An adaptive identification method for outliers in dam deformation monitoring data based on Bayesian model selection and least trimmed squares estimation
Journal of Civil Structural Health Monitoring(2024)
摘要
An important technique for the quantitative analysis of dam deformation state is to establish safety monitoring models using deformation monitoring data. To address the shortcomings of conventional monitoring models, such as difficulty in selecting influencing factors and poor ability to resist the interference of outliers, this paper develops a structural safety monitoring model that can realize adaptive identification of various types of outliers in dam deformation monitoring data. The Bayesian model selection (BMS) method is first introduced to select the explanatory variables with a significant impact on the modeling process. On this basis, robust regression analysis of dam deformation monitoring data is performed by using the least trimmed squares (LTS) estimation. In particular, the recovery of clean data and the regression learning are conducted jointly. Furthermore, the double wedge plot is proposed, a graphical display which indicates outliers and potential level shifts. The engineering example demonstrates that, compared with the widely used multiple linear regression (MLR) model based on least squares (LS) fitting, the robust regression model based on BMS-LTS can not only effectively determine the key influencing factors but also adaptively identify various types of outliers in the regression. This study improves the significance of regression and increases the accuracy of prediction; thus, it has good applicability in anomaly detection of dam monitoring data and quantitative analysis of dam safety behavior.
更多查看译文
关键词
Dam safety monitoring,Outlier detection,Robust regression analysis,Least trimmed squares estimation,Bayesian model selection
AI 理解论文
溯源树
样例
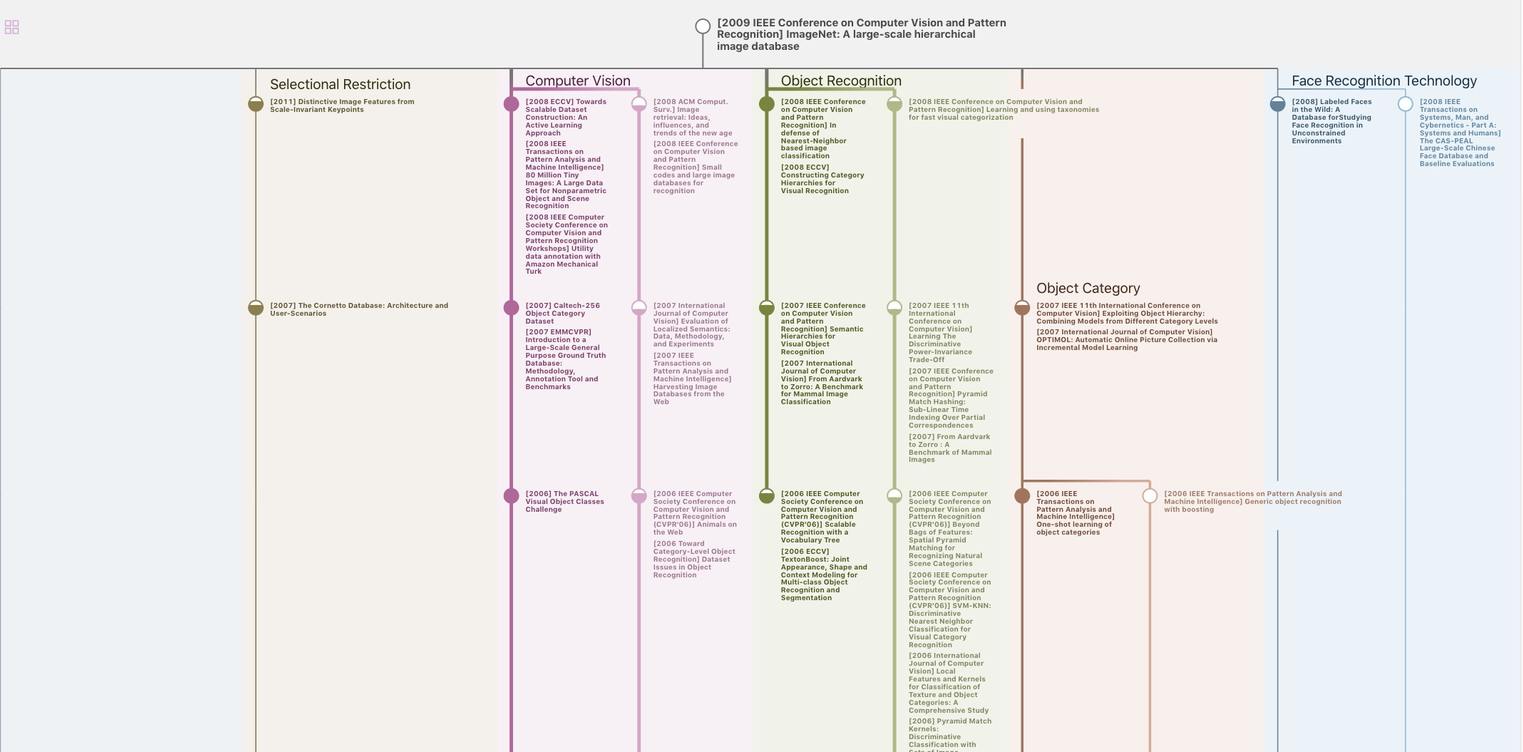
生成溯源树,研究论文发展脉络
Chat Paper
正在生成论文摘要