Eye Movement Characteristics for Predicting a Transition to Psychosis: Longitudinal Changes and Implications
SCHIZOPHRENIA BULLETIN(2024)
摘要
Background and hypothesis Substantive inquiry into the predictive power of eye movement (EM) features for clinical high-risk (CHR) conversion and their longitudinal trajectories is currently sparse. This study aimed to investigate the efficiency of machine learning predictive models relying on EM indices and examine the longitudinal alterations of these indices across the temporal continuum.Study design EM assessments (fixation stability, free-viewing, and smooth pursuit tasks) were performed on 140 CHR and 98 healthy control participants at baseline, followed by a 1-year longitudinal observational study. We adopted Cox regression analysis and constructed random forest prediction models. We also employed linear mixed-effects models (LMMs) to analyze longitudinal changes of indices while stratifying by group and time.Study results Of the 123 CHR participants who underwent a 1-year clinical follow-up, 25 progressed to full-blown psychosis, while 98 remained non-converters. Compared with the non-converters, the converters exhibited prolonged fixation durations, decreased saccade amplitudes during the free-viewing task; larger saccades, and reduced velocity gain during the smooth pursuit task. Furthermore, based on 4 baseline EM measures, a random forest model classified converters and non-converters with an accuracy of 0.776 (95% CI: 0.633, 0.882). Finally, LMMs demonstrated no significant longitudinal alterations in the aforementioned indices among converters after 1 year.Conclusions Aberrant EMs may precede psychosis onset and remain stable after 1 year, and applying eye-tracking technology combined with a modeling approach could potentially aid in predicting CHRs evolution into overt psychosis.
更多查看译文
关键词
eye movement,longitudinal modeling,early psychosis,clinical high risk for psychosis,transition
AI 理解论文
溯源树
样例
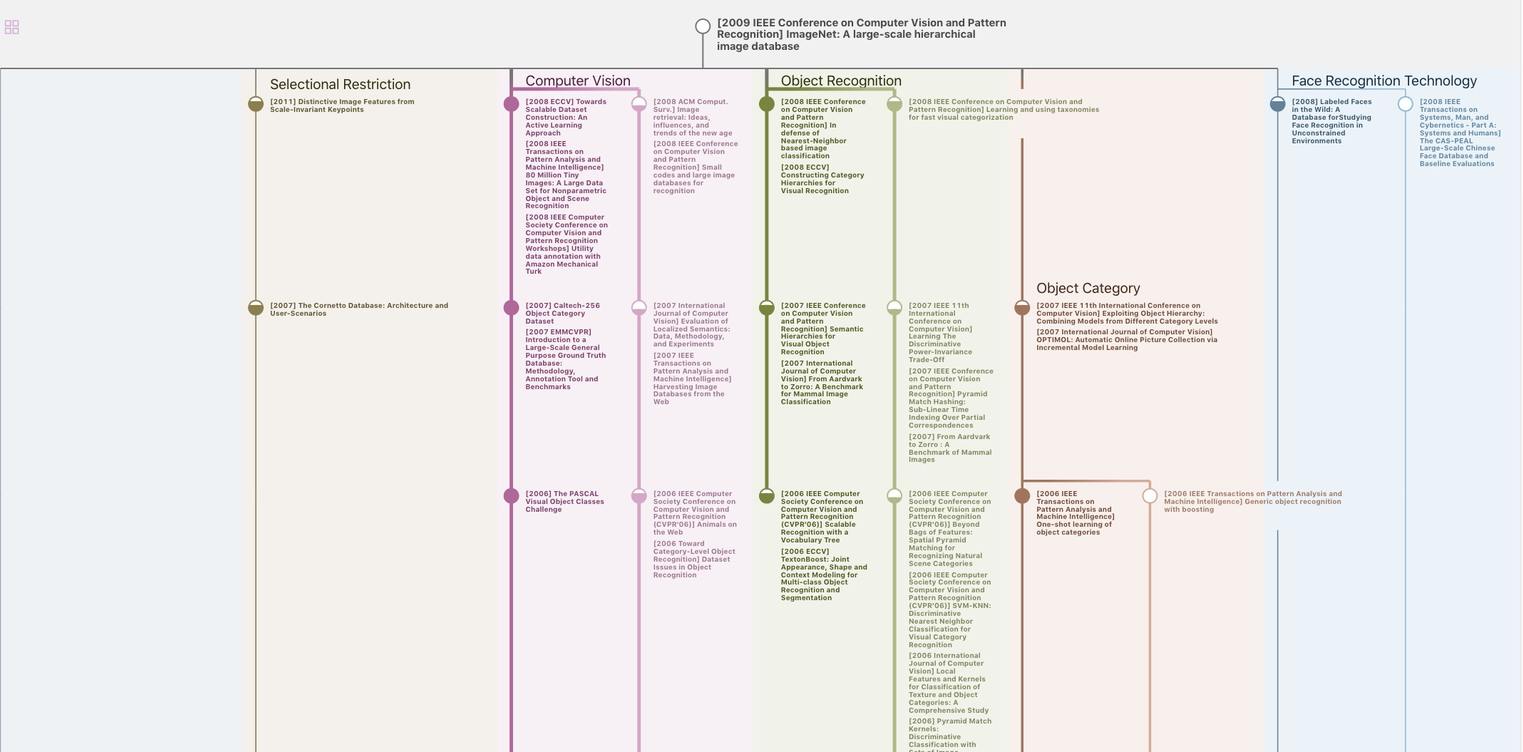
生成溯源树,研究论文发展脉络
Chat Paper
正在生成论文摘要