Non-sample fuzzy based convolutional neural network model for noise artifact in biomedical images
Discover Applied Sciences(2024)
摘要
The use of a light-weight deep learning Convolutional Neural Network (CNN) augmented with the power of Fuzzy Non-Sample Shearlet Transformation (FNSST) has successfully solved the problem of reducing noise and artifacts in Low-Dose Computed Tomography (LDCT) pictures. Both the Normal-Dose Computed Tomography (NDCT) and the Low-Dose Computed Tomography (LDCT) images from the dataset are subjected to the FNSST decomposition procedure during the training phase, producing high-frequency sub-images that act as input for the CNN. The CNN creates a meaningful connection between the high-frequency sub-images from LDCT and their corresponding residual sub-images during the training operation. The CNN is given the capacity to distinguish between LDCT high-frequency sub-images and expected high-frequency sub-images, which frequently have varying levels of noise or artifacts, especially in a fuzzy setting. The FNSST-CNN then successfully distinguishes LDCT high-frequency sub-images from the expected high-frequency sub-images during the testing phase, thereby reducing noise and artifacts. When compared to other approaches like KSVD, BM3D, and conventional image domain CNNs, the performance of FNSST-CNN is impressive as shown by better peak signal-to-noise ratios, stronger structural similarity, and a closer likeness to NDCT pictures.
更多查看译文
关键词
CNN,Biomedical Image,Peak Signal-To-Noise ratio,Structural similarity,Artifact Problem
AI 理解论文
溯源树
样例
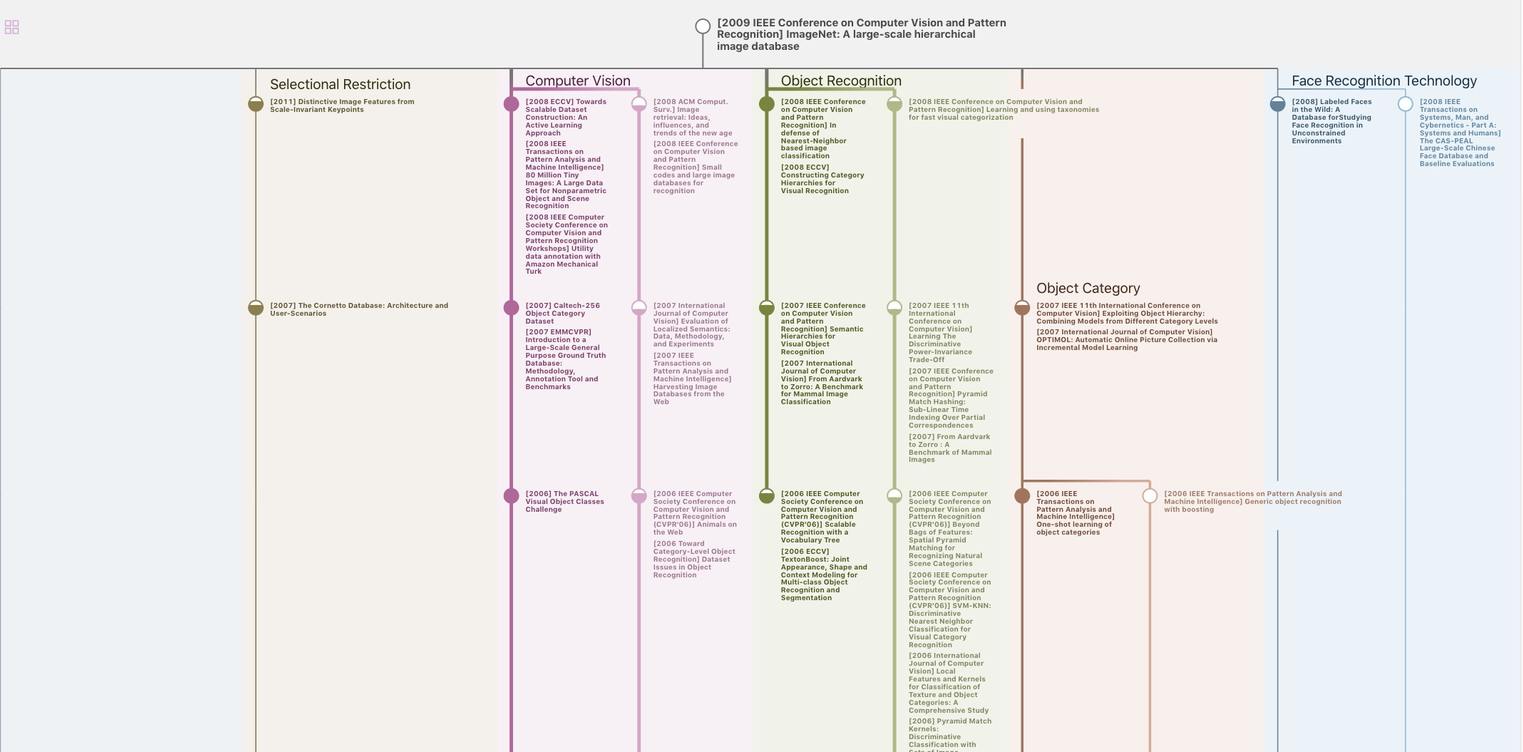
生成溯源树,研究论文发展脉络
Chat Paper
正在生成论文摘要