An End-to-End Multi-stage Network for Ultrasound Video Object Segmentation.
2023 IEEE International Conference on Bioinformatics and Biomedicine (BIBM)(2023)
摘要
Real-time tracking and segmentation of ultrasound video sequence are prerequisite for identifying and analyzing lesions. While significant progress has been made in natural video object segmentation, developing a model for ultrasound video is still challenging due to problems such as low distinguishability and low visual saliency of the target objects, large variation between adjacent frames. These challenges are inherently complex and cannot be effectively tackled through a single process. This paper develops an end-to-end multi-stage network (EMNet) for ultrasound video object segmentation. EMNet consists of two stages. The inital mask generation stage comprises a contrast-enhanced layer to enhance visual contrast between targets and backgrounds. In this stage, a module that adopts the encoder-attention-decoder structure is designed for mask induction. After obtaining the initial segmentation mask, the mask refinement stage is followed to further improve initial segmentation. To prevent the propagation of errors, a gating mechanism is designed to control the fusion of segmentation probability maps in the initial and refinement stages. By transforming certain fixed parameters in different stage into trainable parameters and establishing an end-to-end learning process, we optimized the performance of our approach. We evaluate EMNet on real-world lymphoma ultrasound video dataset. Compared with the best results among seven competing baselines, EMNet achieves the best performance in terms of ℐ&ℱ and ℱ measures, the second-best performance with Param and FPS measures, which demonstrates the competitive performance in terms of both speed and accuracy.
更多查看译文
关键词
ultrasound video segmentation,contrast enhancement,end-to-end,gating mechanism
AI 理解论文
溯源树
样例
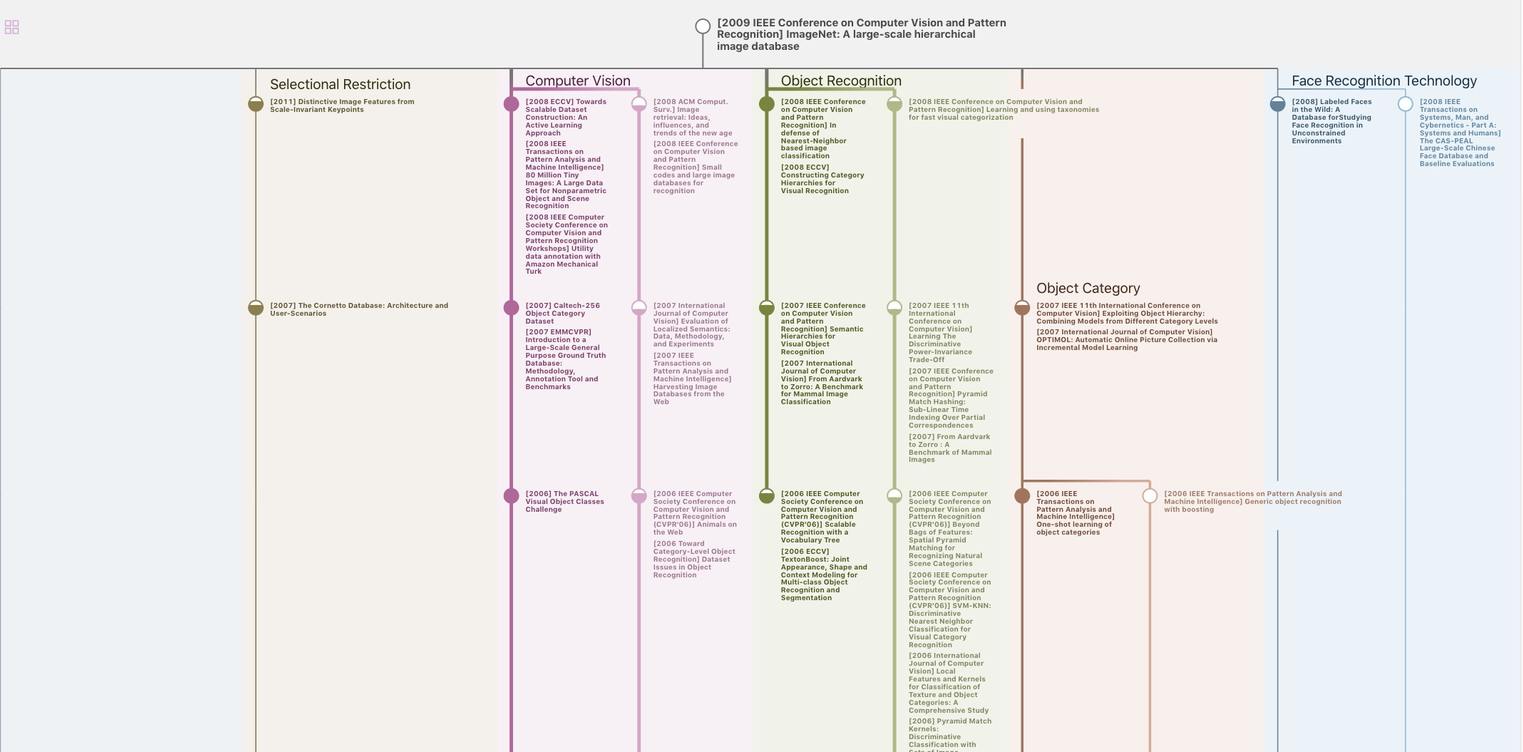
生成溯源树,研究论文发展脉络
Chat Paper
正在生成论文摘要