Effective Drug Repositioning with a Novel Negative Sample Selection Algorithm.
2023 IEEE International Conference on Bioinformatics and Biomedicine (BIBM)(2023)
摘要
Drug repositioning is the process of identifying potential associations between approved drugs and diseases (DDAs) to unveil novel therapeutic applications. Unlike traditional drug discovery approaches, a key advantage of drug repositioning lies in its capacity to leverage the existing knowledge and safety profiles of established medications, leading to significant reductions in both the time and costs associated with drug development. While various methods have been proposed to address this challenge using diverse strategies, the conventional approach for training DDAs prediction models typically relies on random sampling of unknown drug-disease pairs to construct negative samples. However, this method may inadvertently introduce unwanted noise or errors by erroneously categorizing some genuine DDAs as negative samples, thereby leaving room for improvement in current methodologies. In this paper, we introduce a novel negative sample selection algorithm for DDAs prediction that explicitly incorporates causal knowledge inherent in DDAs. To accomplish this, we first construct a heterogeneous information network (HIN) that encompasses various biological entities associated with DDAs and their interconnections. Subsequently, we utilize the outcomes of community detection within the HIN as a form of counterfactual inference, resulting in the development of a negative sample selection algorithm based on a thoughtfully designed counterfactual question. By combining the known DDAs (i.e., positive samples) with the newly generated negative samples, we train a prediction model that incorporates a graph learning module to acquire representations of drugs and diseases. Comprehensive experiments confirm the effectiveness of our proposed model for DDAs prediction.
更多查看译文
关键词
drug repositioning,drug-disease associations prediction,counterfactual links,graph convolutional network,heterogeneous information network
AI 理解论文
溯源树
样例
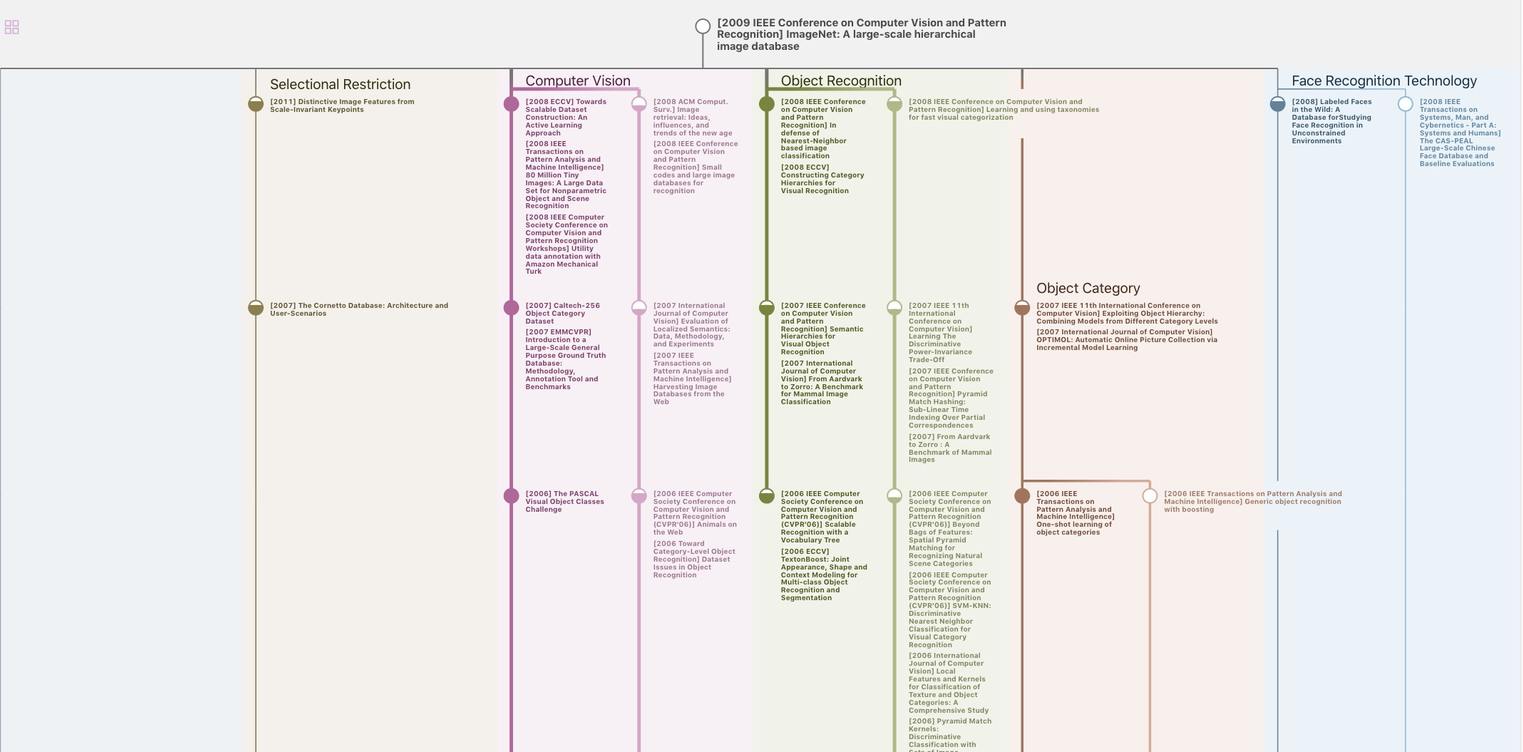
生成溯源树,研究论文发展脉络
Chat Paper
正在生成论文摘要