A Deep Learning Method for the Security Vulnerability Study of Feed-Forward Physical Unclonable Functions
Arabian Journal for Science and Engineering(2024)
摘要
Authentication is critical for Internet-of-Things. The traditional approach of using cryptographic keys is subject to invasive attacks. Being unclonable even by the manufacturers, physical unclonable functions (PUFs) leverage integrated circuits’ manufacturing variations to produce responses unique for individual devices, and hence are of great potential as security primitives. While physically unclonable, many PUFs were reported to be mathematically cloneable by machine learning-based modeling methods. The feed-forward arbiter PUFs (FF PUFs) are among the PUFs with strong resistance against machine learning attacks. Existing studies revealed that only a very small group of FF PUFs with special loop patterns had been broken, and the vast majority of FF PUFs are still secure against all machine learning attack methods tried so far. In this paper, we introduce a neural network that can successfully attack FF PUFs with any loop patterns, with training time even magnitudes lower than existing methods attacking PUFs of the restrictive loop patterns. Experimental results show that, on the one hand, FF PUFs are not secure against attacks even with a large number of complex feed-forward loops, hence susceptible to attacks by response-prediction-based malicious software. On the other hand, the new approach of designing problem-tailored attack methods points to a new way to identify PUF security risks which might be difficult to discover by general-purpose machine learning methods.
更多查看译文
关键词
IoT security,Arbiter PUF,Neural networks,Feed-forward Arbiter PUF,Deep learning
AI 理解论文
溯源树
样例
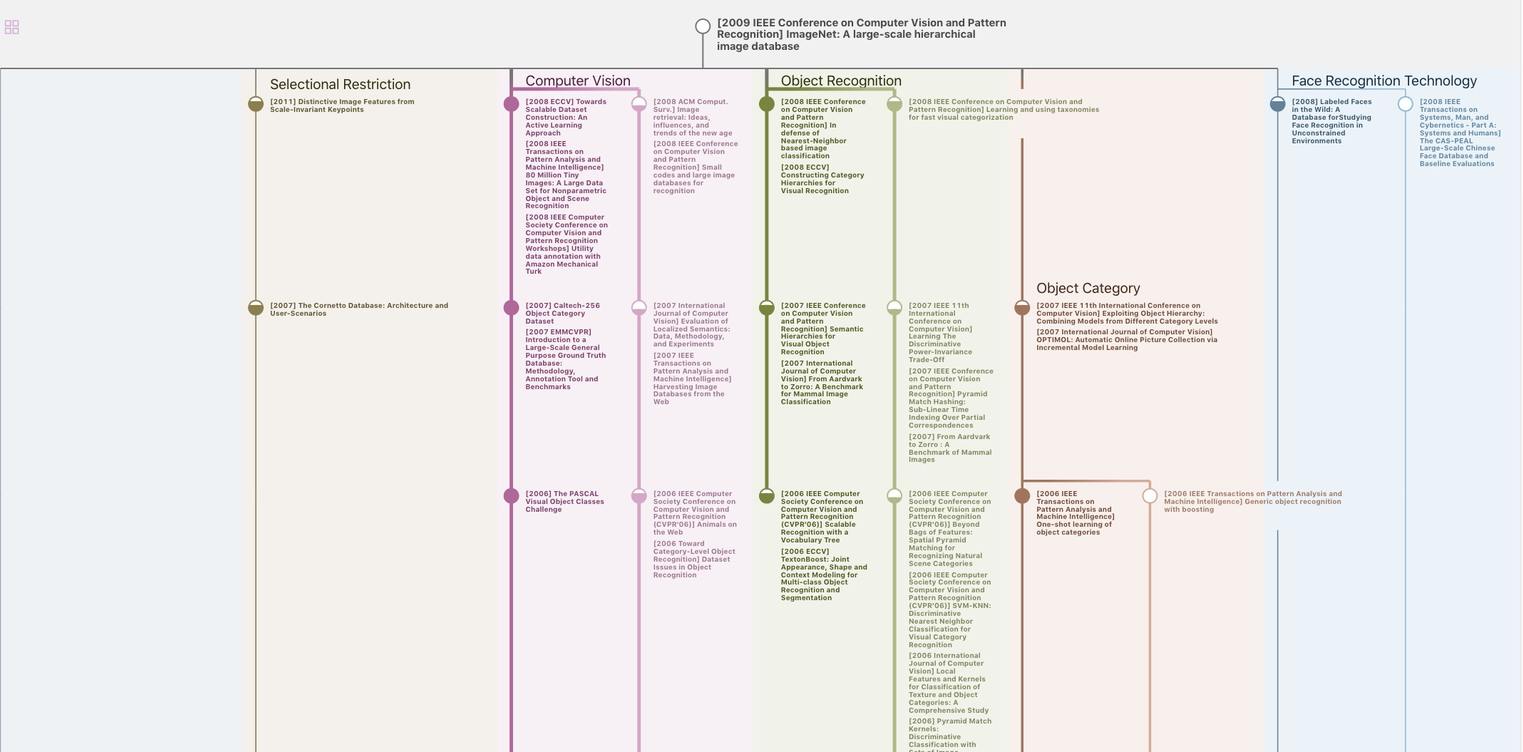
生成溯源树,研究论文发展脉络
Chat Paper
正在生成论文摘要