Pathways-driven Sparse Regression Identifies Pathways and Genes Associated with High-density Lipoprotein Cholesterol in Two Asian Cohorts
arXiv (Cornell University)(2013)
摘要
Standard approaches to analysing data in genome-wide association studies (GWAS) ignore any potential functional relationships between genetic markers. In contrast gene pathways analysis uses prior information on functional structure within the genome to identify pathways associated with a trait of interest. In a second step, important single nucleotide polymorphisms (SNPs) or genes may be identified within associated pathways. Most pathways methods begin by testing SNPs one at a time, and so fail to capitalise on the potential advantages inherent in a multi-SNP, joint modelling approach. Here we describe a dual-level, sparse regression model for the simultaneous identification of pathways, genes and SNPs associated with a quantitative trait. Our method takes account of various factors specific to the joint modelling of pathways with genome-wide data, including widespread correlation between genetic predictors, and the fact that variants may overlap multiple pathways. We use a resampling strategy that exploits finite sample variability to provide robust rankings for pathways, SNPs and genes. We test our method through simulation, and use it to perform pathways-driven SNP selection in a search for pathways, genes and SNPs associated with variation in serum high-density lipoprotein cholesterol (HDLC) levels in two separate GWAS cohorts of Asian adults. By comparing results from both cohorts we identify a number of candidate pathways including those associated with cardiomyopathy, and T cell receptor and PPAR signalling. Highlighted genes include those associated with the L-type calcium channel, adenylate cyclase, integrin, laminin, MAPK signalling and immune function.
更多查看译文
关键词
sparse regression identifies pathways-driven,asian cohorts,genes,high-density
AI 理解论文
溯源树
样例
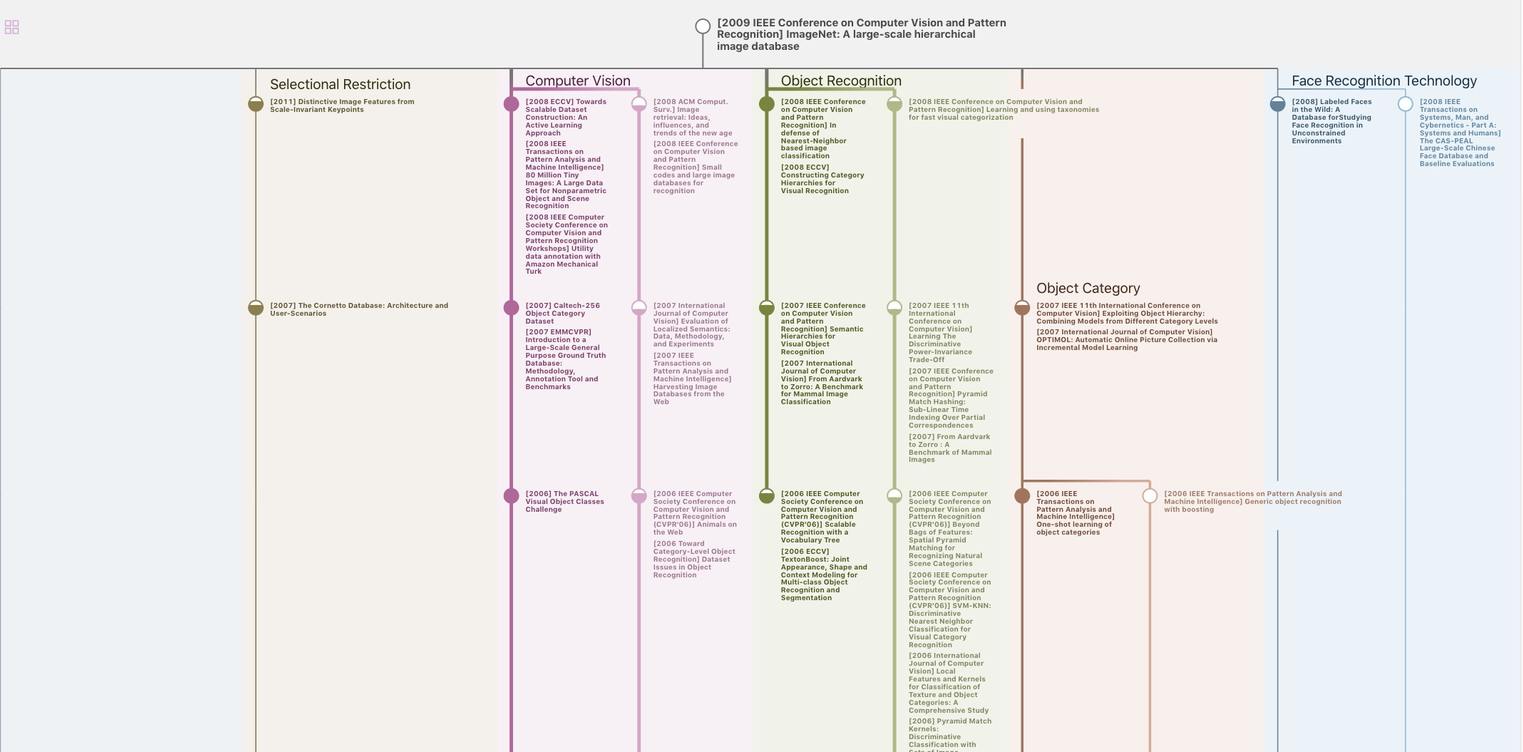
生成溯源树,研究论文发展脉络
Chat Paper
正在生成论文摘要