomes particularly significant when big data sets are used. These pose a burden on the annotators to manually segment regions of interest which is a labor intensive and tedious approach. One solution toward addressing this challenge is to use an iterative expert-in-the-loop approach where models that are initially, albeit weakly, trained on a small expert segmented data set are progressively used to expand the training data. In this work, we explore the viability of this approach through two segmentation experiments. The first is a challenging problem of segmenting the buccal mucosa region from photographs of the mouth for subsequent detection and classification of lesions aimed at an oral cancer prediction application. The other is to segment the lung region in chest X-ray (CXR) images. For simplicity and to focus on discovering viability and any associated shortcomings, we limited our scope to just using an off-the-shelf U-Net algorithm to determine if this approach to training data expansion improved segmentation results. Our findings show that for the buccal mucosa segmentation in oral photographs, the method achieved up to 10% improvement in Dice Similarity Coefficient over three iterations on a blinded manually segmented hold-out test set before the performance plateaued. However, the training data set size almost doubled in size in two iterations. For CXR lung segmentation, we observe slight performance improvement (1% in one iteration) of the method from its initial model which already has a much higher performance (93%). We analyze the performance of the approach for these data sets and comment on the potential of a human expert-in-the-loop method for training data expansion for unlabeled or weakly-labeled medical imaging data.
更多查看译文
关键词
Iterative learning,Image Segmentation,Weakly labeled augmentation,Human-in-the-loop,Oral Cancer
AI 理解论文
溯源树
样例
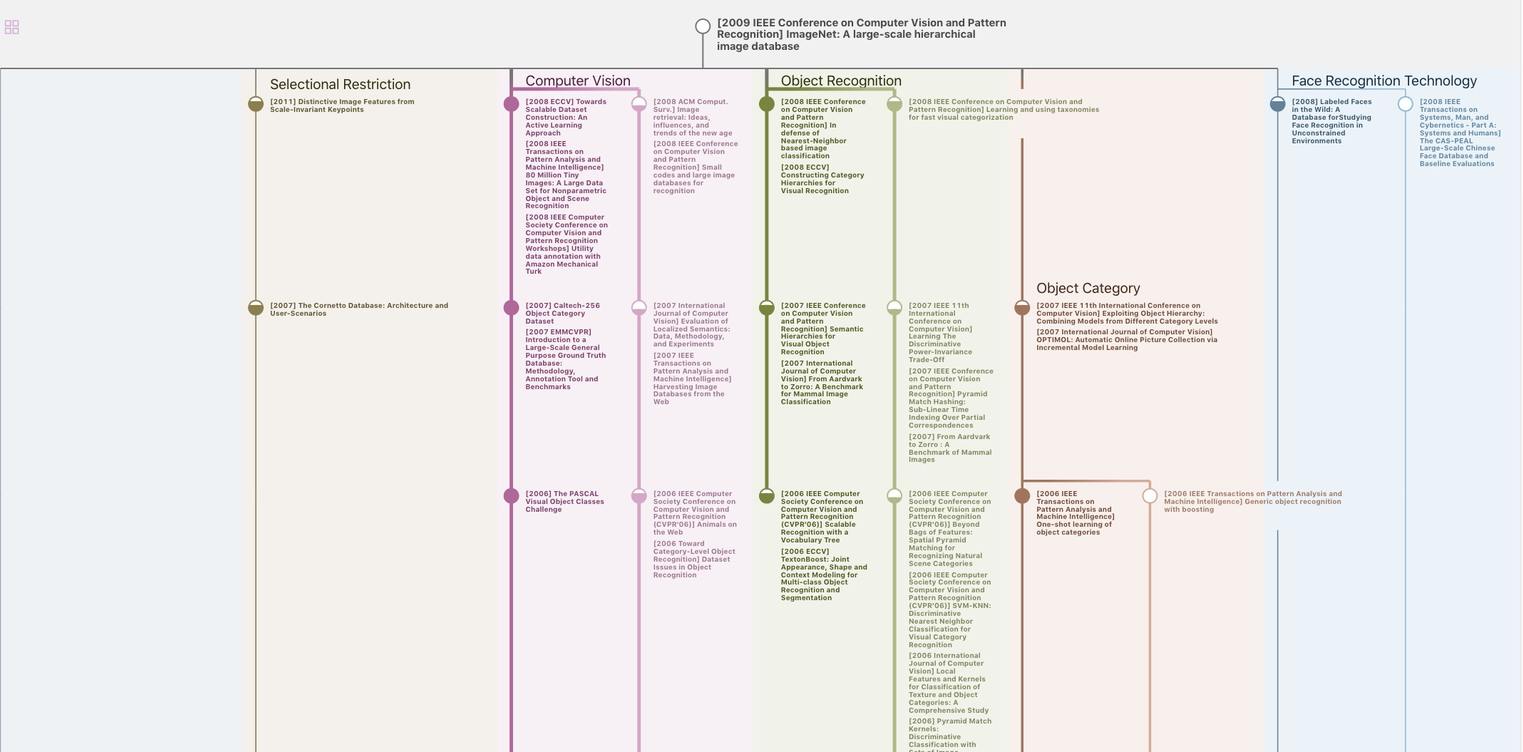
生成溯源树,研究论文发展脉络
Chat Paper
正在生成论文摘要