Shape-aware contrastive deep supervision for esophageal tumor segmentation from CT scans.
2023 IEEE International Conference on Bioinformatics and Biomedicine (BIBM)(2023)
摘要
Accurate tumor segmentation is crucial for esophageal cancer radiotherapy treatment planning. The low contrast among the esophagus, tumors, and surrounding tissues, and irregular tumor shapes limit the performance of automatic segmentation methods. In this paper, we aim to exploit the irregular shapes of tumors to facilitate accurate segmentation. We propose a simple and pluggable shape-aware contrastive deep supervision network (SCDSNet) with shape-aware regularization and voxel-to-voxel contrastive deep supervision. Specifically, the shape-aware regularization with an uncertainty minimization strategy encourages the precise predictions of an additional shape-aware head. The voxel-to-voxel contrastive deep supervision enhances the multi-scale shape-tumor contrast for better voxel-to-voxel prediction of shapes. The proposed method is simple and highly pluggable, which can easily be extended to other frameworks. Further, we establish a large in-house dataset on esophageal cancer to validate the effectiveness of our proposed method. The quantitative and qualitative experimental results demonstrate the effectiveness of SCDSNet on the esophageal cancer dataset.
更多查看译文
关键词
Esophagus tumor segmentation,Voxel-to-voxel contrastive learning,Deep supervision,Shape-aware regularization
AI 理解论文
溯源树
样例
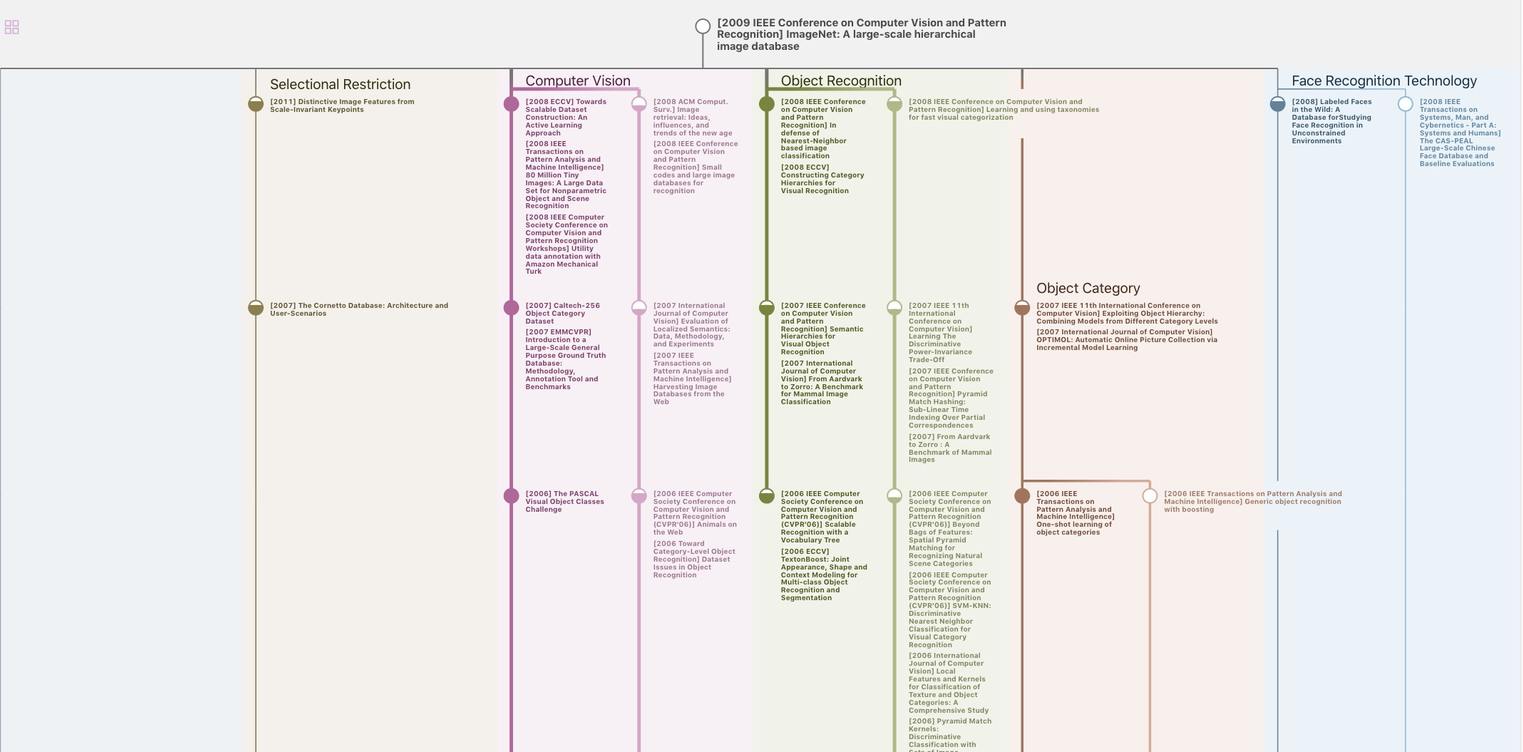
生成溯源树,研究论文发展脉络
Chat Paper
正在生成论文摘要