Emergency Department Wait Time Forecast based on Semantic and Time Series Patterns in COVID-19 Pandemic.
2023 IEEE International Conference on Bioinformatics and Biomedicine (BIBM)(2023)
摘要
This study introduces a new ensemble architecture to improve the wait time forecast for healthcare service in the emergency department (ED) of hospital. The new model first used a fine-tuned text embedding model to extract the contextual semantic meaning of patients’ chief complaint from the electronic patient records to estimate the degree of case urgency and combined to a recurrent neural network to process the regular ED wait time patterns. Four text embedding models including the universal sentence encoder with DAN and transformer encoders, the NNLM, and the Swivel were used for semantic analysis. The results show that the new ensemble model can reduce the prediction errors maximumly by 20.0% in mean of absolute error (MAE), 46.0% in mean of squared error (MSE), and 26.6% in root mean squared error (RMSE). A 5-fold cross validation verified that the new model is robust to the ED wait time prediction before and during the COVID-19 pandemic. We conclude that the new model provides an innovative approach to apply semantic analysis of natural language processing to the domain of time series prediction in the healthcare domain.
更多查看译文
关键词
time series prediction,emergency care,text embedding,transformer,COVID-19
AI 理解论文
溯源树
样例
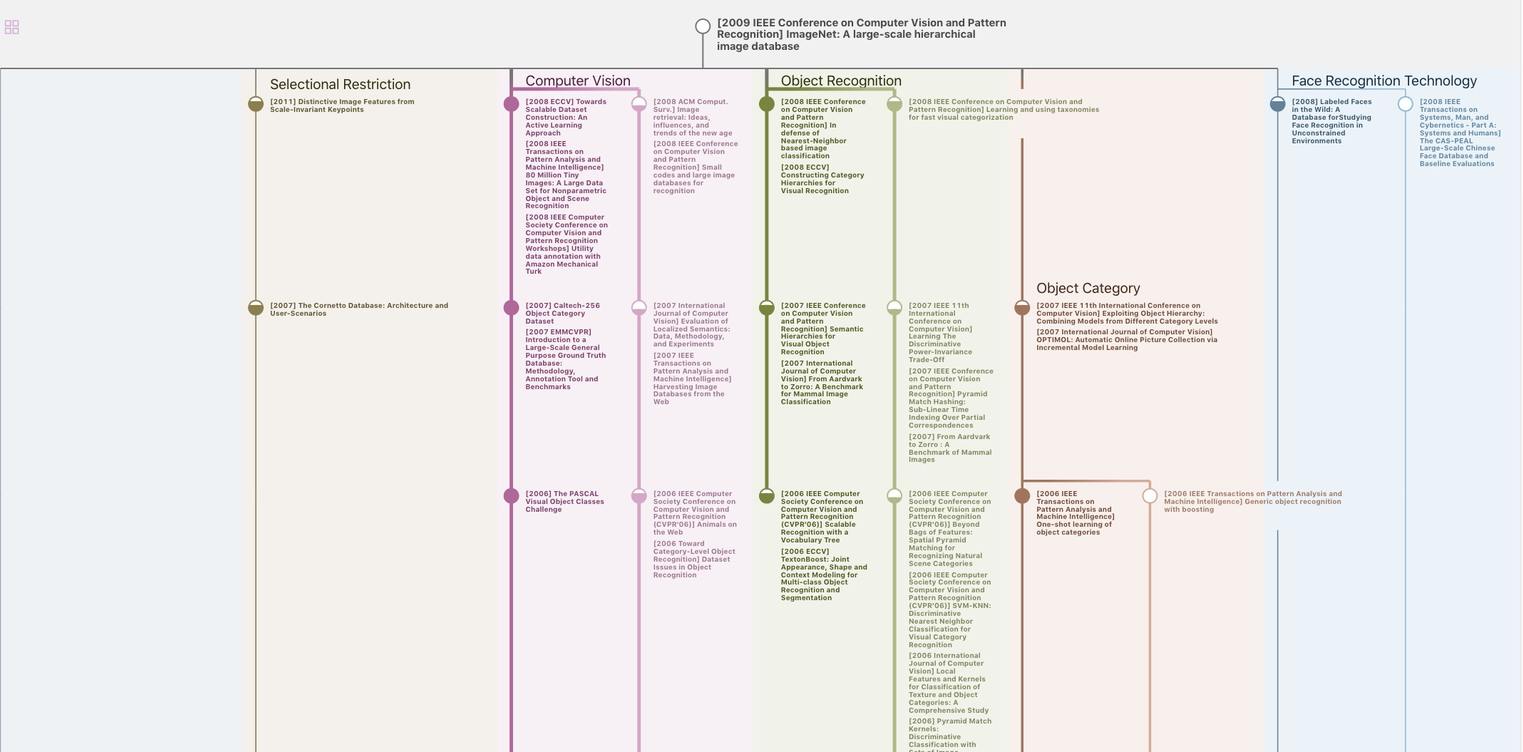
生成溯源树,研究论文发展脉络
Chat Paper
正在生成论文摘要