Transformers for Detection of Distressed Cardiac Patients with an ICD Based on Danish Text Messages.
2023 IEEE International Conference on Bioinformatics and Biomedicine (BIBM)(2023)
摘要
Cardiac patients with implantable cardioverter defibrillator devices frequently exhibit signs of anxiety and depression (termed "Distressed"). Early detection of these patients is vital for evaluation, intervention, and prevention against relapse. Considering the growing datasets relevant to distress, coupled with the evolution of machine learning methodologies, there exists a promising prospect to develop intelligent systems for the detection of distressed cardiac patients through written materials. In this context, data from two sources were collected: a questionnaire and text communication messages, acquired through the randomized ACQUIRE-ICD study of 168 participants. These textual messages were labelled as either Distressed or Non-Distressed based on questionnaire responses. Following preprocessing, the dataset facilitated the development of transformer-based classification models, including mBERT, XLM-RoBERTa, ÆLÆCTRA, and RøBERTa, as well as a hard voting ensemble method to classify patients into Distressed and Non-Distressed categories. To address imbalances in class distribution and dataset scarcity, a data augmentation method was employed. Results indicated the superior performance of the proposed hard voting ensemble, recording weighted metrics of 80% precision, 67% recall, 73% F1-score, and 75% accuracy. Notably, this ensemble correctly identified 67% of Distressed samples, while the most efficient base transformer, mBERT, identified 63% of Distressed samples.
更多查看译文
关键词
Machine Learning,Transformers,Ensemble,Mental Heath,Distress
AI 理解论文
溯源树
样例
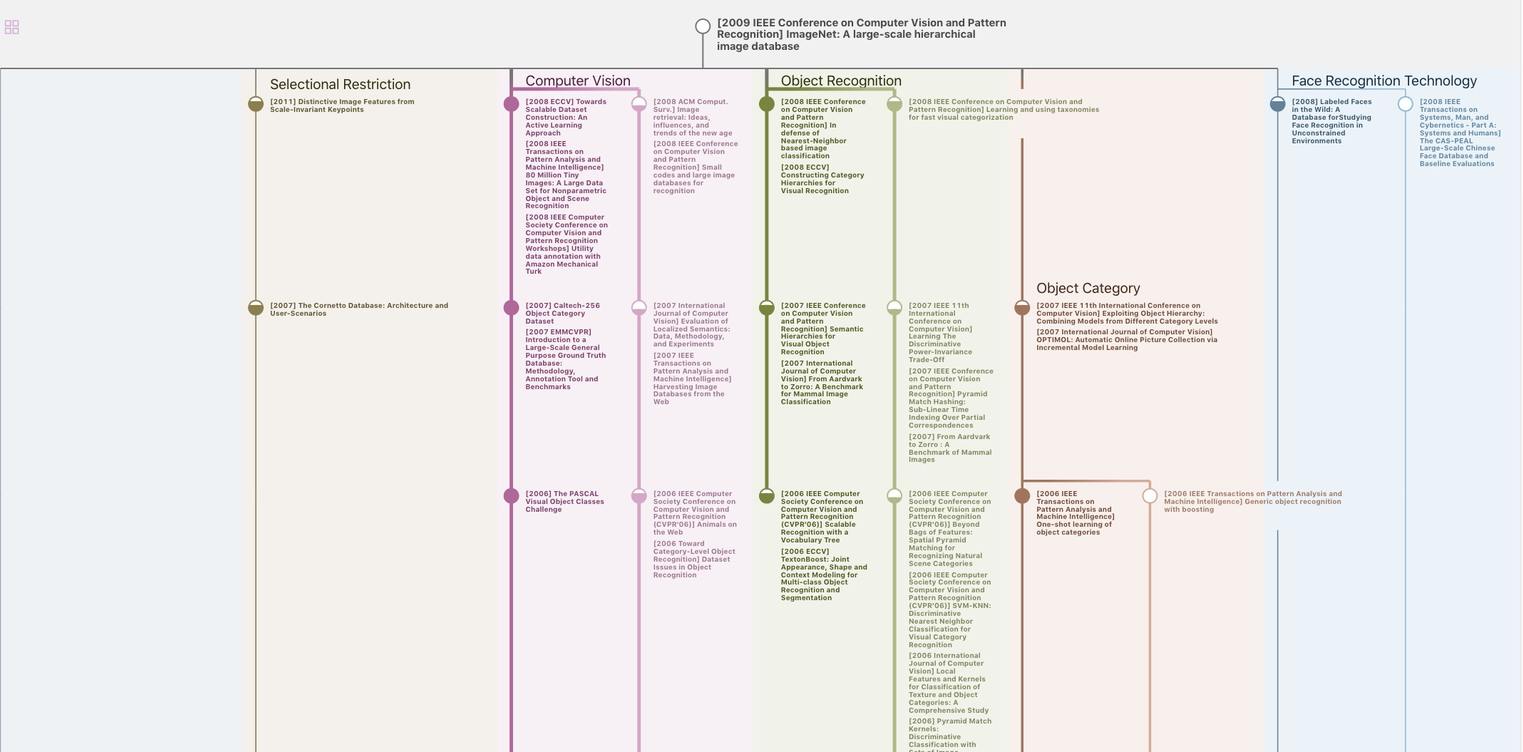
生成溯源树,研究论文发展脉络
Chat Paper
正在生成论文摘要