Learning to Visually Connect Actions and their Effects
CoRR(2024)
摘要
In this work, we introduce the novel concept of visually Connecting Actions and Their Effects (CATE) in video understanding. CATE can have applications in areas like task planning and learning from demonstration. We propose different CATE-based task formulations, such as action selection and action specification, where video understanding models connect actions and effects at semantic and fine-grained levels. We observe that different formulations produce representations capturing intuitive action properties. We also design various baseline models for action selection and action specification. Despite the intuitive nature of the task, we observe that models struggle, and humans outperform them by a large margin. The study aims to establish a foundation for future efforts, showcasing the flexibility and versatility of connecting actions and effects in video understanding, with the hope of inspiring advanced formulations and models.
更多查看译文
AI 理解论文
溯源树
样例
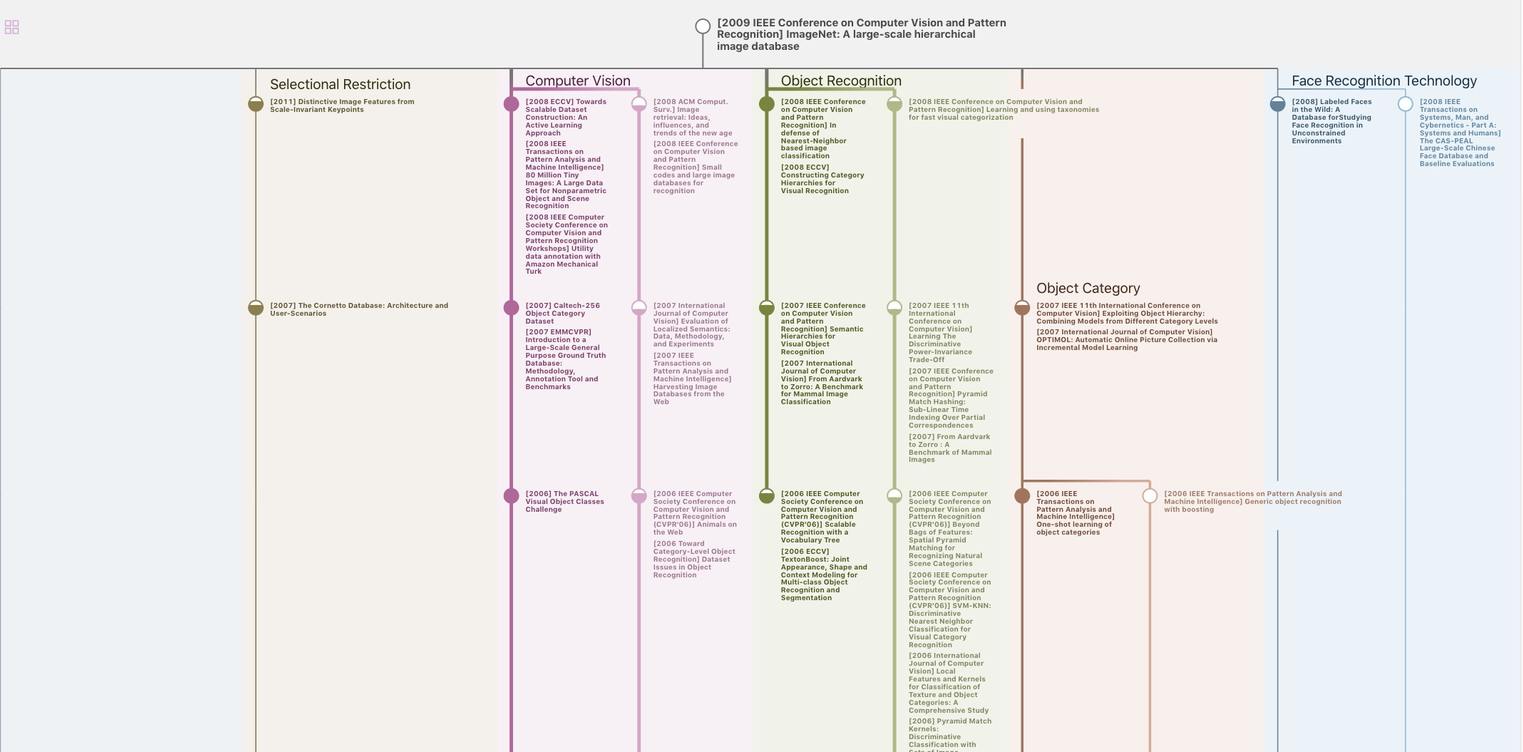
生成溯源树,研究论文发展脉络
Chat Paper
正在生成论文摘要