Retinal vessel segmentation based on multi-scale feature and style transfer
MATHEMATICAL BIOSCIENCES AND ENGINEERING(2024)
摘要
Retinal vessel segmentation is very important for diagnosing and treating certain eye diseases. Recently, many deep learning-based retinal vessel segmentation methods have been proposed; however, there are still many shortcomings (e.g., they cannot obtain satisfactory results when dealing with cross-domain data or segmenting small blood vessels). To alleviate these problems and avoid overly complex models, we propose a novel network based on a multi-scale feature and style transfer (MSFST-NET) for retinal vessel segmentation. Specifically, we first construct a lightweight segmentation module named MSF-Net, which introduces the selective kernel (SK) module to increase the multi-scale feature extraction ability of the model to achieve improved small blood vessel segmentation. Then, to alleviate the problem of model performance degradation when segmenting cross-domain datasets, we propose a style transfer module and a pseudo-label learning strategy. The style transfer module is used to reduce the style difference between the source domain image and the target domain image to improve the segmentation performance for the target domain image. The pseudo-label learning strategy is designed to be combined with the style transfer module to further boost the generalization ability of the model. Moreover, we trained and tested our proposed MSFSTNET in experiments on the DRIVE and CHASE_DB1 datasets. The experimental results demonstrate that MSFST-NET can effectively improve the generalization ability of the model on cross-domain datasets and achieve improved retinal vessel segmentation results than other state-of-the-art methods.
更多查看译文
关键词
retinal vessel segmentation,multi-scale feature,style transfer,pseudo-label learning,deep learning
AI 理解论文
溯源树
样例
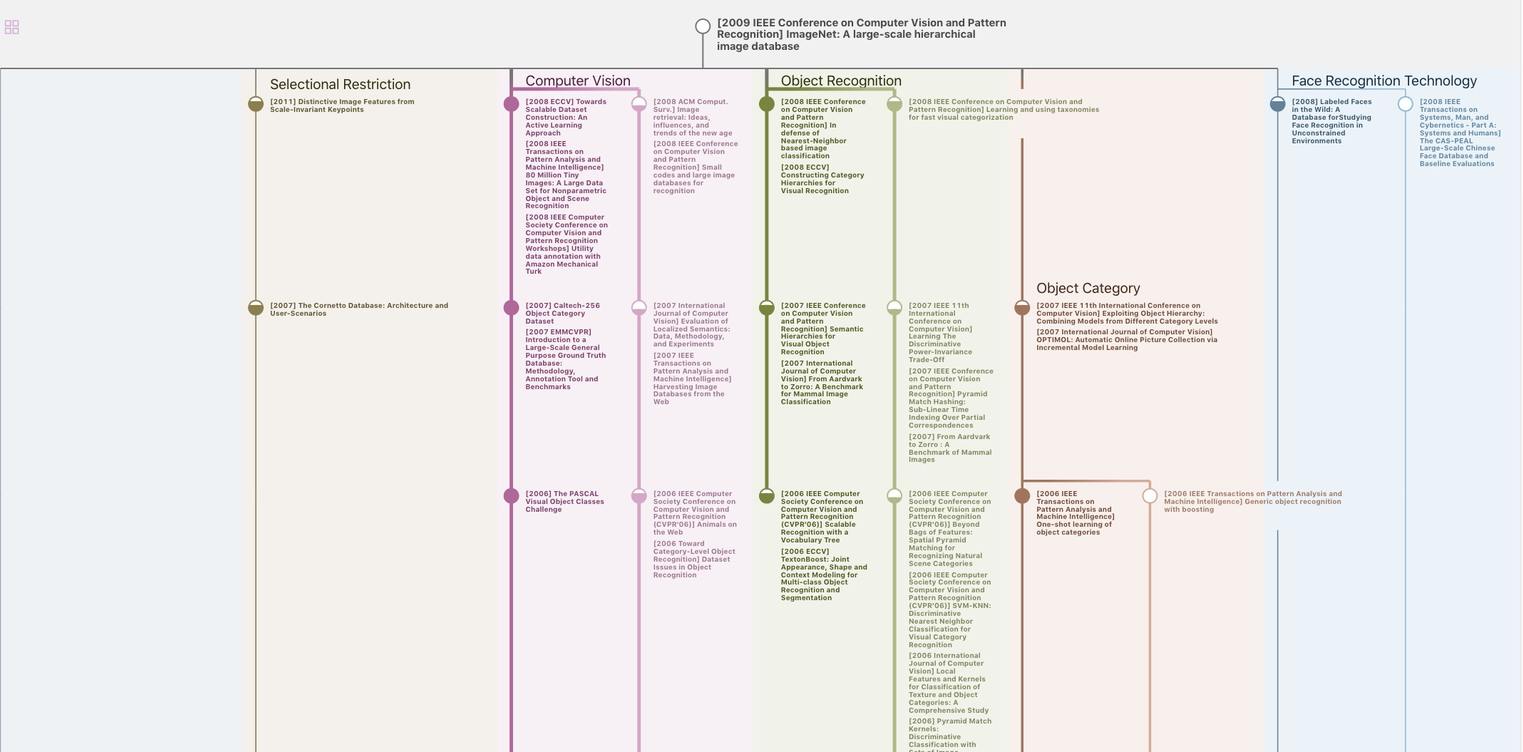
生成溯源树,研究论文发展脉络
Chat Paper
正在生成论文摘要