Multi-Instance Nonparallel Tube Learning
IEEE TRANSACTIONS ON NEURAL NETWORKS AND LEARNING SYSTEMS(2024)
摘要
In multi-instance nonparallel plane learning (NPL),the training set is comprised of bags of instances and the nonparallel planes are trained to classify the bags. Most of the existing multi-instance NPL methods are proposed based on a twin support vector machine (TWSVM). Similar to TWSVM, they use only a single plane to generalize the data occurrence of one class and do not sufficiently consider the boundary information, which may lead to the limitation of their classification accuracy. In this article, we propose a multi-instance nonparallel tube learning(MINTL) method. Distinguished from the existing multi-instance NPL methods, MINTL embeds the boundary information into the classifier by learning a large-margin-based epsilon-tube for each class, such that the boundary information can be incorporated into refining the classifier and further improving the performance. Specifically, given epsilon(K)-class multi-instance dataset, MINTL seeksK epsilon-tubes, one for each class. In multi-instance learning, each positive bag contains at least one positive instance. To buildup the epsilon k-tube of class k, we require that each bag of class k should have at least one instance included in the epsilon(k)-tube.Moreover, except for one instance included in the epsilon k-tube, there maining instances in the positive bag may include positive instances or irrelevant instances, and their labels are unavailable. A large margin constraint is presented to assign the remaining in stances either inside the epsilon k-tube or outside the epsilon k-tube with a large margin. Substantial experiments on real-world dataset shave shown that MINTL obtains significantly better classification accuracy than the existing multi-instance NPL methods.
更多查看译文
关键词
Electron tubes,Training,Support vector machines,Refining,Manifolds,Learning systems,Laplace equations,Multi-instance learning,nonparallel tube classifier
AI 理解论文
溯源树
样例
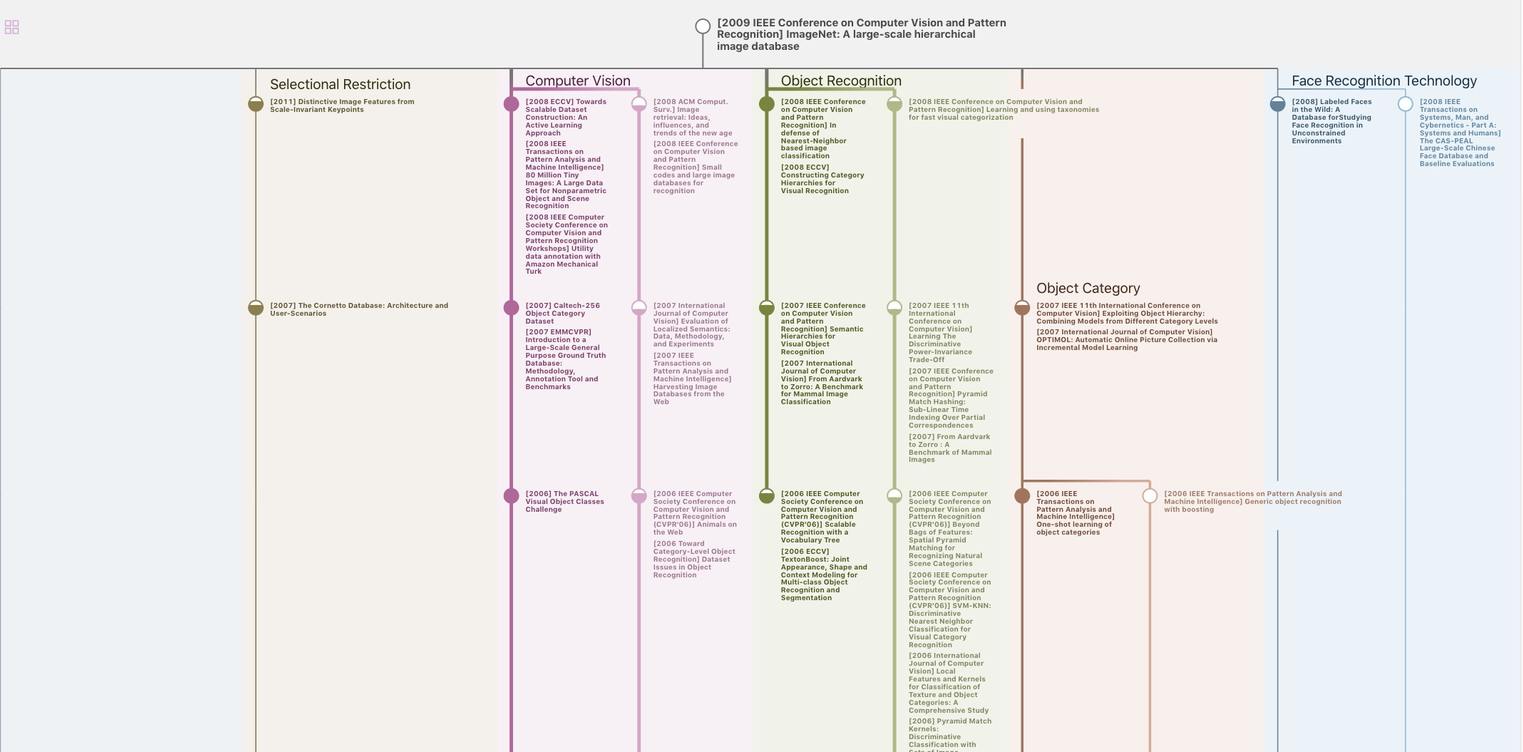
生成溯源树,研究论文发展脉络
Chat Paper
正在生成论文摘要