Towards Automated Driving Violation Cause Analysis in Scenario-Based Testing for Autonomous Driving Systems
CoRR(2024)
摘要
The rapid advancement of Autonomous Vehicles (AVs), exemplified by companies like Waymo and Cruise offering 24/7 paid taxi services, highlights the paramount importance of ensuring AVs' compliance with various policies, such as safety regulations, traffic rules, and mission directives. Despite significant progress in the development of Autonomous Driving System (ADS) testing tools, there has been a notable absence of research on attributing the causes of driving violations. Counterfactual causality analysis has emerged as a promising approach for identifying the root cause of program failures. While it has demonstrated effectiveness in pinpointing error-inducing inputs, its direct application to the AV context to determine which computation result, generated by which component, serves as the root cause poses a considerable challenge. A key obstacle lies in our inability to straightforwardly eliminate the influence of a specific internal message to establish the causal relationship between the output of each component and a system-level driving violation. In this work, we propose a novel driving violation cause analysis (DVCA) tool. We design idealized component substitutes to enable counterfactual analysis of ADS components by leveraging the unique opportunity provided by the simulation. We evaluate our tool on a benchmark with real bugs and injected faults. The results show that our tool can achieve perfect component-level attribution accuracy (100%) and almost (>98%) perfect message-level accuracy. Our tool can reduce the debugging scope from hundreds of complicated interdependent messages to one single computation result generated by one component.
更多查看译文
AI 理解论文
溯源树
样例
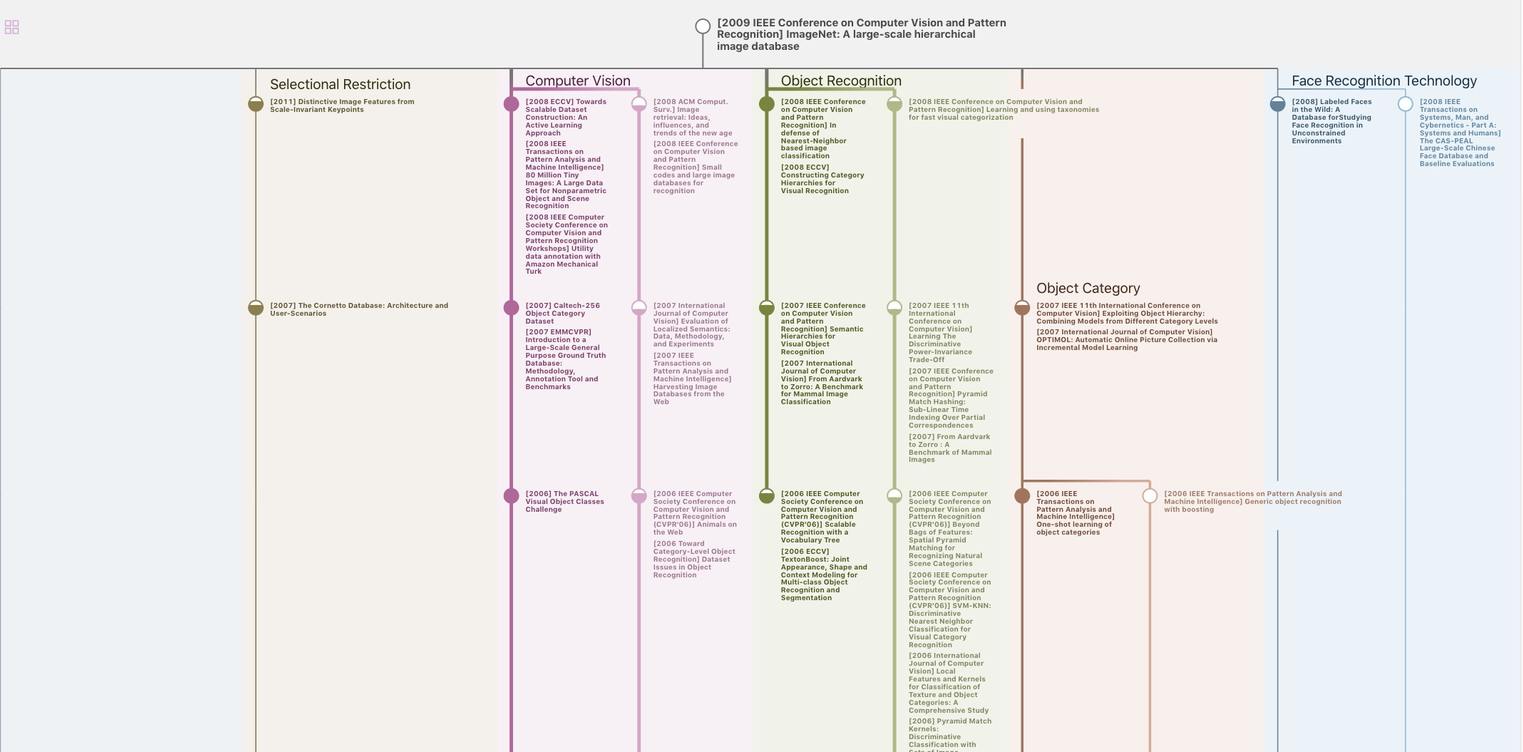
生成溯源树,研究论文发展脉络
Chat Paper
正在生成论文摘要