Virtual Classification: Modulating Domain-Specific Knowledge for Multidomain Crowd Counting
IEEE TRANSACTIONS ON NEURAL NETWORKS AND LEARNING SYSTEMS(2024)
摘要
Multidomain crowd counting aims to learn a general model for multiple diverse datasets. However, deep networks prefer modeling distributions of the dominant domains instead of all domains, which is known as domain bias. In this study, we propose a simple-yet-effective modulating domain-specific knowledge network (MDKNet) to handle the domain bias issue in multidomain crowd counting. MDKNet is achieved by employing the idea of "modulating", enabling deep network balancing and modeling different distributions of diverse datasets with little bias. Specifically, we propose an instance-specific batch normalization (IsBN) module, which serves as a base modulator to refine the information flow to be adaptive to domain distributions. To precisely modulating the domain-specific information, the domain-guided virtual classifier (DVC) is then introduced to learn a domain-separable latent space. This space is employed as an input guidance for the IsBN modulator, such that the mixture distributions of multiple datasets can be well treated. Extensive experiments performed on popular benchmarks, including Shanghai-tech A/B, QNRF, and NWPU validate the superiority of MDKNet in tackling multidomain crowd counting and the effectiveness for multidomain learning.
更多查看译文
关键词
Crowd counting,domain-guided virtual classifier (DVC),instance-specific batch normalization (IsBN),multidomain learning
AI 理解论文
溯源树
样例
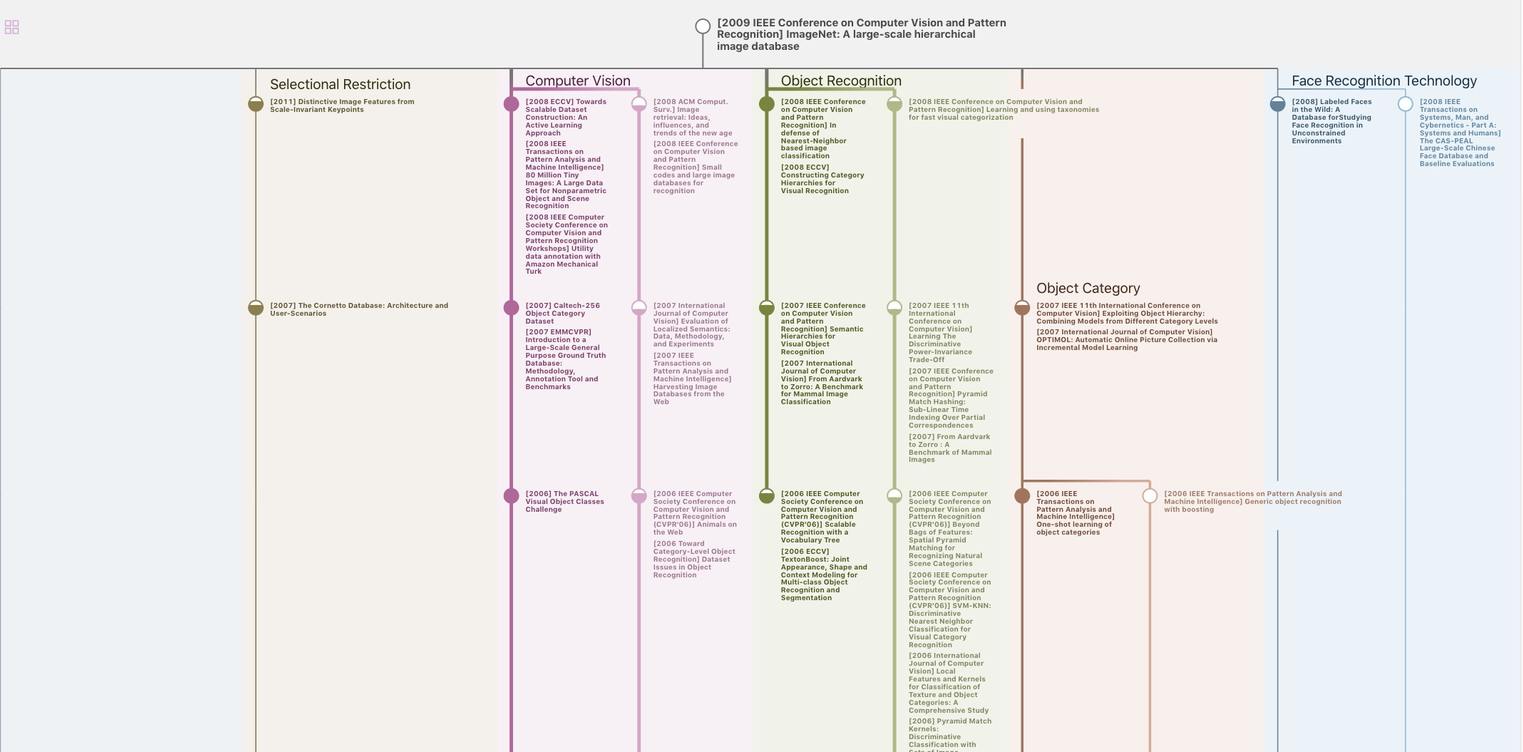
生成溯源树,研究论文发展脉络
Chat Paper
正在生成论文摘要